When a company undertakes a merger or acquisition, the CEO and steering committee go down a familiar path. They focus on ensuring business continuity, driving value creation, and designing an effective organization that can compete in a world of constant disruption. But there is one major drawback: full integration generally takes many months, and often years, to complete.
Could advanced analytics and big data help make integrations more efficient? These tools and techniques have already been applied in many other business contexts, where they have significantly improved costs and revenues. Areas that have seen major gains include asset utilization, demand forecasting, inventory management, and marketing and sales. There’s no reason why companies couldn’t apply the same techniques in mergers and acquisitions, where companies strive for improvement in the same areas.
Some recent shifts make this the perfect time for companies to take this step. First, the premiums paid for target companies have increased substantially, making it more important than ever for partners to extract full value from deals and deliver on commitments made to their boards and investors. Second, about 90 percent of the world’s available data has been generated in the past few years.1 Meanwhile, data-storage costs have drastically decreased, and processing power has soared. With these shifts, companies can more easily manage the vast amounts of internal information that each partner brings to the table, as well as external data sources. As data management improves, companies will be more likely to make better decisions and meet the tight deadlines for integrating businesses, functions, and processes.
With few companies now applying advanced analytics during M&A, their benefits are not discussed in business-school case libraries describing best practices for integrations. To explore the opportunity, specifically within the integration phase, we examined a McKinsey case-study database for almost 200 companies that applied analytics to solve pressing business problems, focusing on those issues likely to crop up during M&A. (Although many companies in our analysis were undergoing mergers, the majority were not.) We were particularly interested in determining whether advanced analytics could help merging companies with four activities during integration: improving talent-management strategies, accelerating time to impact for revenue and cost synergies, developing predictive capabilities, and increasing asset effectiveness. Our analysis suggests that advanced analytics has the potential to improve all four areas during integration, which could accelerate time to impact and increase deal volume.
Of course, caution is required when applying advanced analytics during M&A. The period before closing is particularly sensitive, since there are strict limitations on the type and depth of data that deal parties can share. Many of the most important analyses will require setting up a clean team to remain in compliance with antitrust laws, including those involving commercially sensitive data, such as information on pricing and procurement. Legal counsel can provide guidance.
Enhancing the M&A value chain
Although advanced analytics are already unlocking value within business, their potential remains largely untapped. Only 8 percent of businesses now engage in practices that support the widespread adoption of these tools during any activity.2 But companies that continue to hesitate may lose out. For instance, McKinsey Global Institute estimates that companies could generate $9.5 trillion to $15.4 trillion in business value by investing in artificial-intelligence tools, including those that have a central role in advanced analytics.3
For M&A, an area where few companies now apply advanced analytics, there is the potential to enhance all activities. During due diligence, companies may mine new insights from external data, if available. These analyses may be an important source of additional insights, since companies have limited access to internal data during the due-diligence phase. Advanced analytics may also uncover opportunities for synergy that would have otherwise been overlooked. At the negotiation stage, when transaction documents are being created, companies can use behavioral analytics to understand their potential partners more thoroughly. With this knowledge, they can improve their negotiation strategy. Finally, when the deal is signed, companies can apply advanced analytics to derive maximum value from the transaction. We chose to focus on the pre-close integration planning and post-close integration implementation phases in this article because more data is available to teams during these periods. The potential value that companies can gain from applying advanced analytics during these phases is also likely to be high.
Improving talent management in a competitive market
Acquiring companies may have little information about the workforce they inherit from their targets, including the employees who are truly creating value. Leaders may also be somewhat unfamiliar with the new markets and segments that they’re entering, including the skills required to compete. That could leave them with talent gaps in critical areas.
Using advanced analytics, companies can move beyond traditional talent-acquisition, development, and retention strategies during mergers. And with greater computing power, they’ll be able to assess a much wider range of data, including information from external sources that companies tended to overlook because they didn’t have the capacity to collect, clean, and analyze it while a merger was underway. Here’s how it works.
Talent acquisition
In many fields, top talent is hard to find using traditional recruitment approaches. But big data and advanced analytics can help companies find untapped sources and understand the scale of the challenges involved in getting the right talent in specific markets. In one recent merger, a conglomerate acquired a technology company and committed to increase the technical talent at this target over a specified period of time. The company’s core strategy, and the main reason for the acquisition, was related to its desire to expand into the Internet of Things (IoT), so the talent strategy specified that new recruits should have skills in this area. After the company applied advanced analytics to the local talent pool, it became clear that it would be very difficult to find enough people with the appropriate IoT skills. In fact, to meet its target, the company would have had to recruit over 80 percent of the local IoT talent. In response, the company decided to focus recruitment efforts on technical employees in general, not simply those with IoT skills. Other companies that are recruiting talent can conduct similar analyses, ideally early in the deal process, to understand the available market, including the educational institutions that might produce appropriate graduates. Given the increasing scarcity of technical and digital talent for critical roles, as well as the rising cost of recruitment, such analyses will become even more important in the future.
Similarly, an IT-services company wanted to strengthen its presence in a complex market niche with rapid growth and high margins. The company had little experience in this segment and knew that it would take a long time to build the required internal capabilities and establish a strong presence. It therefore opted to buy a company to facilitate an immediate market entrance and quickly gain scale. While the company considered a range of acquisition targets, many of them were relatively small and had limited information available about their operations or financials.
Would you like to learn more about our Strategy & Corporate Finance Practice?
With such opacity, the company could not determine which target was best positioned for the future. It had a hunch that internal talent differentiated the winners from the pack in the target segment and turned to advanced analytics to verify this hypothesis. First, the company compared the LinkedIn profiles for its own key staff to those of employees at competitors who attracted many of the desired accounts in the target segment. The company used topic modeling, a text-mining tool that discovers hidden semantic structures within text. In this case, topic modeling sifted through the thousands of skills listed on LinkedIn and identified those that were likely to be found together on the same profile. With this information, the company could compare employees based on which group of skills they possessed. After conducting this analysis, the company compared the profiles of its account managers to those of employees at successful companies to determine if they differed with respect to skill groups. It soon became clear that skill groups for account managers at successful companies were very different from those of the company’s current account managers. This information was vital, since the company’s main M&A goal was to improve its talent base. Without a clear idea of the most important skills, it would have difficulty targeting appropriate businesses.
After targeting and acquiring a business with many talented account managers, the company used insights from advanced analytics to create customized retention plans for the best employees to ensure that the deal generated maximum value.
Talent development
In addition to performance reviews, seniority, and education levels, data scientists can examine detailed operational and financial metrics to gauge an employee’s contributions. Within sales, they might look at the number of customers a representative contacted, the frequency of their interactions, and the number of contracts signed. Timesheets could provide clues about whether employees are spending time with the most valuable clients or focusing on accounts that don’t add much to the bottom line. When all data points are considered in combination, companies can identify their top talent or those with the potential to be leaders. With the explosion of data over the past few years, and with more innovative algorithms available, advanced analytics can now deliver more sophisticated insights based on this information. Across industries, companies have already used advanced analytics to improve talent development, although most examples are not within an M&A context. For instance, one insurer was able to identify the top 10 percent of its employees across different roles, departments, and branches. The insights it obtained during the analysis allowed it to improve its performance-evaluation system. Similarly, a telco achieved €15 million in productivity improvements by using advanced analytics to identify high-value employees and then providing them with additional training.
During M&A, clean teams might be able to analyze some confidential data about employees at the target business that can help them identify the most valuable staff. If such teams do not exist, companies can prepare for integration by cleaning and analyzing data on their internal employees. The resulting insights will help them move quickly after closing.
Talent retention
It’s not enough to identify the best employees—companies also have to retain them, and that can be challenging during a merger, when many staff begin looking for new jobs because they fear change or don’t see a future with the new business. In our experience, companies that don’t undertake extensive retention efforts often lose up to 70 percent of their senior managers in the first five years after a merger. This is about twice the attrition rate for companies that haven’t undertaken deals.
Leaders often try to stem the flood by developing a targeted retention plan for critical employees, but they don’t know which roles are driving the most value or which employees contribute most to their organization. To counter this problem, companies should analyze various data to determine which roles are most critical to value creation. They should then identify top performers using traditional methods, such as examination of performance reviews. But companies should also use advanced analytics to assess performance, since traditional reviews may overlook the employees who are generating most value. Such disconnects are extremely common because executives often rank employees based on seniority and personal relationships, or simply rely on intuition. With these subjective assessments, the people who contribute the most to the bottom line may get shortchanged.
Advanced analytics can also highlight the employees most at risk of leaving. A review of online job ads might show that R&D scientists are in high demand in the local area, for example, suggesting that they are likely to be recruited elsewhere. Or an analysis of LinkedIn profiles of current employees might show that many staff have recently updated their profiles or expanded their description of skills—activities that often precede a job hunt.
Once companies have identified their critical employees and those at risk of leaving, they can develop targeted interventions, rather than offering blanket incentives to all staff. For instance, the CEO could call the top 20 scientists at the company or managers could increase salaries for critical roles.
Accelerating time to impact
Even amid the chaos of a deal, advanced analytics can help companies improve some of their most important operations, business processes, and functions—and that could accelerate integration timelines.
Consider how advanced analytics could help accelerate product development—one of the most crucial activities in many industries, such as pharmaceuticals and high tech. Businesses could have future blockbusters that are in the works, but it’s not easy to prioritize R&D activities and keep them on track as companies go through a merger process. The merging companies may have conflicting R&D agendas, making it difficult to determine how the new company should set priorities. Employees may also struggle to keep pace, since they may be grappling with additional responsibilities or adapting to new processes. And even if products do move through the R&D funnel at the expected rate, they might not launch until well after the deal is complete.
By applying advanced analytics, companies can integrate and narrow their R&D pipelines more rapidly. For instance, two pharmaceutical companies that merge may each have dozens or hundreds of products at various stages of development. If they can weed out the weaker candidates more rapidly by applying advanced analytics during early clinical trials, the new company will allocate its R&D spending much more effectively.
During one improvement initiative, a pharmaceutical company used advanced analytics to improve the method for evaluating drugs in clinical trials. It looked at internal data for each R&D site to determine if certain locations had a history of problematic trials or high costs in different therapeutic areas. The resulting information helped the company create a predictive model to determine the best sites for future trials. Data scientists also developed models to optimize clinical-trial enrollment, identify risks to quality, and predict time to completion. These models helped the pharmaceutical company reduce time to market by 15 percent and clinical-trial costs by 11 percent. In a merger, pharmaceutical companies could use similar techniques to determine which R&D sites should be used for future trials or even assess which sites should remain open, especially if there are multiple locations working in the same therapeutic area.
Enhancing predictive capabilities in a time of uncertainty
Before a deal closes, top management may have limited insight into some of the most important aspects of their target, such as the breadth and depth of staff talent or the prevailing company culture. Such knowledge gaps may compromise forecast accuracy and inhibit their ability to make fact-based decisions. After a merger is complete, leaders can access more information, but they’re under pressure to make decisions quickly and simply don’t have time to conduct in-depth analyses on every topic.
Advanced analytics can help sort through the confusion and obtain better insights—and one area where such knowledge is badly needed is customer retention. As companies merge, their competitors may use this busy time as an opportunity to pounce. If loyal customers find the new company unfamiliar or lacking in some way, they’ll be more likely to move. For instance, customers may be confused about how they will be served, what terms will be offered, and what channels are available for communication. For all these reasons, the new business must quickly develop strong customer-retention strategies.
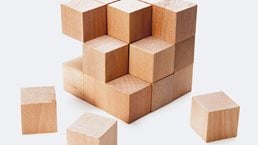
Advanced analytics in asset management: Beyond the buzz
In one case, a retail bank was experiencing increased churn following a merger. In addition to settling the deal, the bank was grappling with greater competition, falling interest rates, and growing broker activity. After developing models to determine which customers were most likely to leave, it was able to identify the underlying drivers of churn. The bank then created targeted retention strategies for those segments most likely to churn. After testing the strategies in the field, the bank fine-tuned the predictive model and the retention strategy. The accuracy of the predictive model increased threefold between the first deployment and the fourth. Overall, the bank reduced the churn rate by 20 percent.
Increasing asset effectiveness
“Good assets, poorly run” is the phrase often used when describing an acquisition target. The assets in question may be machinery, factories, or some other tangible structure, but in the world of merger management, they could also include employees or functional groups. By the time a merger takes place, the target company has usually undertaken standard operational-improvement programs and made some gains. But applying advanced analytics will take asset effectiveness to a new level that’s not achievable with traditional levers.
A global mining firm revealed the power of such efforts when it applied advanced analytics to its work sites. To reduce equipment downtime, it first created a model that assessed the likelihood of machinery failure based on a range of factors, such as the number of operating hours, weather conditions, and average load. The company then created a model that could detect incipient failures based on various sensor inputs, including motor voltage, current, and temperature. Together, the analyses helped the company develop a solution for eliminating early conveyor-belt failures. During an integration, when companies are merging operations, similar analyses could help them develop efficient solutions to common problems. These analytics might be particularly helpful when trying to address issues at the target business, since they may have little understanding of root causes or potential solutions.
The same logic applies to increasing the efficacy of employees or groups. In sales, for instance, merging companies may have significant coverage overlaps. Leaders can eliminate these by gathering data on territories, customers, workloads, and travel patterns. After mapping all coverage overlaps, either within a single company or across both, they should apply advanced analytics to understand the underlying market. For instance, construction players could analyze the number of roads, buildings, and people in specific locations to identify future growth pockets.
The tried-and-true strategies for merging two companies will get the job done, but it’s time to add advanced analytics to the equation. Of course, such efforts may be more difficult during a transaction, when everyone is busy and leaders are occupied with pressing integration tasks—and that means success may require additional staff or resources, such as the addition of data scientists. Companies must also prioritize their advanced-analytics initiatives during integration, since they cannot feasibly pursue every promising opportunity. While advanced analytics may require some up-front investment, they will ultimately increase deal value. They will also reduce stress for everyone involved in integration by deepening insights, increasing transparency, and accelerating timelines—and that gives advanced analytics a value that goes far beyond the bottom line.