Obtaining fair compensation for complex payment products, such as corporate cards, merchant acquisitions, and treasury-management services, has long been a major challenge. This is primarily because these products tend to be intricate, offered in myriad forms, and implemented across diverse markets. Treasury services, for instance, might have 1,000 or more different fees, and prices are often embedded in private contracts not shared within the organization.
Stay current on your favorite topics
Throughout the payment industry, these problems are further complicated by ever-changing payment methods and platforms created by the rapid evolution of payment technologies. And now, expectations of rising interest rates are compounding the situation, increasing uncertainty in product-pricing performance for both the short and long terms.
However, there is good news on the technology front. Just as technological advances are reshaping the payment landscape, they are delivering powerful new analytical capabilities that have the potential to transform the way banks and other payment providers price products and services. In fact, early users are already reporting reduced volume-loss and customer-attrition rates attributable to their use of advanced analytics.
Mining diverse data sets for deeper insight
The complex nature of financial services presents substantial hurdles to those charged with pricing strategically. Merchant acquisition, corporate cards, and treasury services, for example, often include hundreds of products, each with their own distinct fees. Service contracts also differ, and they might begin and end at different times. Moreover, prices tend to be set within the context of the respective client relationship, and transparency within the industry and individual institutions is frequently rare or nonexistent.
In light of such complexities, most payment providers struggle to devise fair and effective price strategies systematically. For instance, a recent McKinsey study of treasury-management services in North America suggests that, over the long term, repricing services leads to value destruction about as frequently as it does to value creation (Exhibit 1). In the study, price increases resulted in revenue declines a year later at more than half of the subject institutions, suggesting the outcome of pricing adjustments is highly unpredictable.
New solutions to cope with pricing complexity are emerging. Developments in computational technology, data engineering, and digitization of general processes can now transform how banks and other payment providers create and implement pricing structures. Rapidly declining costs in high-performance computing and data storage, for example, are enabling them to use larger and more diverse data sets to build more sophisticated analytical pricing models. Unsurprisingly, several industry leaders are already capitalizing on the benefits of these developments.
An especially useful new tool has been SparkBeyond. This application can automate feature engineering by creating a wide range of variable transformations. It is highly efficient in identifying the most effective machine-learning algorithms, such as random forest and XGBoost. The application also enables users to export selected algorithms and features for out-of-sample testing and other modeling needs in an external environment.
Banks that adopted advanced analytics early on have been building massive data sets that integrate customer and prospect details drawn from diverse internal and external data sources. The resulting content-rich data sets are yielding deeper customer and market insights that are unobtainable using traditional data. For instance, government-published econometric data can yield information on economic well-being and thereby better guide a bank’s budgeting process. And adding commercial and benchmark data can help banks more accurately determine their current business share in large corporate relationships.
Would you like to learn more about our Financial Services Practice?
To obtain richer and more actionable insight at a granular level, some institutions are adopting a variety of advanced technological capabilities, including machine learning, deep learning, and artificial intelligence more generally. Artificial intelligence uses algorithms that range from unsupervised (such as clustering and principal-component analysis) to supervised (such as random forest and neural networks) to reinforcement learning.
Some payment leaders are also venturing into interactive digital pricing by either subscribing to third-party services or building their own digital pricing tools. Using new data sources, technologies, and modeling techniques, these early adopters are providing front-end staff with in-depth views of customers and prospects, including such information as their product-acceptance probabilities, price sensitivities, propensities to churn, and lifetime values. These new insights allow managers to identify micromarket segments and thus target pricing more narrowly—down to the individual customer level, when data permits. Closely attuning pricing to customer and prospect needs maximizes price performance while minimizing customer attrition and volume loss (Exhibit 2).
Developing a holistic, multistep approach
Although new tools and capabilities bring opportunities for payment providers to enhance their pricing performance significantly, real success will only come through systematic and comprehensive execution. Achieving maximum effectiveness requires an enterprise-wide pricing transformation.
One way to initiate a pricing transformation is to develop incremental price changes with selected markets or segments using pilot programs that can be quickly learned and iterated before rolled out as a broad pricing program. Once the proof of concept is established, the full program can then be deployed through a three-step approach that includes optional use of early revenue gains to fund subsequent steps:
-
For early transformation success, perform the following initiatives (in addition to other appropriate measures):
- Use advanced-analytics technologies, such as machine learning, to establish pricing benchmarks at a granular level. For example, banks can drill down from traditional segmentation levels (such as geography, industry, and deal size) to postal code or business unit and thus more quickly identify fee leakage at the level of individual customer pricing.
- In parallel with establishing granular benchmarks, develop interactive tools that enable field representatives to recognize pricing opportunities in client portfolios rapidly and simultaneously to leverage other opportunities to expand share of business with the customer.
- Initiate price discussions throughout the organization and redesign the pricing process so it can be implemented in carefully timed waves. A key component of this is building a disciplined exception-management process to strengthen pricing governance and to identify and remedy flaws in current processes and policies.
Initial programs incorporating these three elements can yield revenue lifts of about 15 percent within six to nine months yet incur only minimal client- and volume-attrition rates. And those who implement rigorous service-repricing programs can apply early revenue gains to funding the overall journey.
-
Begin developing new organization-wide pricing skills and capabilities. While this often becomes a longer-term journey, it is one that needs to be initiated and proactively managed from an early stage. This step commonly includes the following actions:
- improving and expanding skill sets throughout the pricing organization
- significantly enhancing current pricing data sets
- building strong pricing-analytics capabilities
- developing enterprise-grade tools to assist in such key areas as new-deal pricing, contract-renewal pricing, and ongoing revenue-portfolio management
Common related investments include acquiring new technology capabilities, such as voice recognition and automation, to reduce manual processing, human-error rates, and technical leakage.
- Equipped with powerful analytical tools, immense data sets, and newfound skills, continually enhance pricing strategies through ongoing monitoring and scaling of new pricing constructs.
Together, these actions are helping many institutions improve the ways they address current and prospective client needs in diverse markets and segments. Pricing tactics, for instance, can be finely tuned to reflect evolving customer and prospect needs by drawing on a variety of pricing approaches, including bundled pricing, subscription pricing for small businesses, and unbundled granular pricing for corporate clients. Consequently, deeper understanding of ever-changing marketplace needs can provide a clear competitive advantage (see sidebar, “How effective is your current pricing strategy?”).
For example, a global payment-network provider developing a new pricing strategy adopted machine learning while applying a similar three-step approach. Drawing on the large amount of transactional data from the past few years, the firm designed a new pricing construct and subsequently simulated its implementation to determine the probable impact on both revenue and attrition. Simulation played a central role in forming the new pricing strategy.
In another case, a global merchant-services company needed to match its established pricing more closely with the current value of its product and service offerings. Using gradient boosting (a machine-learning technique) proved to be highly effective in identifying and realigning numerous mismatched price–value occurrences while accounting for the current competitive environment.
Notably, this framework is expandable to fit a wide range of pricing scenarios. In small-to-medium enterprise lending, for instance, another company devised a value-based account-management strategy; a core component of the effort was developing machine-learning models and a custom user interface that generates client benchmark profits. The customized solution enables relationship managers to simulate various deal scenarios at the full client-relationship level in a test-and-learn environment to ensure continuously monitored and improved results. These changes resulted in value increases of up to 15 percent.
Leaping the hurdles of price transformation
Adopting machine learning and advanced analytics generally gives payment providers significant power to reshape their long-standing pricing strategies. Yet transformation can also present unique challenges.
Advanced analytics presents a variety of sophisticated tools, but their effectiveness depends largely on how the insights are actually derived and subsequently used. For example, traditional approaches to setting pricing targets, such as scoring or ranking customers on price sensitivity, are less actionable than is employing a mathematical model that links offer-acceptance probability to historically accepted offer rates. Pricing models based solely on statistical performance can deliver suboptimal guidance; maximum performance, by contrast, also requires the application of sound business principles and disciplined practices.
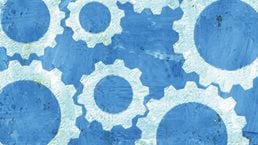
Beyond the buzz: Harnessing machine learning in payments
Aside from aspects of data and analytics modeling, another common obstacle to achieving full effectiveness in the use of advanced analytics is a siloed organizational structure. Organizational silos often lead to departmental misalignments—for instance, among finance, marketing, and sales—when making strategic pricing decisions. In these situations, the best practice is usually to ensure from the outset that all stakeholders have integral roles in planning and implementing the pricing transformation and participate regularly in transformation-planning and progress-review meetings.
To generate positive results, even the best of strategies requires seamless execution. Real or perceived flaws during the rollout of a new pricing tactic can quickly incite rejection among relationship mangers—a problem that successful institutions are overcoming by showcasing success stories and prominently recognizing champions of change within their organizations. Of course, it is also essential to realign performance incentives with new pricing approaches and goals promptly. Engaging relationship managers in codeveloping pricing strategies is a highly effective way to generate positive change attitudes. To instill manager confidence in a new pricing approach, one European bank devised algorithms that can instantly show managers the bank’s pricing structure on comparable deals.
Advanced-analytics technologies are beginning to alter rapidly how businesses operate around the globe. Given the central role of banks and other payment-industry participants, these players are fast becoming subject to those same forces. To remain competitive, payment providers will therefore need to embrace the technologies on a timely basis. Many in the payment industry might be hesitant to change their long-standing approaches to pricing, but those willing to adopt a comprehensive pricing transformation built on deep market insights will clearly be among tomorrow’s industry leaders.