Recovering past earnings is a priority and a significant challenge for banks as they continue to cope with the pandemic’s economic impact. One of the most promising methods to improve profitability is to apply advanced analytics (AA) and artificial intelligence (AI) to better manage nonpersonnel costs. In our experience, such costs account for more than 40 percent of overall expenses at the typical European bank.
Many banks have recently focused on reducing these costs, and the experience of some global banks shows potential for even greater savings. By stepping up cost optimization with AA and AI—in concert with taking a zero-based demand management approach and using a cost-management center of excellence—they have reduced expenses by 10 to 15 percent on average across cost categories—and up to 35 percent in certain categories.
Evidence for the effectiveness of the approach can be found outside of banking as well. According to the McKinsey Global Purchasing Excellence survey, which collects input from more than 1,600 companies, the most mature sectors in terms of procurement—such as consumer and automotive—apply the three approaches to achieve savings in cross-sector categories such as marketing, temporary labor, and IT. (Financial institutions, by the way, fall in the middle of the maturity pack.)
Using advanced analytics and artificial intelligence
AA and AI give banks greater transparency and insights by delving deeper into previously impenetrable cost drivers. A few players are starting to employ analytics-driven approaches by spending category along with external benchmarks to challenge business owners on general and administrative spending and capital expenditures.
Would you like to learn more about our Financial Services Practice?
A number of banks have applied these tools successfully in four types of applications:
- Data transparency. A bank used AA to combine fragmented data sets related to building maintenance—including tens of thousands of maintenance tickets, vendor data, and contract data—into a single data set. This enabled AI enrichment, cleansing, and hyper-granular categorization for unparalleled transparency into major cost drivers. With this “master spend data set,” the bank used machine-learning techniques and automated root-cause analysis to scan outliers in the spending data and rapidly identify anomalies and patterns that would trigger “warnings”; for example, they could flag maintenance ticket clusters with high chance of overpricing, allowing them to streamline and consolidate those clusters.
- Insight generation. Another bank used AA to compare the rents it was paying for its facilities with the average rent in the same micromarket.1 The results and analysis enabled the bank to identify opportunities for renegotiation, as well as mitigate risks of future price increases (Exhibit 1).
- Fact-based negotiation. A bank used a highly analytical “should cost” tool to optimize the prices paid to vendors for software development. Armed with detailed cost breakdowns based on cost-prediction algorithms, specific project schedules and constraints, type of delivery, benchmarks, and other variables, the bank negotiated with vendors to reduce their prices by 10 to 30 percent.
- Demand management. Another bank analyzed the annual number of cash-transfer trips per ATM in relation to the ATM-specific cash stock balance. The analysis revealed a low-to-medium correlation between the number of trips and the cash balance. These findings allowed the bank to run simulations to find the optimal cash stock balance for every ATM cluster (rather than a single blanket balance threshold). The optimal ATM-specific balance reduced the amount of money in stock and the total number of shipments of cash to ATMs, resulting in 14 percent savings on cash-in-transit costs (Exhibit 2).
Apply zero-based demand management
The pandemic has rapidly changed ways of working and associated costs. Lockdowns in many countries increased the need for digital services, and many banks transformed both internal and external processes in response—for example, adopting remote working for non-customer-facing activities and making more services available through nonphysical channels. This puts banks in a position now to take a disruptive approach to demand management, not only to reduce the quantity of purchased goods and services, but to embark on a zero-based review of their requirements, to be aligned to the new needs. Furthermore, many of the solutions that were adopted as rapid workarounds during the pandemic can now be made permanent (e.g., use of digital signature in electronic copies versus hard-copy originals).
In areas such as logistics, travel, and real estate, there are opportunities for significant savings. Many global companies expect to reduce travel costs to some extent with a few adopting an aggressive approach to smart working,2 which would likely lead to a significant compression of expenses by eliminating travel for internal purposes.
In spending categories not significantly affected by the pandemic (for example, building maintenance, physical security, legal expenses) or those cut temporarily (cash in transit, energy consumption, mail and courier services), banks can use a zero-based demand approach along with the new analytical tools described in the previous section to determine the right level of demand. For instance, banks could perform a bottom-up assessment of internal courier services to determine the actual need for these services at headquarters and at each branch.
In areas where costs are increasing (for example, IT devices, digital infrastructure, and telecommunications), it is important to address demand in an informed way, so that when the COVID-19 pandemic finally recedes, these areas are sized properly and resources are not wasted. For example, banks should carefully rationalize their PC and network needs to avoid overpaying for running costs and maintenance.
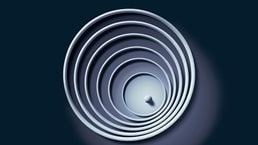
Beyond digital transformations: Modernizing core technology for the AI bank of the future
Adopt a cost-management center of excellence
To unlock all opportunities raised by AA/AI, banks need to adopt a business governance approach that embeds these cost-management best practices in their budgeting, reporting, and control processes. Since the financial crisis, many European banks have been shifting demand management to dedicated cost-management centers of excellence, which focus almost exclusively on demand while often leaving pricing negotiation to a separate procurement unit.
In our global banking cost-management benchmark, we found that banks are leaning toward a more centralized model for cost management, with a central unit and solid-line reports in the business units. They are also structuring internal cost-management units mainly by category (such as IT or real estate) and moving toward more formal “category councils”—cross-functional groups comprising interested parties for a category, including cost management, procurement, business/budget owners, and other support functions such as legal and compliance, when required. A few banks are starting to employ analytics-driven approaches per category and external benchmarks to challenge business owners on general and administrative spending as well as capital expenditures.
Our benchmark defines four archetypes of operating models of the cost-management units, by the level of control the cost-management units exert in the organization. (In Exhibit 3, the archetypes are shown left to right in ascending order of cost control.) The Accountant archetype uses a decentralized cost-management approach, focusing only on selling, general, and administrative expense (SG&A), typically with no cross-functional teams and limited cascading of objectives defined at macro level. The Challenger archetype—the one advanced players tend to adopt—is more centralized, with a broader scope of responsibility on SG&A, capital expenses, and in some cases personnel costs. This approach employs cross-functional teams, with clear price and quantity objectives and widespread adoption of business intelligence tools—in the best cases integrated with AA. Banks can use these archetypes and the associated processes, performance management approaches, and tools to guide their journey to increasing control of costs.
Digital tools and innovative cost-management techniques will be critical to competitiveness in banking. We estimate that by embracing zero-based demand management, applying AA and AI, and adopting a more centralized cost-management operating model, banks can build on their previous efforts to reduce non personnel costs and achieve about 30 percent of the cost cuts necessary to return to pre-COVID-19 levels of profitability.