The modern risk-management framework generally relies on the “three lines of defense” scheme, with the businesses, control functions, and audit as the first, second, and third line, respectively. The concept borrows from the language of military strategy, in which intelligence plays a key role. For risk management, intelligence means effective detection: to prevent the bank’s reputation, liquidity, and capital position from being harmed, the lines of defense must detect risks early.
Detection is fundamental in risk management, embedded in its activities and processes. Credit scoring, for example, is a tool for detecting potential borrower-default risk at the application stage, while customer due diligence is designed to identify high-risk customers during the onboarding process, as part of the bank’s know-your-customer (KYC) program. Risk managers are practicing the art of detection when they identify instances of fraud, spot a drifting investment strategy in an asset-management business, monitor their network’s end points to locate cyberintrusions and data theft, or identify potential rogue traders.
Stay current on your favorite topics
Most executives and risk professionals will quickly acknowledge the basic importance of detection. Yet the efficacy of detection—and the levels of “detection risk”—vary widely among risk disciplines and from bank to bank. With poor detection, threats can rise to existential proportions, as some of the world’s largest institutions have learned in recent years. Weak detection capabilities can be costly. Manual controls, for example, are not especially effective and yet they always cost more than automated controls. Poor detection can result in high levels of false positives and the needless diversion of valuable risk resources.
Assessing control effectiveness
Most banks manage operational and compliance risks through detection processes. Accordingly, inherent risks are classified by their likelihood and severity. The effectiveness of the controls is then evaluated, usually on a three- to five-point qualitative scale, with such ratings as “unsatisfactory,” “satisfactory,” and “strong.” In more advanced approaches, the effectiveness of the control is subtracted from the level of the inherent risk, producing a measure of residual risk. For example, an inherently high risk of noncompliance with the Truth in Lending Act can result in a low residual risk if the controls are considered strong (as when customer disclosures and redisclosures are automated).
This type of assessment is frequently deployed as part of the bank’s risk and control self-assessment and independent risk assessment for operational and compliance risks. Used judiciously by trained frontline and risk personnel, the approach can yield valuable insights into the control environment. If applied mechanically, results will be less helpful. Suboptimal outcomes are often caused by the inadequate assessment of control effectiveness, including imprecise testing for accuracy. Without knowing how well their controls are detecting true risks and differentiating them from false positives, banks will be unable to identify gaps in control effectiveness and may have no choice but to add costly layers of controls.
Probability theory
Highly illustrative of the problem of accurate detection are diagnostic tests for diseases, which must account for the potentially high number of false positives resulting from relatively sound tests for rare conditions. Two primary parameters determine the reliability of such tests:
- Accuracy is the probability that a sick person tests positive for a given disease. It reflects the sensitivity of a test in measuring the percentage of people predicted to be sick who are actually sick. A test that is 99 percent accurate means that if it is performed on 1,000,000 sick people, 990,000 will test positive for the disease.
- Specificity is the probability that a healthy person tests negative. A test with 97 percent specificity means that if it is performed on 1,000,000 healthy people, then 970,000 will test negative.
A rare disease might have a frequency of .01 percent, affecting 1 person in 10,000. If the test to detect it is 99 percent accurate and 97 percent specific, then for every 1,000,000 subjects tested, 99 of the 100 who actually have the disease will be correctly diagnosed. At 97 percent specificity, however, the test will also incorrectly diagnose 29,997 of the 999,900 healthy individuals as having the disease. For those who test positive, therefore, the chances that they actually have the disease are less than one-third of 1 percent. That this probability should be so small is counterintuitive and even astonishing. The unmitigated consequences can be devastating: healthy people might believe they have deadly conditions; qualified job applicants might be rejected for assumed drug use. For banks, the consequences of poor risk detection can be seriously damaging as well.
False positives and risk management
Banking executives and risk practitioners seeking to detect and prevent low-frequency events will recognize the problem of false positives. In anti–money laundering (AML), for example, a monitoring system is usually deployed that produces alerts on atypical transactions. These are referred to a financial investigations unit (FIU) comprising experts who often have a background in law enforcement. Based on certain criteria, they attempt to identify likely instances of fraud from among the alerts and accordingly file suspicious-activity reports (SARs) with the appropriate authorities.
Should a transaction-monitoring control detect suspicious activity with 95 percent accuracy and specificity, 5 percent of the activity it determines to be legitimate or suspicious will not actually conform to the established criteria. If 0.1 percent of transactions truly do meet the criteria for suspicious activity, then this particular control could produce a false-positive rate of over 98 percent. Fewer than 2 percent of alerts will correspond to activity that upon further examination will qualify as suspicious. The FIU investigators will have to spend a lot of time investigating cases that do not qualify as suspicious, leading to a low conversion of alerts into SARs.
In practice, controls may be even less specific. If the control in the example above were 75 percent specific, more than 99.5 percent of transaction alerts would be false positives. Increasing the accuracy of the control to 99.9 percent will not reduce the false-positive rate significantly (the false-positive rate would remain above 99.5 percent).
The implications of inadequate control specificity
Improving control performance demands increased focus on specificity. A control that detects only 50 percent of positives, for example, but is highly specific—incorrectly signaling a positive for only 0.1 percent of negatives—would have a false-positive rate of 67 percent. If the specificity were improved from 0.1 to 0.01 percent, the false-positive rate would drop to 17 percent.
Good detection is not simply about reducing false positives. Controls must also be highly accurate, detecting a large percentage of positives. But equal attention must be paid to control specificity for the control environment to perform optimally. In AML, the objective is the accurate identification of transaction patterns associated with illegitimate activity. This implies the ability to distinguish such patterns from those originating with legitimate clients. To reach this objective, control assessments are vitally important. Unfortunately, many control assessments are merely qualitative or unable to differentiate between control accuracy and specificity.
Would you like to learn more about our Risk Practice?
Inadequately specific controls cause valuable resources to be diverted from actual risks. Fraud investigators are taken away from vital work, such as identifying connections between cases—“connecting the dots”—to detect networks of criminal activity. The problem of false positives is more than a matter of cost control. While regulators at times take a favorable view of increased spending on controls, the addition of manual controls is not always the best way to resolve detection issues. Banks should be focusing on improving the effectiveness of the control environment in critical risk areas—an approach that can also lower spending on manual controls.
Making progress in key areas
Leading banks are making progress in risk detection in several areas.
Anti–money laundering
AML activities are triggered by alerts generated by rules-based binary criteria. The alerts, investigated manually, usually have very high false-positive rates. Banks have discovered that tighter segmentation of alerts, the use of KYC data, and the admission of additional variables can improve the specificity of AML controls. In one example, the false-positive rate was cut in half with the use of additional data on internal transactions (see sidebar, “Deploying AML resources where they are most needed”).Compliance testing and monitoring
Banks frequently have difficulty determining whether compliance controls are truly effective at reducing noncompliant outcomes. One reason is that controls are often designed to ensure that internal procedures are followed, rather than to detect and prevent noncompliant outcomes. Having recognized the issue, some institutions are exploring ways to enhance the detection of compliance defects with system-generated process data—such as time stamps, queue status, work-flow history, and transaction attributes. In mortgage servicing, for example, institutions are increasingly using system data to achieve greater accuracy and specificity in their compliance controls.
Cybersecurity and fraud
Detection tools used to flag potential cyber-intrusions and unusual network and system activity generate alerts, which, like their AML equivalents, require further investigation. Many companies invest heavily in detection capabilities without giving sufficient consideration to how accurate and specific these alerts are in practice. The return on security investments should be the ability to separate “noise” (false alarms) from “signal” (real threats). One company is now pooling its security data into a data lake and exploring all potential correlations among the incidents and events that trigger alerts. The result is a material improvement in the signal-to-noise ratio and faster response times by company security analysts. This approach to reducing false positives and redirecting resources to real threats can be enhanced by adding external data sources, such as threat feeds or “dark web” scanning.
Consumer-credit underwriting
Most consumer-credit decision algorithms in the United States and Canada use a combination of credit scores and binary exclusion criteria. The credit scores correspond to the expected probability of nonpayment as derived from credit-bureau data, while the exclusion criteria might include such data points as “no bankruptcy in the last five years.” The binary data are usually effective in filtering out high-risk borrowers but can produce a large number of false positives—credit applicants will be rejected who would have actually performed well. One credit-card executive referred to his firm’s process as a “meat cleaver” that tended “to chop off” many potentially good customers. In response, the most sophisticated credit-card issuers are reducing the false-positive rate by improving the specificity of their proprietary credit scores and the binary exclusion criteria. This approach allows them to book profitable accounts that were deemed too risky by less advanced lending criteria.
Credit collections and portfolio management
The effectiveness of credit-collection strategies depends on the ability to identify high value-at-risk borrowers—those with significant credit balances that are also likely to be charged off. Often the best approach to these borrowers is to offer settlements and partial-payment programs early in the collections process. Obviously, such an approach cannot be deployed indiscriminately or it will result in unacceptable losses. Highly specific and reliable early detection of these borrowers is the goal, but it has been difficult to achieve in practice. Banks are beginning to explore advanced analytical techniques, such as random-forest algorithms, which can produce higher specificity than traditional logistic-regression models. To detect borrowers with high value at risk (VAR), one institution replaced a traditional VAR model with a machine-learning model and improved detection performance by 60 percent. In combination with contact-and-offer strategy models, the new analytics-based approach has significantly increased efficiency of the agents.
Credit portfolio management
In credit portfolio management, the early identification of borrowers at risk of imminent default is highly advantageous for retail and commercial lenders. This capability permits banks to respond by reducing credit lines, securing additional collateral, or modifying loans. Early-warning signals must be highly specific or unacceptable levels of false positives would result, outweighing the benefits of the approach. The costs of false positives include loss of revenues due to lower balances and customer attrition; in the long run, the institution’s reputation can suffer. Lenders are already exploring ways of predicting defaults with greater accuracy, such as with machine learning applied to market data (such as credit-default-swap spreads) as well as unstructured data (such as sentiment analysis).
Five actions can improve risk detection significantly
Accurate detection is an essential capability for robust risk management. Institutions cannot improve detection effectiveness and efficiency overnight, but experience shows that meaningful progress can be achieved in 9 to 12 months. To be successful, near-term transformations of the control environment should include the following five actions.
1. Reviewing the control framework
This review improves the control environment by identifying gaps and inefficiencies and taking remedial action. Unnecessary or ineffective controls are culled, as are some manual controls or controls based on procedural adherence. The required accuracy and specificity of controls in high-risk areas, such as AML, fraud, and cybersecurity, should be determined by the frequency of the underlying risks. Throughout the review, testing based on residual risk or outcomes should be promoted over control testing, especially where results have been statistically unreliable.
Manual controls may be symptomatic of high false-positive rates in detection processes and therefore warrant close analysis. The control review should focus on replacing or augmenting manual controls with system-driven detection algorithms. Risk and control assessments, in particular those based on subjective or abstract criteria, may simply be unreliable, creating a false sense of security. Such programs should be evaluated for their efficacy relative to the nature and frequency of the risks. Assessments that do not enhance resilience of the bank will have to be dealt with critically. Resources freed by improvements (for example, the elimination of unnecessary manual reviews of false positives) should be deployed to critical areas.
The control review must be executed with good judgment to ensure that it increases control effectiveness. It cannot be approached as a template-driven, mechanical exercise: the risks are too high and regulators will be watching closely.
2. Changing the detection paradigm
Transformative change requires a fundamental shift in strategy. Banks should move beyond the detection of individual suspicious activities to detecting clusters of such activities. In AML and fraud, this means identifying the bad actors as opposed to focusing predominantly on potentially suspicious individual transactions. To do this effectively, banks will need to acquire more data sets. This will allow them to filter out more noise—the false positives—and to create risk scores that achieve better predictive power than binary detection criteria. Investigators of security threats may flag the purchase of fertilizer or the renting of a truck as warning signs of a potential terror attack, but they must also account for the fact that the vast majority of these transactions are completely legitimate. In our experience, traditional, rules-based detection methods in AML reach their potential for reducing false positives at around 90 percent. To go further, banks and regulators alike must reframe the problem statement and apply advanced-analytics solutions to look for networks of events.
3. Applying advanced analytics and automation
Improving detection by replacing ineffective and expensive controls will require that banks develop significant capabilities in advanced analytics and automation. Where suitable, machine learning should also be integrated into existing analytics capabilities. Institutions can even set up a dedicated machine-learning factory as long as they guard against it becoming a “hammer looking for nails.” Analytics efforts must follow practical necessity and not create problems to solve. The “decision science” model used by credit-card issuers to support credit, marketing, and collections strategies, for example, is a technique-neutral approach. The decision trees, logistic regression, and other modeling techniques it may employ are selected based on their applicability to a specific detection problem.
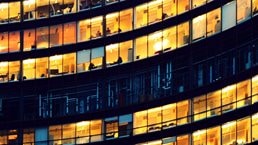
Sustainable compliance: Seven steps toward effectiveness and efficiency
One misconception about advanced-analytics techniques is that they require vast quantities of high-quality data. While some techniques (such as neural nets) do require a lot of data, many do not. Furthermore, many very productive advanced-analytics methods thrive on unstructured and imperfect data. They can even help make the data accessible for more traditional techniques. Banks must always seek to improve data quality, but perfect data is a quixotic goal—unattainable in the near term and never cost-effective. With advanced analytics, data-quality issues are a fact of life, and banks must deploy measures to account for them.
Problems of model validation give pause to even the most committed proponents of advanced analytics. Current model risk management approaches have been honed on relatively well-understood regression and decision-tree techniques. For more complex machine-learning models, banks are still developing standards. Spurred by difficulties with validation revenue models for regulatory stress testing, some banks are starting to define universal principles of forecasting and modeling techniques that could be applied to nontraditional and advanced methods. This could open the way to a more flexible—but still policy-driven—model-validation approach.
4. Developing a portfolio of use cases and matching processes
Banks need to develop and manage a portfolio of use cases. The program should be designed to encourage expert creativity while ensuring a balanced portfolio. The use cases should address a diverse set of risks, with a range of probabilities and potential impact. Good governance is important, as the actual impact of the use cases will need to be validated against initial assumptions; furthermore, the feasibility of implementation must be assessed in light of regulatory requirements, system implications, and operational impact. A significant portion of the use cases developed for compliance and operational risk should contribute to simplifying and strengthening the control environment. The effort will generate demand for advanced analytics and automation in this area. Examples of potential use cases include monitoring employee conduct, contract compliance, and payment-fraud detection.
5. Engaging with the regulator
To improve detection, rationalize controls, and strengthen risk management, appropriate regulatory engagement is required. The conditions for such engagement may not yet be in place for banks addressing enforcement actions or major regulatory enhancements. Nevertheless, all regulatory issues need not be resolved before banks begin this program. Some banks are deploying advanced techniques in place of manual solutions as part of their major regulatory programs, including resolution planning and Comprehensive Capital Analysis and Review.
Banks might be surprised at regulator reaction to their plans to rationalize controls. Efforts to improve detection effectiveness in KYC and AML, for example, may be welcomed, given recent high-profile detection failures. In the approach we have been discussing, efficiency gains and greater effectiveness are closely linked. The business case for rationalizing expensive manual controls is based on better detection and risk management. Getting comfortable with efficiency gains as part of the business case for better detection is a requirement for success.
Nowhere is regulatory dialogue more important than in model risk management. Input from the regulator is required to meet the challenges posed in the validation of sophisticated models. Banks may therefore want to focus first on machine-learning models used to detect fraud and money-laundering activities before tackling models affecting consumer access to credit. With such checks in place as model performance controls and parallel runs, the models for detecting fraud and money laundering may be cleared for testing and deployment more readily.
Regulatory and competitive pressures as well as rising business costs are driving banks to improve the effectiveness of their risk controls. To reduce unmanageable levels of false positives—and all the added work they entail—leading banks are developing significant advanced-analytics capabilities and automating costly manual steps. Complex risk-detection problems, such as those involved in model validation, are inspiring innovative approaches to improve control standards and resource allocation. As the early movers are discovering, these investments lower operating costs while returning business, compliance, and reputational dividends. To make further progress, banks and regulators should consider the limitations of existing detection approaches and be open to applying methods that enhance results.