Artificial intelligence (AI) is the word on everyone’s lips. But in the automotive industry today, many products and services being labeled as such are in fact reliant on a form of advanced analytics (evolving from conventional algorithms) that enables those features—for example, predictive maintenance in manufacturing.
Stay current on your favorite topics
Theories of AI have existed since 1950. However, AI itself gained wider functional applicability only in the past few decades, with the rise of machine learning and deep learning. This has also been facilitated by advances such as improved algorithms and training methods, greater computing power, and the availability of large amounts of data in the cloud.
Despite these developments, the automotive industry is still only at the beginning of the AI disruption. State-of-the-art AI applications remain narrow—they can perform better than humans, but only in very specific tasks. And the level and nature of AI technology varies widely; for example, “narrow AI” encompasses classic navigation systems as well as autonomous-driving tasks processing one gigabyte of data per second, or a million times more data than current navigation systems handle. Matching human ability in an even larger number of contexts is still some years out.
For all the talk of what AI may be able to achieve, the question remains: Is it all hype, or an important technology that companies must master? A next-level improvement from AI could drive huge competitive advantage, and this is particularly true for the automotive and mobility industry. To understand the landscape, McKinsey surveyed 3,000 consumers in China, Germany, and the United States; interviewed industry leaders, including automotive incumbents, tech players, and academics; and analyzed start-ups, investments, and patents. This allowed us to develop a view on what seems likely from a market—not a theoretical—perspective.
In this article, we focus on AI systems that use machine-learning and deep-learning techniques to enhance or create new applications in the automotive industry. Players must contend with several questions on the technology and business of machine learning in automotive and mobility:
- How important is machine learning for the industry?
- Are consumers receptive to using AI in mobility, and what are the core applications of machine-learning technology in the space?
- What challenges must be tackled to monetize the technology?
- Which strategic actions might automotive and mobility players take to prepare?
Beyond the hype, machine learning could be a source of competitive advantage
Machine learning makes AI possible. Applying a practical working definition, the technology is able to deliver in three key areas in automotive and mobility:
- act in highly complex situations (as measured by the amount of data needed to describe it)
- cope with a high number of possible situations that cannot be covered adequately by explicit programming
- improve over time without explicit instructions, learning from data of previously unknown situations in an unstructured way
For AI in mobility, machine learning will not be optional; it will be the technological foundation and the source of significant competitive advantage for decades to come. For example, machine learning is required for autonomous driving (AD), at the very least in image recognition, where human programming cannot possibly keep up. Human-level image recognition typically requires systems with tens of millions of parameters that are trained on a supercomputer for two to four weeks—a task that would take 1,000 to 3,000 years if done manually by a person.
Consumers are more open to machine-learning applications than expected
One clear finding of the research bodes well for AI’s development: consumers expect AI to have a large impact and enable broader change (Exhibit 1). Specifically, and contrary to expectations, most are open to the ways AI might improve their lives—only 25 percent see a major risk associated with AI. This openness is primarily driven by the increased levels of comfort and convenience offered by AI applications. For example, 75 percent of consumers say they would be interested in having an AI-driven robot do housework.
Consumers’ general interest in and acceptance of AI extends to the mobility space. We looked at how comfortable people are with the technology’s application to AD, and 47 percent say they would feel good if family members used fully autonomous vehicles. This sentiment is especially prevalent among Chinese consumers, young people, and those living in urban areas.
This overall interest in what AI could enable in automotive and mobility technology leads to a considerable willingness to pay for those features. Of the consumers who indicated high interest in AD features (24 percent of those surveyed), 46 percent are willing to pay more than $4,000 for autonomous-driving features on their next car. And AD features are so important to consumers that 65 percent would switch OEMs for better AD functionality; that figure exceeds 90 percent for young consumers and those living in large cities. Expectations are high, though, and may need to be tempered. On average, consumers expect full autonomy to be widespread in about five years—a tight timeline for any player, and for regulators.
Machine learning will have a significant impact on the automotive and mobility industry, since it will unlock entirely new products and value pools and not just lead to productivity improvements. Given consumer interest, we identified a number of areas in automotive and mobility where machine learning could be applied (Exhibit 2). They fall into three distinct categories:
- process optimization and increased productivity (often rooted in advanced analytics but enhanced by machine learning)
- new or enhanced products (mainly enabled by machine learning)
- entirely new (vertical) businesses, along customer use cases, making use of these new products
In the rest of this article, we focus on the applications that could create the largest value pools—autonomous driving and in-vehicle entertainment.
Would you like to learn more about the McKinsey Center for Future Mobility?
Automotive players need to overcome three challenges to benefit from AI
Applying machine-learning technology in a mobility environment is so complex that it will require new structures around the mobility ecosystem (Exhibit 3). The diverse stakeholders in the new industry landscape and the technology that supports it will require completely new commitments, systems, and ways of working. There will be a number of different machine learning–enabled systems in and around the car—some embedded, with occasional communication and updates to a back end, and some cloud based, pushed real time to the car. All these systems rely on a wide set of stakeholders (including third parties) and specific technology constraints, and they’re also influenced by local circumstances and regulation.
Here, we see three kinds of challenges: taking on technological challenges, especially around embedding technology in vehicles; meeting regulatory standards; and defining appropriate business models.
Technology. Many of the underlying algorithms and approaches for machine learning in mobility are already available in theory. Significant gains still have to be made in implementing and embedding the technology, though. Take autonomous driving and in-vehicle experience, for example: these systems will rely on amounts of data that are too large to transmit in their entirety, and the quality of output and timing are critical and affect safety. While machine-learning technology can already run in the back-end environment (sufficient computing power and appropriate hardware exist), embedding it requires technological hardware advancements and connection solutions.
Approximately 500 companies in machine learning in the automotive and mobility ecosystem are working to build the technology know-how necessary to claim their positions in the value chain (Exhibit 4). Total disclosed investments of around $52 billion have been made in those companies since 2010 (Exhibit 5), exceeding the $32 billion in disclosed investments in all shared-mobility and e-hailing start-ups in the same time frame. Nearly all of those investments (97 percent) have come from non-automotive players—such as private-equity and venture-capital firms, as well as tech players. And money has largely gone to full-solutions providers developing autonomous driving and in-vehicle entertainment. The timing of these investments shows that the race is accelerating: investments for 2014 to 2017 were four times higher than for 2010 to 2013.
Regulations and standards. “Automotive grade” requires higher safety standards and more accuracy than many current machine-learning use cases. We would expect machine learning to be used for specific tasks, however, in combination with conventionally programmed guardrails to ensure automotive-grade safety and quality. In general, for automotive and mobility, regulatory involvement is likely, together with coordination of industry standards to allow for scale and for the integration of various systems (for example, OEMs and traffic-management systems). Players in the space might actually shape these standards early on during the development of the technology and its applications, as some did when antilock braking systems were developed.
Regulations and circumstances—for instance, infrastructure—differ by region and even by city. Autonomous driving will most likely be rolled out gradually, city by city. Moreover, standards for many elements, such as interfaces and data types, differ between stakeholders. These conditions require a high degree of adaptability.
Business model. The emergence of new business models poses a challenge for traditional OEMs. Many of these new business models might shift the OEMs’ businesses more toward B2B relations (for example, fleet sales or mobility services to municipalities), which alone could put additional pressure on margins. On the other hand, the emergence of new services will require vehicles to become more software-centered and, in an end state, more like smartphones—the latest updates are a must-have.
Putting machine learning on the road requires smart moves
Artificial intelligence could be a significant source of competitive advantage, and there are ample opportunities in mobility. But progress won’t come without challenges. Nonetheless, automotive OEMs are well positioned to profit from developments in AI, especially due to the embedding control point. Furthermore, a majority of consumers are looking to car manufacturers to lead the development of autonomous functions and expect them, rather than tech players, to bring those to market—interestingly, particularly in China (Exhibit 6).
Using these advantages, OEMs can make five smart moves now to leverage their position for success:
1. Focus on core application areas. In determining the appropriateness of developing various machine-learning application areas in automotive and mobility, OEMs should consider a few aspects:
- Profit pool. What will end users find most relevant or demand most, and what will be the size of the new revenue pools?
- Competitive landscape. How many and what other players do we expect to play in this field? What advantages do they have over us?
- Market position. Where do we stand vis-à-vis others (for instance, are we the first mover, always with the newest technology), and what do we need to offer from a strategic point of view?
- Control-point logic. Which control points do we naturally own or have access to?
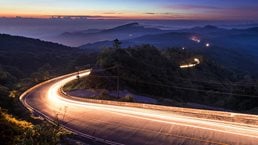
The automotive revolution is speeding up
2. Leverage breadth of data. To create new business models, companies need to better understand consumers by improving their collection of data from consumers. This is, for example, absolutely necessary for the development of autonomous-driving technology. Furthermore, creating application areas (such as traffic guidance) that rely heavily on data collection might require the development of standards across OEMs to enable data sharing.
3. Drive standards. Proactively shape new standards, together with other automotive players and the government.
4. Grow tech and business partnerships. Business models will often require capabilities that are not available in-house. For areas that are long-term sources of differentiation, select an appropriate partner to gain access to the required technology or customers. For areas where the value is shorter term, select or build up suppliers.
5. Business-model hedging. There will be a plethora of potential new business models. Build business cases and select from available business models based on the degree to which you want to be involved. Try different business models early, learn by doing, and expect to fail in certain areas.
Download the full report on which this article is based, Smart moves required—the road towards artificial intelligence in mobility (PDF–890KB).