Today biopharmaceutical innovation is advancing at breakneck pace, with opportunities to address diseases once considered intractable using technologies that were previously restricted to the realm of science fiction. These advances have delivered measurable impact to the lives of hundreds of millions: for instance, US life expectancy at birth increased by 3.4 years between 1990 and 2015.1
Stay current on your favorite topics
Moreover, there is no sign of this innovation slowing. The past ten years have been exciting ones: we have seen the evolution of immuno-oncology from a niche therapy to mainstream (PD1 therapies are forecast to be worth $30 billion within five years2), the dawn of cellular therapies (with over 500 clinical assets3), and the promise of gene therapies able to target individual mutations (such as for severe combined immune deficiency). There are now over 4,000 unique assets in clinical development4 and almost 1,700 investigational new drug applications (INDs) were filed in 2016, up from 1,300 in 20075 (Exhibit 1).
What’s more, this innovation is taking place against a backdrop of unprecedented health-system change and seismic shifts in stakeholder expectations. This combination of pace and volatility has been experienced by other innovation-driven industries disrupted by the advent of digital, coupled with rising customer expectations. In most of them, we detect a strong focus on “agility” to speed the development of products that better meet customer needs. The attractiveness of agility stems from its potential to increase time spent on value-added work and ensure decisions take days, not months, to enable faster reallocation of resources and to enhance engagement—all of which ultimately translates into dramatically reduced time to market.
This applies equally to pharma where companies are actively experimenting across the value chain with how agile principles can drive a step change in performance—not least in R&D. Inevitably, given the capital-intensive nature of pharma R&D, companies require a stable “backbone” of technical competencies in any R&D operating model; however, there are significant opportunities for companies to become nimbler and to outpace the competition in bringing valuable medicines to patients.
Shifts in the pharma landscape
So why is now the time to reevaluate pharma R&D?
Diversification and acceleration of innovation
Over the past decade, R&D horizons have expanded as a wider range of players has begun to pursue innovative therapies and solutions, using a broader set of technologies.
At the heart of this democratization has been the dramatic increase in the availability of venture-capital (VC) funding for early-stage biotechs (Exhibit 2)—this has increased from $4.0 billion in investments in 2007 to $9.8 billion in 2017 (seed to stage C).6 As these projects have matured, both the number and proportion of trials initiated by non–top ten players has increased, from 4,500 trial starts in 2007 (72 percent total) to 5,900 in 2017 (85 percent total)—see Exhibit 3.7
Along with, or perhaps because of this democratization, innovation has diversified with the industry’s pipeline moving from one dominated by small molecules and monoclonal antibodies to a plethora of new technologies (Exhibit 4). Products based on cell and gene therapies now make up 12 percent of the global clinical pipeline—an 11 percent compound annual growth rate from 2007 to 2017.8
In the context of this increasingly varied and fast-moving innovation landscape, we believe the most successful players will be characterized by the following:
- Continuing to move from in-house discovery to “differentiation through identification.” Companies such as Celgene are at the vanguard of this trend, with an explicit focus on search and development. Celgene has almost 40 publicly announced R&D partnerships and a distributed R&D model with investment at the earliest stages of development in a “buy-to-build” model.9 While it is essential to maintain core capabilities in house to select the winners, a lack of unencumbered late-stage assets will push players to look for new ways to access innovation. By adopting a more “VC-like” approach, pharmacos can more nimbly enter and exit focus areas (whether defined by pathway, disease, or modality) and explore broader swathes of the innovation ecosystem over a given period.
- Increasingly virtualized R&D models. Collaboration with external partners allows pharmacos to access specific expertise and minimize the buildup of infrastructure, particularly where a portfolio is spread across multiple modalities. Indeed, we are now seeing the emergence of purely virtualized biotech where no lab or clinical work is done internally (for example, Alkeus, Velocity, Virobay, and Tioga). While major pharma companies will retain in-house capabilities, there is opportunity to further outsource both basic science and clinical research, particularly for non-core areas.
- Rapid decision making and reallocation of resources. Accelerating innovation cycles and increasingly diversified portfolios drive a need for consistent assessment of value and subsequent resource allocation. In the most competitive arenas—such as PD(L)1—success will depend on resource allocation both in terms of assets and indications, while adaptive trial design will be used to rapidly home in on winning assets and deliver a step change in speed to market.
Would you like to learn more about our Life Sciences Practice?
Explosion in volume and quality of data
The exponential growth in data and analytical capabilities continues unabated with expanding opportunities around data linkage. However, as data availability expands, so does the complexity of the required analysis, for example, to understand the biological interdependency of multiple pathways. We broadly see data being applied in three contexts within the R&D space:
- Deepening disease and patient understanding. There is an increasingly rich pool of patient data to tap into, including genomic, proteomic, microbiome, and biomarker data. The deCODE database10 has demonstrated the utility of large-scale genomic data to identify therapeutically relevant mutations, while organizations such as NashBio are developing longitudinal linked genomic and clinical data. In oncology, there is a race to use data to drive a step change in diagnosis and treatment rates: for example, the company GRAIL uses blood tests to identify circulating tumor-derived DNA (ctDNA) and diagnose asymptomatic patients based on tumor-derived peripheral nucleic acids. “Lifestyle companies” are also amassing vast pools of data: 23andMe now has genomic data on five million patients11 and iCarbonX promises longitudinal genomic, proteomic, and microbiome data based on the fitness industry. Together these patient and disease data should enable pharmacos to develop significantly tighter, evidence-driven disease hypotheses, enabling an earlier discussion on the value a medicine might deliver to patients and payers.
- Designing better therapies. A wealth of data now exists to inform lead identification and optimization, spanning drug libraries, formulation, and clinical pharmacological data sets. Founded in 2012, the British company Exscientia aims to automate drug design using machine learning to design millions of compounds and pre-assess them for potency, selectivity, and absorption, distribution, metabolism, and excretion (ADME). Such an approach has the potential to generate molecular candidates in a quarter of the time taken by traditional methods, thereby reducing the amount of preclinical experimentation required.
- Running more agile studies. Patient data sets are transforming as traditional electronic-medical-record (EMR) data sets are augmented by sophisticated linked data sets and new forms of data collection. For instance, databases, including Crossix, can link claims, EMR, prescription, and media data for over 250 million US consumers to facilitate market segmentation, channel optimization, and campaign measurement.12 Meanwhile, we also see new streams of data generation using patient sensors and disease-related apps to support patients in trials and beyond. The pharmaceutical industry is using pooled historical trials data to inform trial design (for instance, the Transcelerate Placebo SOC database13); this enables early-stage single-arm studies against matched historical controls and helps to optimize the design of inclusion/exclusion criteria and endpoints for pivotal studies. These patient data sets are allowing companies both to design accelerated clinical programs (using increasingly sophisticated surrogate endpoints) and also to course correct and accelerate programs midflight (through comparison with historical studies/records). As a consequence, clinical trials are able to move at a pace that five years ago would have been considered extraordinary.
Capitalizing on these opportunities necessitates availability of both broad and deep data sets (combining both internal and external data) as well as access to specialized analytics capabilities.
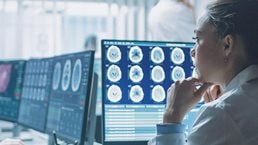
Designing an agile transformation in pharma R&D
Shifting and diverging evidence requirements for payers and regulators
The past ten years has seen patient access to medicines shaped by two, sometimes divergent, themes:
- Regulators moving faster to bring medicines to patients. Regulators are increasingly willing to expedite review processes and approve drugs on more limited data, particularly in areas of high unmet need. The number of US orphan designations has increased from 121 in 2007 to 477 in 2017,14 particularly concentrated in oncology and rare diseases (Exhibit 5). Further, accelerated pathways have led to increasingly rapid drug approvals as highlighted by the approval of Tagrisso (osimertinib), in under three years after entry into the clinic.15 In addition, real world evidence (RWE) has become increasingly central to regulators, and the FDA’s Sentinel initiative16 has expanded to include data on 193 million patients to allow rapid identification of safety signals.
- Sustained payer focus on value and evidence. In both the United States and Europe, relentless payer focus on value continues. This pressure is greatest in Europe where clinical efficacy alone is now insufficient for reimbursement and launches may be restricted to subpopulations. Looking forward, European market access pressure is expected to increase with increasingly frequent use of post-launch drug reassessments and uncertainty around combination pricing, while the 2017 Valletta declaration17 heralded a potential move toward multilateral pricing agreements. The US payer environment is also at a stage in its evolution where there is significant consolidation among both payers and providers and increasing use of care pathways, while formulary restrictions are expanding to include specialty products—all of which makes future adoption of levers such as value-based pricing more likely.
This evolving payer and regulator landscape requires companies to take a more sophisticated approach to trial design and payer engagement by considering key market and regional variations. Signs of a divergence between US and EU trial design are already evident in areas of highest pricing pressure. For example, Janssen is conducting parallel EU and US clinical programs as Darzalex (daratumumab) expands into first-line multiple myeloma therapy (based on divergent standard of care18). More broadly, successful players will be those that engage their market-access functions early, collaborate with regulators to explore new types of evidence, and build sophisticated understanding of total payer cost base.
Read our latest thinking on agile pharmaceuticals
Fundamental changes to the R&D operating model are required to position pharmaceutical companies for success
This fast-evolving external landscape, combined with sustained cost pressures and shareholder expectations, are driving pharmacos to operate in a more nimble, agile way to increase their “metabolic rate” and improve their R&D productivity.
Given the inherent complexities of R&D and the investment levels at stake, shifting the R&D operating model is tough. Nevertheless, we have observed several industry players moving swiftly to make material shifts in the way they operate and have laid out the key elements here.
Increasing the metabolic rate of ‘strategy’:
- more VC-like external sourcing model to access the broadest spectrum of scientific progress, rapidly enter and exit focus areas, and balance the internal R&D risk profile, including novel partnerships with other pharmaceutical companies, biotechs, technology players, and investors
- softening of therapeutic-area (TA) boundaries with flexibility to explore biological pathways that present opportunities across multiple disease areas
- differentiated, fit-for-purpose asset strategies based on the unique requirements of the asset and disease (for instance, use of RWE, single-arm studies, predictive biomarkers)
Flexing the ‘structure’ to meet the needs of the portfolio:
- overall organizational flexibility to deal with internal and external interchange and to allow rapid acceleration of priority programs
- flexing the makeup of project teams to optimize delivery against the asset strategy (given its experimental stage and targeted biological pathway)—this could include adding, for example, data “translators” or external partnership relationship managers to the team, and including commercial representation earlier in the life cycle (for example, in the case of accelerated-approval assets)
- reducing internal infrastructure (for instance, via external strategic partnerships) to enable greater flexibility in capability and capacity, in order to follow the innovation opportunities and to access cutting-edge technologies and nimbly explore new areas
Accelerating processes, governance, and mind-sets to drive to decisions:
- evolving governance models and systems to support real-time decision making (for example, to support asset acceleration) based on interim clinical read-outs and external opportunities/threats (rather than conforming to fixed governance cycles and a requirement for final clinical read-outs following database lock)
- embedding integrated-resource-management solutions to provide real-time insight on the progress of clinical programs, create transparency on their current resource allocation, and, importantly, enable rapid reallocation decisions informed by the potential portfolio impacts of those decisions
- resetting the governance mind-set to one where rapid decision making is seen as a key source of competitive advantage—characterized by an increased willingness to accelerate (or deprioritize) trials based on interim data
Turbocharging the operating model with data and analytics:
- ensuring a step change in the level of investment in data sets and analytical capabilities, with incubation investment potentially ring fenced
- establishing partnerships with a full gamut of tech players to access cutting-edge data sets and analytics
- embedding digital and analytical teams across the organization to allow faster and earlier decision making, empower asset-development teams, and elevate the position of digital and analytics leaders within internal governance
Embedding the capabilities for success:
- building expertise, particularly around digital and analytics, but also in core scientific areas requiring more fluid inflow (and outflow) of talent as the portfolio evolves—including with talent from beyond the industry
- shifting the mind-set to embrace different ways of working for each asset (rather than one size fits all), seeing external partners as true collaborators, and being ready to deprioritize programs when better opportunities arise (even if a project continues to meet predetermined stage gates)
- creating new incentive models linked to project success and individual performance in a project context, rather than the performance observed along functional reporting lines
Read our latest thinking on agile pharmaceuticals
Activating agile R&D
Collectively these changes would represent a fundamental shift in the R&D operating models of “traditional” players and require new ways of thinking, working, and leading. Therefore, players may elect to focus first on a specific set of enablers with priorities determined by their starting point and final aspiration. Regardless of which approach is adopted, a structured approach to any agile transformation is essential to maximize impact and minimize disruption. Typically, this includes three key steps:
- Diagnosis. Assess the current level of agility across the areas outlined above to identify areas of strength and gaps where an opportunity exists and, importantly within this, call out differences by TA, geography, or platform and identify any ongoing efforts.
- Aspiration setting. Define the target end-state and the time frame for achieving it, including laying out which agile approaches will be implemented and where in the organization—and also how success will be measured.
- Agile transformation. Adopt agile techniques to trial and refine prioritized interventions, with clear senior operational sponsorship and interim targets—then scale successful approaches at pace.
Across these areas, the breakdown of established silos is an important unifying theme. First, the concept of a TA will become increasingly less important in R&D as the interrelationships between different disease states are further clarified. Targets and technologies will be important across multiple indications, and R&D organizations must evolve to reflect this. Second, functional lines will become less important within R&D organizations as resources are fluidly deployed against projects informed by data. The makeup of these teams will be highly dependent on the needs of an asset and its development plan, and we will therefore see new combinations of functions collaborating to bring drugs to patients. Finally, the division between the internal and external world will become increasingly blurred as pharmacos build external partnerships across all aspects of development and draw on talent from beyond the payroll to bring the best-available capabilities to key projects.