As retail executives know all too well, most pricing decisions require a trade-off between margin and price perception. To avoid a “race to the bottom”—the self-defeating exercise of trying to beat every competitor’s price on every item—retailers must hone their ability to make smart pricing investments. Indeed, the savviest retailers identify key value categories (KVCs) and key value items (KVIs)—those product categories and SKUs whose prices consumers tend to notice and remember. If a retailer can do this accurately, it can price those specific products competitively while charging higher prices on other items.
Yet, despite the importance of KVC and KVI identification, many retailers still lack a systematic, fact-based process for doing it. Some retailers rely almost entirely on the commercial intuition of experienced category managers. To be fair, a number of retailers do use data to try to isolate KVCs and KVIs: for example, they might benchmark their assortment and prices against those of discounters, on the assumption that price-sensitive consumers use discounters as a baseline for comparison shopping. Some retailers apply a simple heuristic—they use a combination of weighted criteria such as purchase frequency and brand perception to select KVIs.
But in today’s data-rich business environment, retailers can—and certainly should—go beyond these basic techniques. To accurately identify KVCs and KVIs, leading retailers tap into the treasure trove of transaction data, loyalty-card data, and online research available to them. They use sophisticated methodologies that require the ability to analyze billions of transactions and hundreds of gigabytes of data. Harnessing the power of advanced analytics to improve price perception can have significant impact: a margin boost of one to two percentage points, with steady or even increasing sales volume.
Which categories and items affect consumer price perception?
Broadly speaking, products can be classified into two groups: frequently bought items (purchased twice a month or more often) and infrequently bought items. Most grocery items fall into the former classification, but grocery retailers—particularly hypermarkets, which have higher shares of nonfood products—also carry infrequently bought items. By contrast, the assortment of home-improvement retailers consists mainly of infrequently bought items such as power tools and home appliances. Our recommended methodology for identifying KVIs and KVCs differs slightly for each of these two product groups.
Ideally, KVIs will account for 15 to 25 percent of sales in the category. Other products in the assortment are classified as either “foreground” or “background” items (exhibit).
Identifying KVCs and KVIs among frequently purchased products
For frequently bought items, retailers can select KVCs by calculating a normalized score for each category based on three criteria: frequency of purchase (weighted at 40 percent), customer reach (40 percent), and promotional share (20 percent). Then, to identify KVIs, retailers can take four sequential steps, each of which involves the use of big data and advanced analytical models and calculations.
Would you like to learn more about our Retail Practice?
- First, identify SKUs that are a ‘good deal’ or represent good value for money. These SKUs are either cheap relative to the category or have a low per-unit price. A two-liter bottle of soda, for example, might qualify as a good deal whereas a half-liter bottle might not, since the two-liter bottle’s price per liter is much lower. These calculations should be done for every item for every week of data, to correct for any temporary price changes and promotions. (An item on sale might be a good deal that week, but not during other weeks when it is sold at full price.)
- Next, identify customers who buy mostly good-value-for-money SKUs, on the assumption that these are price-sensitive customers who are likely to remember the prices of the products they buy. The analysis can only be done with loyalty-card data. Retailers without loyalty-card data can identify price-sensitive baskets instead of customers, using transaction data.
- Third, assess the relative importance of items purchased by price-sensitive customers. This step requires the retailer to answer two questions about each item: What percentage of price-sensitive customers buy the item? And what percentage of all the customers who buy the item are price-sensitive customers? Those two metrics are then combined and averaged into a price-awareness score.
- Finally, rank the SKUs, according to price-awareness scores, within their categories. The top-ranked SKUs are KVIs.
A methodology for infrequently bought products
For retailers whose assortment consists primarily of infrequently bought items, we recommend a slightly different methodology that combines three sets of analytics, again using big data (see sidebar, “Case example: Nonfood retailer”). Each set of analytics helps the retailer determine which product categories meet the following criteria:
- Frequently researched online and purchased fairly regularly (perhaps once every two to three months). The assumption is that consumers tend to remember the prices of items in such categories. The data on online prepurchase research is typically sourced from web-analytics providers such as Google Analytics, whereas the data on actual purchases is from the retailer’s own transactional data.
- Expensive or purchased fairly regularly. To perform this analysis for a category, the retailer needs to calculate average ticket price and frequency of purchase.
- Often found in price-sensitive baskets. By analyzing transaction or loyalty-card data, retailers can determine which products often appear in baskets alongside other price-sensitive items (that is, those that meet the first two criteria).
Triangulating the three sets of results yields a comprehensive list of KVCs. The retailer can then calculate a price-awareness score—based on frequency of purchase and share of category sales—for each item in the KVCs. The highest-scoring items are the KVIs.
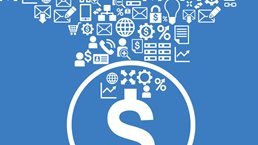
Using big data to make better pricing decisions
Practical advice for implementation
In each case, the results of the analyses should be commercially validated—that is, category managers and the commercial team should review and approve the results. Typically, they would evaluate the KVC list using several lenses. For example, does the category play a strategic role for the retailer? Is the category a traffic driver or one that typically triggers additional purchases? (The purchase of a can of paint, for instance, is likely to trigger purchases of paintbrushes, a ladder, drop cloths, paint thinner, and so on.) Does the category have one or more highly visible brands? We’ve found that the most accurate KVC and KVI lists result from a blend of art and science: category managers’ commercial knowledge and experience, combined with the rigor of big data analytics.
Implementing these methodologies doesn’t require expensive new systems or an army of data analysts. We’ve found that many retailers need just one person with analytical skills to learn how to run the algorithms and codes. That person can then train the commercial team to interpret and use the results.
These methodologies have yielded impact across different types and sizes of retailers in both small and large markets. An Eastern European grocery chain, for instance, had been trying to beat all of its main competitors’ prices on almost every item. Since shifting to a KVC- and KVI-focused pricing strategy, its margins have risen two percentage points. Similarly, a Western European specialty retailer used these methodologies to revamp its pricing architecture and achieved a margin increase of 1.5 percentage points.