Advanced analytics is enabling superior performance in organizations willing to make the proper commitment: across all industries, companies that are more analytically driven realize financial growth three times higher than their less analytical competitors, according to McKinsey’s Analytics Quotient (see sidebar).
Industries ranking highest on analytics maturity include pharmaceuticals and medical products; insurance; and energy, materials, and agriculture. But banking, with a long history in leveraging data, starts from the strongest position.
However, progress within banking is relative—many firms have yet to realize the full potential from embedding analytics deep into their culture, decision processes, and business operations. We recently conducted an in-depth analysis of more than 20 banks in Europe, Middle East, and Africa (EMEA), assessing their analytics maturity across six dimensions. While the banks in this group have generally constructed strong initial analytics foundations, there is still room for them to improve performance. The study revealed five areas where banks can improve returns:
- Align analytics priorities to strategic vision. For more than half of the banks surveyed analytics is a strategic theme, but the majority struggle to connect the high-level analytics strategy to a targeted selection and prioritization of use cases, and to implement them in an orchestrated way. Banks are starting to leverage advanced-analytics techniques in several areas—commercial, risk, innovation, and technology—but for many, top-down views limit the potential of analytics in their core strategic activities.
- Embed analytics into decision making and workflows. Senior managers acknowledge the potential value of analytics, but they often do not know how to expand from a few pilots to a full-scale impact. Some firms are challenged by shortages of technical production and engineering capabilities. For others, the problem lies in the absence of a change-management program to drive adoption or a culture that fails to support data-driven decision making and rapid, agile iterations.
- Develop advanced-analytics assets and teams to scale. Most banks have successfully launched single, stand-alone initiatives in advanced analytics, but few have developed them into efficient, large-scale operations. More successful organizations gain traction through advanced-analytics centers of excellence (COEs) and expand their analytics scale to accelerate impact. Broader analytics reveal transformative opportunities and enable interfaces with third-party vendors, allowing development of external capabilities, know-how, and assets.
- Invest in critical analytics roles. Banks are expanding their analytics teams to meet the growing need for specific technical profiles: data engineers, data scientists, visualization specialists, and machine-learning engineers. But, in addition to adding pure technical analytics talent, banks are facing challenges in building “translator” capabilities and fostering effective collaboration among different roles. Translators are a crucial link between business and analytics and typically come from within business units. They help data scientists understand business problems and priorities and ensure that analytics insights are communicated back to business units, and therefore they must be fluent in the languages of both.
- Enable the user revolution. Banks possess great sources of data suitable for many use cases, but data practices tend to be narrow in scope and focused on regulation. Moreover, data often is not made readily available to the broader organization. Companies typically impose formal constraints on data security, privacy, and compliance, but few define clear data ownership and maintain high-quality data that is ready for application to develop analytics use cases.
Banks display a range of approaches to analytics and varying success in embedding analytics into their “cultural DNA.” This article details our findings among the sample of EMEA banks and provides more detail on the five imperatives that will enable banks to reach their full analytics potential.
Align analytics priorities to strategic vision
Advanced analytics in banking has evolved considerably in the last few years. Most banks can articulate an analytics strategy and have implemented—or are in the process of implementing—a set of use cases. However, in many cases there is a disconnect among the use cases defined by business units, the broader goals of the organization, and the aspiration to use advanced analytics to help realize these goals in the next three years.
Among the banks we surveyed, only 30 percent have effectively matched their analytics efforts with their business goals. However, among the subset of firms that scored high on analytics maturity overall, 60 percent have aligned their analytics use cases with their strategic priorities.
Next steps
Banks currently concentrate most of their analytics use cases in sales management (for example, next product to buy, digital marketing, and transactional analytics), financial risk management (collections), and nonfinancial risks (cybersecurity and fraud detection). These are logical first choices, but banks also need an analytics road map for the entire organization to ensure transparency and clarity on their aspiration for advanced analytics.
Before launching efforts on specific use cases, banks should identify those areas where analytics will do the most to enhance their value propositions, in line with their business strategies. Over time, banks should extend analytics to other functions and set their ambitions for how analytics will help the organization in the years ahead.
Across industries, analytics leaders integrate analytics not only into a few crucial business units but also across all operations. This is true for analytics leaders among banks as well: more than half have introduced use cases to three or more functional areas.
Would you like to learn more about our Financial Services Practice?
Embed analytics into decision making and workflows
Firms also face a significant challenge in turning their analytics insights into business outcomes and realizing the full value of analytics—what we term “attention to the last mile.” In addition to significant technical and production engineering challenges, getting the most from advanced analytics requires business adoption and change management. By their own reckoning, only 7 percent of surveyed banks had achieved full integration of key analytics use cases.
Moreover, many banking leaders have not yet adopted a data-driven mind-set for decision making: just 15 percent of respondents believe that their bank leadership makes decisions from a heavy reliance on analytics, and only 20 percent of firms believe that their leaders will be persuaded by analytics insights that run counter to their initial beliefs.
While many banks surveyed are convinced of the potential impact of analytics, in many instances this message is not clearly transmitted from senior management to the front line. Without this emphasis, business teams will fail to understand the power of analytics, and in turn, relationship managers, marketing teams, and credit underwriters will not be motivated to make the necessary changes in mind-set. As a result, while half of management teams at EMEA banks surveyed claim to be sold on the value of analytics, only 25 percent effectively communicate a change story on how analytics will improve operations, and only 20 percent of banks succeed in expanding investments in analytics to scale.
Even data-savvy banks have a hard time gaining high-visibility, firm-wide engagement from middle management, and building front-line and management capabilities to scale advanced analytics efforts across the organization.
Next steps
At almost two-thirds of banks applying analytics, C-suite sponsors evangelize their programs and give progress reports on strategies to the broader organization. These communications should emphasize how analytics can be a complement—or counterpoint—to established practices.
Once strategically relevant use cases are defined, management should identify end-to-end business owners who can move them from pilot stage to full scale and create accountability and incentives to capture maximum impact.
Early on in analytics planning, banks should consider how the insights will be delivered and contribute to decision making. Data-visualization specialists, designers of user experiences and interfaces, and behavioral economists all can play a role and reshape the bank’s workflow design, digital tooling, and decision processes.
Banks also should invest time and effort into completing the last mile. Analytically mature firms typically allocate more than half of their investments to embedding decision making in line organizations—process and workflow definition, team capabilities, and an effective rollout. Banks can address the key challenges to adopting analytics by providing front-line staff with actionable real-time insights, establishing intuitive key performance indicators, and ensuring that business owners move from idea to implementation.
Operationally, managers should leave analytics complexity behind the curtain. For example, whether banks follow rule-based logic for mapping product offers to basic customer segments or employ more sophisticated machine-learning methods for targeting product offers to customer microsegments, front-line representatives and call-center agents need not be aware of the underlying technology when prompting best next actions.
Develop advanced-analytics assets and teams to scale
Banks follow disparate approaches to positioning their analytics teams. Forty percent of banks follow a hybrid approach that concentrates analytics talent in COEs, providing solutions to the entire bank and balancing analytics efforts within business units. About one-fourth take a completely decentralized approach, while the remainder implement highly centralized solutions.
Decentralized approaches are effective when there is a need to stay close to the business units to infuse domain expertise and drive adoption (and when a lower priority is assigned to scaling quickly and consistently across business units). Centralization of the analytics organization is typically better suited to a company that is starting its analytics journey and seeking to establish groupwide capabilities and consistent policies and language. A hybrid state with a COE defines the direction of the strategy, stays abreast of the latest methodological advancements, provides shared analytics services, and moves the organization towards an agile culture.
Next steps
Banks should focus on building core advanced-analytics capabilities to capture transformative opportunities and to interface with third-party vendors, which will enable banks to leverage existing know-how, solutions, and assets as well as infrastructure. These efforts start with a COE engineered to quickly deliver successful use cases. Establishing such a foundation and leveraging quick wins are prerequisites to building a data-driven organization.
There is a proven approach to designing the core structural elements of an advanced-analytics COE: identifying impact opportunities, establishing reporting lines and ownership, hiring and training, developing the analytics delivery model, and building organization-wide analytics capabilities.
Invest in critical analytics roles
Banks are short on analytics talent. Few managers know the exact number of dedicated specialists—data scientists, engineers, and architects, as well as visualization specialists, workflow integrators, delivery managers, and product owners—within their organizations or can fully define their roles. Little wonder, then, that 80 percent of surveyed banks find it difficult to recruit the right analytics talent.
Organizations require a variety of skills and well-defined roles and modus operandi to bring together business, analytics, and technology. Our findings indicate that stakeholders at only 20 percent of banks believe that analytics has been given an adequate role, and just 14 percent report that their teams of data scientists, architects, and engineers have been assigned clear responsibilities.
Irrespective of their organizational structures, banks have underemphasized the crucial role of translators—the ambassadors who ensure that analytics solves critical business problems. Translators typically guide and collaborate with data engineers and data scientists to translate business problems into a language they can clearly understand, and then ensure actionable insights are integrated into the business units’ workflows and decision making. Translators typically come from within business units, allowing them to build an analytics awareness in the broader organization. They are especially important in organizations and business units that have not previously emphasized analytics literacy.
Next steps
Banks must step up their investments in analytics, expanding their recruiting strategies and competing with leading tech firms for the talents of data scientists, data engineers, and translators. High achievers centralize their analytics talent, providing analytical support across bank business units (for example, marketing, risk, human resources, IT, and operations).
Banks need to create or expand training programs to broaden analytics understanding at all levels—senior management, business-team leaders, and non-analytics employees. Several major European banks have made fluency in analytics a requirement for advancement not only to the C-suite but also for the top echelon of all management. Typically, such efforts to upgrade skill levels span two years or more.
Banks should also expand the number and scope of use cases they undertake, engaging additional stakeholders and adopting a “fail fast, win fast” philosophy. Most banks surveyed are already planning an average 20 percent increase in their analytics investment over the coming three years and an expansion of analytics teams and translator teams by more than three times.
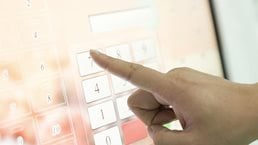
A bank branch for the digital age
Enable the user revolution
Data collection and security have long been core priorities for banks: more than half of those surveyed report having formal systems for data security, privacy, and compliance. However, just one-third possess data resources that are clean and ready for advanced analysis.
Moreover, banks’ analytics strategies and efforts to date have focused primarily on fulfilling regulatory requirements, not on strategic value creation. Few banks have data strategies that support delivery of broad-based analytics efforts, and a similarly small number have provided employees with ready access to information.
However, because they are data conscious, banks have a head start on their analytics solutions. Two-thirds already offer their data analysts and scientists a state-of-the-art workbench with an extensive set of tools. But there is a wide variance in their deployment and sophistication. Most of the banks we studied rely heavily on simple descriptive and predictive models; more sophisticated techniques, such as real-time predictive analytics enabled by machine learning, remain in the early stages.
Next steps
Banks should strengthen their data-management processes to ensure that adequate amounts of relevant data are gathered, available, and actionable. Central data repositories (for example, data lakes) stocked with exhaustive primary information on customer transactions are a foundation, but management should also be open to data that is less rigorously structured, such as logs from call centers. Moreover, valuable insights can be drawn from information that originates outside the organization—from governments, trade organizations, industry utilities, and cross-industry coalitions sharing information. Data should also be “democratized,” giving open but secure access to all those entitled to draw on it.
Analytics leaders should have a range of tools to call upon; more importantly, they need an approach for integrating them across their systems and business operations. Banks leading the way in analytics have a methodology for the development of models, interpretation of data, and deployment of new capabilities—heavily involving the front line—to generate relevant real-time insights for the business. These leaders also continually score the results of a range of models and implement the most effective.
For banks, advanced analytics should be a broad capability rather than a stand-alone function. Success in this regard requires that banks rethink tools, platforms, and methodologies. To support this shift in mind-set, leading banks are creating pull from users with prominent in-house academies. These programs address analytics literacy for all employees and inspire tangible and practical use cases to mobilize the organization.
McKinsey estimates that sharpening analytics efforts could lead to an increase in earnings of as much as $1 trillion annually for the global banking industry. The benefits would be widespread, but about one-third of the gains would come in reduced fraud losses and about 20 percent from better informed pricing and promotion. To realize these benefits, banks need to build on their long history of using data to become true analytics leaders.