Over the past decade-plus, data rose to become a major source of competitive differentiation for businesses. The COVID-19 pandemic arguably skyrocketed the value of data even higher as it guided businesses, governments, and health professionals to target interventions that aimed to protect and save human lives. And now, as the economic fallout of the COVID-19 crisis threatens the health of organizations, data will once again play a critical role.
While leaders can be assured that the uneven recovery from the pandemic will be digital, they’ll need to answer many questions as they work to secure their organizations’ financial footing and discern new sources of growth: Which sectors and segments will drive demand? Where is the supply chain most exposed? What is the best way to serve a more digitally engaged customer base and a workforce that is likely to continue to desire remote and flexible arrangements?
Answering all of these questions requires lots of data and the know-how to use it effectively. Businesses will need to model information from more sources, apply insights over more channels, and do all of this continuously while ensuring that the data are clean, privacy is protected, and compliance responsibilities are met.
Building the capabilities to do this comes with a cost. Most companies will have to modernize their data architecture, ingest data from novel sources, design algorithms to model data and derive insights, and hire or train the talent to do it all. The price tag for these efforts can run from hundreds of millions of dollars for a midsize organization to billions of dollars for the largest companies. Before the COVID-19 crisis, many organizations were projecting the need for more data investment, and the crisis has likely only increased this need (Exhibit 1). With bottom lines already under pressure from the pandemic’s economic fallout, businesses might wonder where they can find the resources to meet that funding requirement.
The answer, surprisingly, may come from better managing the data. Applying greater management discipline to what can often be sprawling data-architecture, -sourcing, and -use practices can unlock significant savings. Our client work shows that by enabling greater visibility, standardization, and oversight in five areas, companies can recover and redeploy as much as 35 percent of their current data spend. Even better, many of the recommended improvements can be applied quickly. In cases we have seen, businesses have captured double-digit savings within six months. Institutionalizing and expanding these changes can lead to bigger gains over the long term.
Would you like to learn more about McKinsey Technology?
Data may be abundant, but managing data isn’t cheap
Many organizations are unaware of just how much they are spending on data because costs are diffused across the enterprise. Third-party data expenditures might come out of the business unit’s budget, for example, while reporting cost resides in relevant corporate functions, and data-architecture spend is managed in IT.
When pulled together, the tally can be jarring. A midsize institution with $5 billion of operating costs, for example, spends more than $250 million on data across third-party data sourcing, architecture, governance, and consumption (Exhibit 2). How data cost breaks down across these four areas of spending can vary across industries. For example, industries such as consumer packaged goods that don’t directly engage customers often have a higher relative spend on data sourcing. The result, however, remains the same: managing data is a large source of cost at most organizations.
Addressing this fragmentation can deliver quick wins. Targeted improvements in data sourcing, architecture, governance, and consumption can help companies tamp down waste and manual effort and put high-quality data within easier reach. These efforts can cut annual data spend by 5 to 15 percent in the short term (Exhibit 3). Longer term, companies can nearly double that savings rate by redesigning and automating core processes, integrating advanced technologies, and embedding new ways of working. To get these benefits, here are the four things that leaders need to do.
Optimize third-party data procurement
After crunching the numbers, a regional bank in the United States discovered it was spending about $100 million annually to procure credit-risk data and market data, among other external data. To fund wider data transformation, it had to bring that figure down. The bank began by taking inventory of all the different data feeds it licensed and how frequently they were used. It found that a handful of third-party data sources accounted for the majority of all use, and a significant percentage of data was being used by individuals whose roles did not require real-time updates. By eliminating unused and underused feeds, defining clearer permissions around data access, and allowing credit-risk scores and other proprietary data to be reused for longer periods, the bank would be able to cut data costs up to 20 percent.
Thoughtful measures like these can reduce unnecessary third-party spend. Amending existing vendor contracts and instituting usage caps for the most commonly used feeds can provide additional gains. Later, as contracts come up for renewal, companies can compare the value and pricing they’re getting against alternative data sources (the number of which is growing rapidly) to find the best match and negotiate the most favorable terms.
We also recommend setting up a central vendor-management team with business-unit- and function-level gatekeepers to oversee data subscriptions, usage terms, and renewal dates. With appropriate procurement and business sponsorship, this team can help manage demand for third-party data and optimize vendor agreements.
Simplify data architecture
A leading global bank had more than 600 data repositories in different silos across the business. Managing these repositories cost the bank $2 billion annually. Recognizing that this was unsustainable, the bank created a joint enterprise data-architecture team consisting of the CIO and relevant business leaders. Together, they agreed to simplify the data environment into 40 unique domains and standardize “golden source” repositories, allowing them to downsize and, in some cases, fully decommission data repositories. The streamlining shaved more than $400 million in annual data costs while also improving data quality, making it easier for the bank to update systems and integrate insights into its processes.
Like this bank, many mature organizations suffer from fragmented data repositories. Storing and maintaining those troves can eat up between 15 and 20 percent of the average IT budget. The lack of standardization around data-management protocols can also create a validation headache, resulting in lost time as teams chase down needed information and increased error when they use the wrong data. To get the performance they need, organizations must revisit their core data architecture.
In the short term, organizations can generate savings by optimizing infrastructure—for example, by offloading historical data to lower-cost storage, increasing server utilization, or halting renewals of server contracts. Additionally, firms can take a hard look at the entire architecture-development portfolio and slow down or stop low-priority projects while also reducing deployment of high-cost vendor resources. Likewise, companies don’t have to wait for the target architecture to begin extracting value from their data. More widespread use of application programming interfaces (APIs) can allow businesses to put the data buried within their legacy systems to work without having to design costly custom workflows.
Over the longer term, bolder, transformational shifts can generate significantly higher savings. For example, migrating data repositories to a common, modern data platform (for example, a data lake) and evolving the infrastructure to a cloud-centric design allow a company to rationalize legacy environments and reduce average capacity required to handle spikes in computation and storage. In addition, organizations can initiate changes to boost productivity more broadly—for example, employing metrics and scorecards to improve performance, automating manual activities, and nearshoring or offshoring some resources.
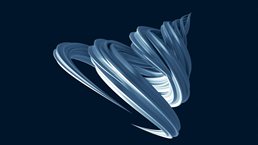
How chief data officers can navigate the COVID-19 response and beyond
Design data governance for value
A leading mining company had hundreds of sources of operational data that were scattered in small silos across multiple sites. Every new analytics use case or digital application built required months of data discovery, data ingestion, data cleansing, and data-pipe engineering, as there was little data documentation and no common standards. The company launched an integrated technology-modernization program that includes a shift from on-premises to a foundational cloud-first approach and a data operating model that builds on a federated, standards-based data architecture and disciplined domain-based data governance. This enables the creation of reusable, sustainable, and easy-to-access data assets that drastically reduce the time for data engineering and increase the stability and maintainability of applications. Data domains are developed and implemented together with the business in a use-case and value-back manner.
Our research shows that this example is not an outlier. Data users can spend between 30 and 40 percent of their time searching for data if a clear inventory of available data is not available, and they can devote 20 to 30 percent of their time to data cleansing if robust data controls are not in place. Effective data governance can alleviate these hassles. Establishing data dictionaries, creating traceable data lineage, and implementing data-quality controls can improve productivity and performance significantly.
At the same time, companies don’t want to apply so many strictures that governance itself becomes a costly impediment. By focusing the scope, aligning rigor with risk, and applying technology, organizations can help strike the right balance. Rather than attempting to govern all sources and uses of data, we recommend that organizations prioritize based on needs, value, and risk. Leading organizations, for example, often restrict the scope of data governance to fewer than 50 reports and fewer than 2,000 data elements.1
Taking compliance and other needs into account, organizations should then calibrate which activities require the most stringent data protocols and which need only basic data hygiene. A marketing organization, for instance, would likely want to employ more robust controls around sensitive customer data than it would around an event-planning database. Striking the right balance applies across all capabilities, from the breadth and depth of data dictionaries to the frequency and precision of applied data controls. For example, a North American bank that was spending more than $100 million on data lineage cut that cost by pulling back on the granularity required—going from the data-element level to the data-feed level—and running transaction testing on a sample of data elements instead to compensate.
Better use of technology can also improve performance and costs. At one North American bank, anti-money-laundering (AML) processes had a 95 percent false-positive rate. Chasing down those false positives overwhelmed the bank’s 40-person AML team. To address the issue, the chief data officer partnered with compliance and analytics on a machine-learning model that cut down on the number of false positives and reduced AML account-review efforts by 75 percent.
Streamline data consumption
In our experience, between 30 and 40 percent of the reports that businesses generate daily add little to no value. Some are duplicative, and others go unused, with the result that considerable resources are wasted.
To manage consumption more effectively, best-in-class companies map reports by topic, such as commercial reports and board reports. They then redesign data-gathering processes, automate pipelines, and explore new ways to model and visualize data and deploy the results in a paperless fashion. Rapid prototyping and testing cycles refine the report-generation process. This holistic approach helps to synthesize production across the organization, ensuring that the reports and metrics generated are of high quality and take relatively little effort to curate. Using methods like these, a European bank trimmed the number of reports it produced by 80 percent and reporting-related costs by 60 percent.
Organizations can gain additional benefits by making their business-intelligence capabilities available to employees on a self-serve basis. Remaining business-intelligence resources could then focus on more complex reporting needs and issue remediation.
Adopting data-driven approaches to optimize costs in other functions
Organizations can extract cost savings not only by improving efficiency and performance within the data function but also by applying data to identify potential cost savings in other parts of the business. Procurement is an especially promising area. Using artificial intelligence, for example, businesses could detect higher-than-average rates of energy consumption in different locations or atypical travel-cost patterns, and then use those insights to provide recommendations on how to derive greater efficiency. Likewise, specialized algorithms can scan invoices, vendor data, contract data, and service consumption to spot anomalies in the underlying spend. Such practices can help lower total procurement costs by as much as 10 percent in some organizations. For example, a European home-appliance manufacturer used advanced analytics to scan more than 12 million invoices across 5,000 suppliers, identifying opportunities to reduce total costs by 7.8 percent.
Mobilizing a data-cost-reduction program
How far and how fast an organization proceeds with a data-cost-reduction program depends on its strategic goals and the current economic climate. Some businesses may wish to apply a majority of their data cost savings to their bottom line. Others may wish to modernize their capabilities as quickly as possible. Regardless of the pace or scale, we recommend that organizations lay the groundwork with the following efforts:
- Elevate data cost as a cross-functional priority. Particular roles to include are the CFO, the chief procurement officer, heads of business, and key data and technology leaders. The support of this group is critical, given that a majority of data costs are often owned outside of the data organization and, in many cases, jointly overseen.
- Create a clear view of current spend. Develop a cross-functional baseline that accounts for both direct costs (such as licensing fees on hardware and software and compensation for employees in the data office) and indirect costs (such as effective full-time equivalents involved in managing and remediating data quality, manually compiling data for monthly reporting, and so on).
- Estimate the value at stake early, to drive focus. Rapidly identify, size, and prioritize savings opportunities by expected impact and feasibility. Invest disproportionate effort in the largest opportunities, rather than exploring every possibility.
- Establish a clear owner for the effort. This ensures accountability and effective coordination. In many organizations, data-cost programs are managed by a leader within the data organization under the chief data officer’s watch.
A program for reducing data costs can create a much more efficient data foundation, in addition to enabling near-term bottom-line impact. The effort will enable organizations to transform faster as they emerge from the COVID-19 crisis and will prepare them to stay ahead of the pack over time.