Customers have so many ways to engage with companies, it may be surprising that voice conversations remain a popular choice. Recent technologies such as voice data analytics are allowing companies to use these personal interactions as a new driver of insight.
In this episode of McKinsey Talks Operations—the first in a series of two—host Daphne Luchtenberg is joined by Paul Humphrey, CEO and founder of Call Journey, a global thought leader in conversation intelligence and speech analytics, and Eric Buesing, a partner at McKinsey and a leader of McKinsey’s customer care offering. Their conversation has been edited for clarity.
Daphne Luchtenberg: Your company’s future success demands agile, flexible, and resilient operations. I’m your host Daphne Luchtenberg, and you’re listening to McKinsey Talks Operations, a podcast where the world’s C-suite leaders and McKinsey experts cut through the noise and uncover how to create a new operational reality.
Positive customer experiences are driven by high-quality, personalized interactions. And with the rise in bots and all things digital, it could be a fair assumption that businesses would be looking to slim down their call center operations, moving away from human voice interactions. Yet this has not happened, as many organizations are now taking a new look at the value that this personal interface can bring, less as a cost driver and more as an opportunity to provide strategic experience-oriented customer insight. Thanks to advances in technology, we’re now seeing a core analytics use case emerging for voice data analytics. But many businesses still struggle to capture and process the voice conversations they have with their customers in a way that drives real, measurable, bottom-line impact.
This is such a broad topic that we’re going to cover it over two episodes. The first episode will explore the wider trends related to voice interactions in customer care and the technologies that are available. The second episode will explore use cases and best practices for implementing the new analytics tools. I’m joined by Paul Humphrey, CEO and founder of Call Journey, a global thought leader in conversation intelligence and speech analytics, with more than 30 years of experience across multiple industries; and Eric Buesing, a partner at McKinsey and a leader of our Customer Care service line.
Eric and Paul, great to have you here. I’d like to open up our conversation today by exploring why, despite the growth of digital, voice remains a dominant channel.
Subscribe to the McKinsey Talks Operations podcast
Paul Humphrey: I think what’s happening is that people are looking for more of that personal touch; they want to talk with real people. And they want to communicate and converse with people who feel and hear and empathize. Post COVID, we’re looking for more of an emotional connection. In fact, a 2021 Harvard study found that—if I remember it correctly—36 percent of all Americans, including around 60 percent of young adults and about 50 percent of mothers with young children, felt serious loneliness.1 That’s an interesting one from an engagement perspective. In fact, Brand Keys, which is a New York–based brand loyalty and engagement research consultancy, does annual behavior assessments and emotional engagement metrics specifically around customer loyalty etcetera, and they deliver an index to identify the trends.
An index in another study found that customer foundations for brand engagement, product and service purchase, and brand loyalty will almost entirely be emotionally based now.
The index for 2022 goes on to show cross-category decision-making ratios of about 80 percent emotional, 20 percent rational for 2022. The rational aspect for categories and sectors will get filled by customers under what they [the index owners] call primacy of product and service, but brands will need to know the emotional-to-rational ratio for values that drive consumer behavior in those categories. So digital interactions and that utilization clearly drive a better bottom line for company performance, particularly cost to serve. But if you do look deeper at customer journeys, overall engagement, sentiment, emotional engagement with organizations and brands, the smarter organizations we see will know how to engage, who to engage, where and how they engage those people, with ongoing customer interaction intelligence driving that evolution.
Customer foundations for brand engagement, product and service purchase, and brand loyalty will almost entirely be emotionally based now.
That’s all about emotional connection, which is what is lacking in the digital world and why we’re seeing that growth. So emotional connection is the new-age CX [customer experience] key.
Daphne Luchtenberg: That’s a nice way of putting it. And Eric, from your perspective, are you seeing that evenly distributed across all industries? Or are some industries leading the way?
Eric Buesing: It probably does vary by industry. I think there was a perception five or ten years ago that the traditional call center was becoming extinct. To your point, a lot of industries and organizations underestimated how the growth of digital would impact voice and/or maybe how quickly volumes might be replaced. I think instead, the importance of speaking with an informed representative of the organization, as Paul mentioned—that need for human and emotional connection in what we call the moments that really matter to the customer—has actually increased. So yes, the share of digital will probably grow as a percent of total interactions, but total volumes are going to continue to rise. And the complexity of those interactions is also increasing, which impacts handle times.
The question of what’s contributing to this trend—I think there might be a couple. It could be structural. For example, a poor digital interaction is always going to result in a call. Just think about the last time you tried to do something online and you got frustrated. The first thing you want to do is pick up the phone and potentially tell somebody off. So bad digital experiences drive live interactions—that’s the first thing. The other thing to recognize is that more people are transacting digitally and attempting to do more complex things. If more people are doing complex things, then that requires potentially a partial live interaction along with it.
Daphne Luchtenberg: Paul, anything to add there?
Paul Humphrey: No, I was going to mirror what Eric was saying. In fact, there are a couple of really good examples of financial-services organizations that we’ve been working with that are reporting exactly what Eric was mentioning. So if the ratio is staying fairly similar in terms of offline and online interactions, then the more digital, the more growth, the more exposure, the more things that people are exposed to, the more volume they’re going to continue to get by just having that natural growth of interactions. And as Eric was saying, it’s hard to digitize everything; it’s hard to make that easy interaction for customers via digital as simple as possible.
Would you like to learn more about McKinsey’s Operations Practice?
Daphne Luchtenberg: Yes, and that completely changes the purpose and the function of the contact center from delivering a basic service initially, which is what they were intended to do, now to a much more strategic generator of value and potentially also a powerful differentiator, I assume. And Eric, what have you seen organizations doing well? What are some of the steps to becoming a leader in this space?
Eric Buesing: Organizations that aspire for the servicing function of their contact center to really provide an exceptional experience—and by that, I mean they invest in ways to delight customers and really build loyalty versus organizations that view the channel as the last resort, a pure cost center—I think that’s a differentiating factor. And even to push on that, organizations that recognize that the data and intelligence coming out of the contact center are incredibly valuable in other parts of the organization—I’d also characterize that as truly differentiating. For example, in financial services, we’re seeing organizations putting real investment in capacity and rethinking the strategic value of what a contact center is. They’re rethinking their talent, potentially hiring differently. They’re introducing new knowledge systems and technology, all with the aim of increasing the capabilities and the productivity of employees.
Organizations that recognize that the data and intelligence coming out of the contact center are incredibly valuable in other parts of the organization—I’d characterize that as truly differentiating.
Airlines are also a great example of linking customer data from contact centers and live interactions together to create a much more precise and even predictive view on the customer experience. In other words, they don’t need to wait for an NPS [net promoter score] survey to come back to know when a passenger has had a really bad day. They know their flight was delayed, and they missed a connection, and when they called, they waited for 45 minutes, and then their luggage got lost, and they missed a meeting. They can actually verify that through data. And if you can find that out earlier, you can be much more personalized or even faster in how you respond.
Daphne Luchtenberg: I love that. And that all comes back to listening and the listening skills around not just what are your customers saying but also how are they saying it. I know that a lot of us have seen advanced thinking around human speech and developments in neural-network language models, helping to overcome some of the legacy problems related to difficulties in extracting and using call center data. Paul, can you talk a little about what’s the latest and greatest now in terms of natural-language processing technology?
Paul Humphrey: I think, Daphne, it’s best to first define NLP. Some people think it’s not natural-language processing but another version of NLP, neuro-linguistic programming. But natural-language processing, if I give it a basic definition for context, is the art of utilizing computer science and AI to understand and analyze interactions involving computers and humans or human language. So NLP in our world is utilized to process and analyze huge amounts of conversation or conversation transcripts. It looks to understand context, to understand contextual nuances within the language and interactions, which then form actual conversations. NLP is getting toward what our data science folks might call computationally efficient. Historically, delivering NLP solutions has meant a ton of CPU and computing to make it work, which is super expensive and very rigid in what it can do.
Now the new kid on the block arrives, and that new kid is NLU, which is natural-language understanding. Whereas NLP uses algorithms that try to understand language and conversation, NLU looks to understand context. With that smarter technology and computing that we’re talking about now, a combination of NLP and NLU is much quicker and is a better utilization of the good old term “fuzzy logic.” So we’ve gone from NLP, which is kind of “look for these three phrases and see if I get a hit,” to now skipping words that don’t make sense, inferring context, and giving a holistic view of the nuances of a conversation—not just a couple of people talking at each other but a couple of people actually conversing with each other.
For example, if we look at how NLP and NLU are being used at the moment in contact centers, which is a key topic of the discussion, there’s a big demand for NLP and NLU for call summarization. There’s a huge need for that in the world of contact centers. In fact, we did some work recently for a quite large bank, who found that 63 percent of their disposition codes were incorrect. A disposition code is where an agent summarizes what the call was actually about when they’re wrapping up the call. This means that they got the reason for the call—why they [customers] rang or why they contacted the bank—wrong, and they got it wrong 63 percent of the time. That meant that a huge amount of call-reason data being fed to the marketing folks of the bank was wildly inaccurate. And you can imagine the costs of the incorrect data insights to a big bank. That is massive— a huge impact.
This lines up with best practice for organizations to use effective NLP and NLU tools and combine them with machine learning, which is the contact center, and that’s the best place to utilize that type of technology. And it’s not just making the contact center more efficient. In the contact center are massive amounts of rich conversations and interactions that happen with customers every day. In fact, if we look across the US, Australia, and the UK, it’s about 16 billion minutes of contact center conversations and conversations with people every month. So there are huge opportunities being missed if you’re not tapping into that properly.
The other point I think you were talking about earlier, Daphne—the difference between good and great systems when talking about the NLP and NLU—is the utilization and mix of NLP and NLU and combining that with machine learning. I would caution, though, that the view is not just a silo view of having that combination of tools, because you could have great tools, but if you engage a bad carpenter, then the house you want to build won’t be what it should be. So best-of-breed technology and best-of-breed implementation. For example, in our world, 67 percent of speech analytics solutions fail. Some of that’s because of technology capability, and some of that’s because of delivery capability, organizational capacity, and expertise. So you really do need to mix all of those and have the right expertise and thought leadership wrapped around what you’re doing in that world of NLP, NLU, and machine learning.
In the contact center are massive amounts of rich conversations and interactions that happen with customers every day. . . . There are huge opportunities being missed if you’re not tapping into that properly.
Daphne Luchtenberg: Thanks, Paul. So basically, we need to think about NLP, we need to think about NLU, and then there’s the whole element of machine learning that brings this together in a framework that answers the right questions, and that has the folks in the team who know exactly how to analyze and then apply some of the insights that come from that. Eric, you talk to clients every day. Where have you seen clients adopt and embrace this new way of working to enhance what they’re doing?
Eric Buesing: I think we’re seeing new opportunities for impacts being dreamed up and unveiled at our clients constantly. The challenge and the problem that they’re solving are the same, and that’s that the amount of data available or becoming available is unwieldy and overwhelming. Organizations need tools like NLP, which has been around for a while, and NLU, which is really the understanding to help navigate that, not to replace the decision but actually to make a better human decision.
Where we are seeing it, it can be some even foundational basics done well. So sharpening visibility into classic contact center operations, like average handle time: What’s actually happening when agents are speaking? If they’re putting customers on hold, what’s happening during that time? What triggered that? If I was able to resolve the issue in that interaction, which sometimes is referred to as first-contact resolution [FCR], why did that happen—not just the number of FCRs but the actual understanding of what led to resolving the issue the first time?
As Paul mentioned, root cause analysis has been an idea that every organization has been trying to understand for a while, but one of the challenges is customers don’t always call in for one reason. Daphne, you might call in because you’re checking your balance, but by the way, there are three other things you wanted to do while you had that person on the phone, because good luck trying to get them on the phone again. So oftentimes there are multiple things. We call that multiple intent. NLU can uncover pairings. When customers call about one topic, they’re very likely to want to ask about another, which allows a more personalized or predictive interaction in the future.
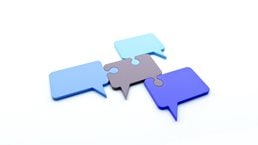
The hidden value of voice conversations: Part 2, Reaping the rewards
That’s the basics, but there are other interesting things that NLU is unlocking on the agent side and from customer cues. So, for example, some organizations might ask themselves, “What are my best people saying? How do they navigate difficult interactions? How do they delight and surprise customers? And how’s that different from an average performer? Can I really get insight into that, so that I can train better, adjust how I upskill or how I retrain or how I coach people?” That’s new and unique.
In areas like compliance, NLP and NLU are helping organizations move from manual sampling, which is literally somebody listening in randomly—you hear that all the time: “This call might be recorded for quality assurance”—moving into AI or machine learning listening to every interaction. In regulated industries like medtech, a sales environment, or in financial services, it’s also providing more assurance that we’re doing right by the customer.
I would be remiss if I said that we’re not looking at employees themselves. A big challenge right now is supporting the employees in an organization better as well. And sometimes we don’t know are people happy or not happy, are they having a good day or a bad day, are there ways that I can create a better experience for them. Sometimes NLP and NLU also give insight into the mindset of the employee and help the organization or help their direct supervisor or managers create better interactions and hopefully a better experience for everybody.
Daphne Luchtenberg: That’s really clear and so exciting in terms of the opportunities. It seems like we’ve only just started to touch on where this can take us.
Now that we’ve explored the technology and the insights it can give us, I’d like to wrap things up for this episode. I hope our audience will join us again for the next episode, where we will take some time to understand the implementation of these tools and how to capture the value. You’ve been listening to McKinsey Talks Operations with me, Daphne Luchtenberg. If you like what you’ve heard, subscribe to our show on Apple Podcasts, Spotify, or wherever you listen.