It’s no secret that organizations have been increasingly turning to advanced analytics and artificial intelligence (AI) to improve decision making across business processes—from research and design to supply chain and risk management.
Stay current on your favorite topics
Along the way, there’s been plenty of literature and executive hand-wringing over hiring and deploying ever-scarce data scientists to make this happen. Certainly, data scientists are required to build the analytics models—largely machine learning and, increasingly, deep learning—capable of turning vast amounts of data into insights.
More recently, however, companies have widened their aperture, recognizing that success with AI and analytics requires not just data scientists but also entire cross-functional, agile teams that include data engineers, data architects, data-visualization experts, and—perhaps most important—translators.
Why are translators so important? They help ensure that organizations achieve real impact from their analytics initiatives (which has the added benefit of keeping data scientists fulfilled and more likely to stay on, easing executives’ stress over sourcing that talent).
What exactly is an analytics translator?
To understand more about what translators are, it’s important to first understand what they aren’t. Translators are neither data architects nor data engineers. They’re not even necessarily dedicated analytics professionals, and they don’t possess deep technical expertise in programming or modeling.
Instead, translators play a critical role in bridging the technical expertise of data engineers and data scientists with the operational expertise of marketing, supply chain, manufacturing, risk, and other frontline managers. In their role, translators help ensure that the deep insights generated through sophisticated analytics translate into impact at scale in an organization. By 2026, the McKinsey Global Institute estimates that demand for translators in the United States alone may reach two to four million.
What does a translator do?
At the outset of an analytics initiative, translators draw on their domain knowledge to help business leaders identify and prioritize their business problems, based on which will create the highest value when solved. These may be opportunities within a single line of business (e.g., improving product quality in manufacturing) or cross-organizational initiatives (e.g., reducing product delivery time).
Would you like to learn more about our Analytics Practice?
Translators then tap into their working knowledge of AI and analytics to convey these business goals to the data professionals who will create the models and solutions. Finally, translators ensure that the solution produces insights that the business can interpret and execute on, and, ultimately, communicates the benefits of these insights to business users to drive adoption.
Given the diversity of potential use cases, translators may be part of the corporate strategy team, a functional center of excellence, or even a business unit assigned to execute analytics use cases.
What skills do translators need?
The wide range of responsibilities—leader, communicator, project manager, industry expert—inherent in the translator role makes the following skills essential.
Domain knowledge
Domain knowledge is by far the most important skill for any translator. Translators must be experts in both their industry and their company to effectively identify the value of AI and analytics in the business context. They must understand the key operational metrics of the business and their impact on profit and loss, revenue, customer retention, and so on. Additionally, knowledge of common use cases (e.g., predictive maintenance, supply-chain management, inventory management, personalized marketing, churn prediction, etc.) in their domain is important.
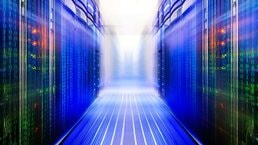
Advanced analytics: Nine insights from the C-suite
General technical fluency
In addition to their domain knowledge, translators must possess strong acumen in quantitative analytics and structured problem solving. They often have a formal STEM (science, technology, engineering, and mathematics) background or self-taught knowledge in a STEM field. And while they don’t necessarily need to be able to build quantitative models, they do need to know what types of models are available (e.g., deep learning versus logistic regression) and to what business problems they can be applied. Translators must also be able to interpret model results and identify potential model errors, such as overfitting.
Project-management skills
A mastery of process-management skills is a must. Translators should be able to direct an analytics initiative from ideation through production and adoption and have an understanding of the life cycle of an analytics initiative and the common pitfalls.
An entrepreneurial spirit
In addition to these “teachable” skill sets, translators also should have an entrepreneurial mind-set. They need the enthusiasm, commitment, and business savvy to navigate the many technical, political, and organizational roadblocks that can emerge. This is often less teachable—or at least less straightforwardly so—and the availability of entrepreneurial individuals can depend in part on the organization’s culture.
Where can organizations find translators?
Given the urgent need for translators, hiring externally might seem like the quickest fix. However, new hires lack the most important quality of a successful translator: deep company knowledge. As a result, training existing employees often proves to be the best option for filling the translator void.
Of course, this route presents its own challenges, considering there are currently no certifications or degrees for translators. In response, many companies have created their own translator academies. One global steel company, for example, is training 300 managers in a one-year learning program. At McKinsey, we’ve even created an academy in our own firm, training 1,000 translators in the past year.
Academy curricula frequently ranges from exploring the art of the possible to studying specific AI techniques and methods. Formats include both courses and immersion. Some organizations train translators through apprenticeships in multifunctional, agile teams on real AI and analytics transformation projects. These companies often combine apprenticeship programs with an academy, designing deliberate learning journeys, typically a year in length, for each individual.
Who is currently responsible in your organization for connecting AI and analytics with business goals? In many organizations, data professionals and business leaders often struggle to articulate their needs in a language that the other can execute on.
Translators bring a unique skill set to help businesses increase the return on investment for their analytics initiatives. They’re instrumental in identifying, from the myriad possible opportunities, which are the right opportunities to pursue, and they can help ensure that all participants, from data professionals to business executives, work in harmony to realize the promise these technologies offer.
This article first ran in Harvard Business Review.