As autonomous-driving technology advances, new transportation use cases will emerge, largely driven by factors such as what is transported, type of vehicle ownership, and where the vehicle operates. Use cases drive business models, value chains, and strategic decisions. Asutosh Padhi, a senior partner in McKinsey’s Chicago office, and Philipp Kampshoff, a partner in the Houston office, share the McKinsey Center for Future Mobility’s perspective on how the most prominent autonomous-driving use cases are developing to help executives navigate and stay ahead of upcoming changes.
When will autonomous-driving technology be market ready?
Asutosh Padhi: Our expectation is that true Level 5 autonomy is about ten-plus years away. But we are likely to see geofenced applications of autonomous vehicles (AVs) in the next three to five years. The progress on the hardware has actually been very significant. The cost of LIDARs [light detection and ranging sensors], for example, has dropped by a factor of ten over the last five years. Similarly, the amount of computational capacity that the GPUs [graphic processing units] can provide has gone up dramatically.
There are still two challenges that remain. The first one is object detection and categorization, which is the ability of a car, for example, to recognize a pedestrian: this is what it looks like if a pedestrian is pushing a stroller, if the pedestrian is carrying an umbrella, if the pedestrian is carrying a plant, when a pedestrian doesn’t look like a pedestrian, etc. And the second challenge is decision making. When there is human driving, there are a lot of subtle signals that drivers send to each other—right of way, etc.—and often if you’re an autonomous car, you can’t pick those up. So I think a combination of those two, and in particular the edge-cases question; there’s going to be high time required to be able to teach the car how to recognize and deal with the edge cases.
Philipp Kampshoff: That’s really the reason why we don’t see autonomous vehicles already being readily available as robo-taxis driving around. The decision making is done by the neural net in the car, at least for the large part. And you can train the neural net relatively quickly to accurately assess 95 percent of the situations. But it takes a lot more training and a lot more miles that you have to drive in order to train the neural net up to 99 percent correctness.
Edge cases is where the problem is. If you take a regular human driver, on average it’s roughly every 165,000 miles he has an accident. That means, in 99 percent of the cases, the human person is right when they drive themselves. However, for an autonomous car, regulators will require the autonomous car to be much safer than current human behavior. So it will come down to handling these edge cases—for example, the four-way traffic sign where these days hardly anybody really comes to a full stop. So do you have the autonomous vehicle be the only one that’s behaving correctly and sits there forever waiting for everybody else to stop all the way? Another example is a construction site where you have a red traffic light, and the autonomous vehicle is approaching the red traffic light, but there is a construction worker who waves the people and cars through. How does the autonomous vehicle know that it can ignore the red traffic light? So these are the kinds of edge cases that have to be overcome for autonomous vehicles to really be out there in mass adoption.
Why is testing and validation a concern for autonomous-driving-technology readiness?
Asutosh Padhi: Our view is that there’s going to be a completely new paradigm that has to emerge for testing and validation in the world of autonomous vehicles. In this new world, it is less about driving millions of miles. If you drive millions of good miles, there is, in effect, no new learning that happens. It really is about looking at the millions of edge cases that you’d expect that do not usually happen on a more traditional and a more frequent basis. And it’s about teaching the car and the algorithms to recognize object detection as well as decision making in those edge cases. And what it essentially implies is that you can’t do this through physical validation and testing, because you can’t have a car drive a million miles. You have to borrow techniques that are used around software-based simulation from other industries like gaming as a way to be able to complete the necessary level of validation.
Philipp Kampshoff: The interesting question is, “What is the driving test for autonomous vehicles going to look like before you let them on the road and work as robo-taxis or private AVs?” And for sure it’s going to be some sort of virtual testing, where you throw situations at the car and see how it would react in different situations. As a regulator, you want to keep on changing that so you cannot program the autonomous-vehicle software so it recognizes the pattern. And then you’ll also need some sort of a physical driving test that these vehicles have to undergo before they’re going to be allowed on the road.
Would you like to learn more about the McKinsey Center for Future Mobility?
One important thing that people often forget is it’s not only about the first time that they’re allowed on the road, but then how do you make sure they are properly maintained? It’s like in the airline industries, where before the airplane can actually take off, it has to go through certain security checks and safety checks to make sure all the parts are in place and everything is running smoothly. It’s going to be somewhat similar for autonomous vehicles, where you really have to make sure, at any point in time, your sensors and other components that you need for the car to function properly are working before you let the car out on the street.
What is your view on vehicle-to-infrastructure (V2I) technology: Necessity, or nice-to-have?
Philipp Kampshoff: The answer is not as straightforward as yes or no. It really depends on which situations, and it also depends on which manufacturer you’re talking to. Some of the manufacturers will say, “We are trying to build cars that are self-sufficient. We don’t want to rely on anything else. We want our cars to be able to behave correctly in any given situation, without having to rely on the right infrastructure around it.”
There are some use cases that would make it a lot easier for the technology and for autonomous vehicles to be out there if V2I were available. One of the examples is the car approaching a construction site. There’s a red traffic light, and the construction worker waves you through. How does the car know that this is actually a person of authority? You could give the person, the construction worker or a policeman, a chip that basically signals very clearly, “I’m a person of authority, and I have the right to override the red traffic light.” So in these types of situations, V2I would make things a lot easier for the autonomous car. So coming back to your question, it’s not a clear-cut answer. Manufacturers will try to make the cars as self-sufficient as possible.
But we believe there is going to be some sort of V2I, especially in the beginning, as technology is probably, in some of instances, not quite ready. It might also depend on the geography. There might be cities, that are just being built right now where it’s a lot easier to put in this infrastructure, since sometimes, in more traditional cities, it’s harder. And the cars will have to rely more on their own rather than on new infrastructure.
Another example for V2I or application of autonomous vehicles in combination with infrastructure adjustments would be, for example, a scenario where you say, “Hey, let’s use what we currently have as high-occupancy-vehicle (HOV) lanes, and we make them autonomous-vehicle lanes.” Basically, you have a car that has the potential of driving autonomously, and before you enter that lane, you have to activate your autonomous mode. And while you’re driving in the HOV lane or, in that case, the AV lane, the car is going to drive autonomously. Obviously, you need the infrastructure that checks with the car, “Is the car really on AV mode?” If you assume that these AV cars are not crashing as often because they communicate with each other and react quicker than a human would react, you can actually allow them to go faster than regular cars. So, you might have AV lanes where cars, instead of just going 60 or 70 miles an hour, go 120 to 150 miles an hour toward downtown, based on this combination between AVs and infrastructure.
What type of autonomous-driving use cases do you expect to see in the future?
Asutosh Padhi: The Society of Automotive Engineers’ Level 1 to Level 5 taxonomy is very helpful to describe the technological evolution of autonomous vehicles. However, as you think about commercial applications and policy making, a use-case-based approach is more helpful. We are going to see a spectrum of use cases that emerge from autonomous vehicles. The first element of the use-case-based approach is, “Where will the autonomous vehicles operate?” Requirements for hardware and software are very different for driving in urban environments versus highway-type environments. The second element is, “Who owns these vehicles?” Is it going to be individuals, or are they going to be owned by private fleets? This is important, since the operating models and the economics are going to be very different. The final element is, “What is being transported?” Is it individuals, or is it different types of goods? Depending upon the choice to all of these, we’re going to see a whole different set of applications and use cases that will happen over the next few years.
Philipp Kampshoff: Let me tie all these elements together to give you a couple of examples of these use cases. If we talk about transport of people in an urban environment [with AVs] owned by a professional company instead of a private person, then we are talking about the use case of robo-taxis.
One question that we are always being asked is, “Is there going to be an Airbnb model of autonomous cars in the future, where people own autonomous vehicles, the autonomous vehicle takes them to work, and then they put it into the mobility-as-a-service system to work for them?” The math suggests that it is difficult for this vehicle to compete with a professional-fleet provider. The reason is that the professional-fleet provider, just because of economies of scale and purchasing power of not buying just one car but many, will get a different purchase price. They will be much more professional in terms of servicing and maintaining the fleet. And likely, the utilization of the car is going to be higher, too. So they will be able to operate at a different cost point in comparison to a private person who owns an AV and brings that into the system to work as a robo-taxi. Most likely we do not see the Airbnb model for robo-taxis, but professional-fleet providers providing that.
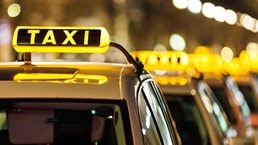
Gauging the disruptive power of robo-taxis in autonomous driving
Other examples of use cases would be highway application for long distances for goods transport. We talk about “platooning,” at least as a bridging technology, where you have two trucks, and the second one platoons behind the first one. You don’t need a driver in the second one anymore. It just brakes and accelerates in line with the one that’s in front of it. You will have two trucks in the beginning; potentially, it will increase to a train of different trucks. Eventually, you will be able to take the driver out of each one of these trucks. When we look at the numbers, initially platooning is going to help with the total cost of ownership for a fleet provider, driving down 10 percent of the cost. Later on, when you take out all the drivers and you have this concept of platooning trains, you can go as high as 40 percent reduction on total cost of ownership.
How will robo-taxis disrupt existing mobility models?
Philipp Kampshoff: Robo-taxis have huge potential to disrupt the industry as we know it and to encourage people to go away from private ownership to using robo-taxis 100 percent of the time. We’re going to see that most likely in urban environments than in rural areas. In comparison to private ownership today, if you live in an urban environment, robo-taxis can be 30 to 50 percent cheaper for you, using robo-taxis at all points in time rather than owning a car. And that is if you factor in all the different costs around insurance, parking, and so forth. When is that going to happen? We think the true disruption is going to happen as soon as these robo-taxis are able to operate in not only an urban environment but also in suburbia or on highways.
Highways right now are a little bit of a challenging environment, mainly since you’re traveling at much higher speeds. In an urban environment, if the car doesn’t know what to do, it can, in theory, park at the side and wait and figure out what it needs to do next. But on a highway, when you go 60 miles an hour, for example, that’s not possible. In terms of object detection, the car needs to be able to recognize exactly what is in front of the car. Imagine something falls off the truck in front of you; the car needs to be able to say, “Is that a paper bag, or is that a rock?” And based on whatever it detects, it needs to make the right decision of either going around the object or just driving through it.
What does it take to be a leader in the robo-taxi market?
Philipp Kampshoff: The robo-taxi market is going to be a very interesting and attractive market. We see that already today with the movement toward shared mobility, where this is only going to pick up as we move toward robo-taxis. The market today is already 50 billion, and it will be a lot bigger once we move to robo-taxis. So the market is going to be competitive, and it’s not that simple, at this point in time, to say who is going to be the winner going forward.
If you come from the customer angle, there is a good question around why you choose one provider over another. One of the key reasons you would choose one provider over another is availability of cars. Once you call a shared-mobility provider, how long does it take for the robo-taxi to actually show up at your house? So the shorter that time is, the more attractive the provider is for the customer. And in order to address that, scale is going to be important. If you have a large fleet and you can provide good coverage of the network, then obviously that is going to be helpful. The other angle is the price. If two cars are at my house at the same point in time, and the cars are basically the same, then I would probably go for the one that is cheaper.
Now if we look at the operations side of things, cooperations are going to be very important—so having the right technology in terms of autonomous vehicles, having access to different car options. Maybe you don’t want to have the same car, the same layout, of every robo-taxi. But robo-taxis are maybe more purpose-built for certain applications. So you want to have more flexibility there. All of a sudden you’re in the business of managing a fleet of thousands of cars, maybe similar to rental cars today, where you actually have to maintain and service a huge fleet of cars at any given point in time. So being lean in your operations, making sure you service your cars in the most effective way, is going to be very critical for these companies.
Will advanced driver-assistance systems still be relevant?
Asutosh Padhi: ADAS stands for advanced driver-assistance systems. Our view is that ADAS is going to be very important on the path toward full autonomy. That’s because there are a number of different features that are already in place, and you largely see these in more premium products that we believe can actually pay for the path toward full autonomy. ADAS teaches consumers how to be able to use these different features, and this makes it affordable for the OEMs and suppliers to invest in these technologies.
The current revenue generation from ADAS is pretty limited, but, going forward, our view is that the revenue potential is actually going to go up dramatically. How does that happen? It happens by expanding the usage of these features from premium products to more mass vehicles. It happens by automotive companies making it easier to partner with third parties, like insurance companies around variable insurance for car drivers. It comes through working with other regulatory agencies and informing customers of the benefits of these different features. And frankly, it will happen by expanding usage of these features in emerging markets, particularly markets like China. I think there’s a very high degree of appetite for ADAS-like features and a high customer willingness to pay for them.