Are we entering a world of smart machines that can warn their operators before they break down? Advanced predictive maintenance (PdM), enabled by extensive sensor integration and machine-learning techniques, is one of the most widely-heralded benefits of the fourth industrial revolution. The idea is certainly a compelling one, and it is encouraging companies in asset-intensive sectors to pursue investments in digital maintenance and reliability.
Stay current on your favorite topics
In our view, however, treating PdM as panacea for maintenance and reliability challenges may prove to be short-sighted. In part, that is because today's advanced predictive techniques can only be practically applied to a subset of use cases. But it is also because an over-emphasis on one approach means companies won’t position themselves to capture all the potential benefits of a fully digitized maintenance and reliability function—one that’s focused on increased uptime and improved maintenance efficiency.
And those benefits are significant. Based on our observations of digital maintenance and reliability transformations in heavy industries, we see the potential for companies to increase asset availability by 5 to 15 percent, and reduce maintenance costs by 18 to 25 percent.
The opportunities and challenges of PdM
It’s easy to see why advanced predictive maintenance has been seen as a killer app for Industry 4.0. The approach combines many of the technologies that underpin the new wave of industrial digitization, such as networked sensors, big data, advanced analytics, and machine learning. It is a powerful technique that, by identifying complex patterns over hundreds or thousands of variables in ways that traditional analysis cannot, enables operators to develop a deeper, data-driven understanding of why failures occur. Most seductively, it promises a very tangible benefit: machines that don’t break down.
But in practice, economically viable, real-world uses for these advanced PdM techniques are less than universal. Where a machine is prone to a narrow range of well-understood failure modes, it is often possible to address a potential problem in a simpler way, for example by monitoring the temperature or vibration of a component against a set threshold, or by consistently and rigorously applying data-driven reliability analysis techniques to address the root causes of failure modes. Conversely, where a machine can suffer hundreds or thousands of different kinds of failures (some of them very rare), it can be impractical to create sufficient models of high-enough quality to adequately predict them all.
When factoring the effort and expertise required to develop accurate machine-learning models, model-based predictive maintenance becomes a breakthrough way to solve selected high-value problems, rather than the whole universe of maintenance opportunities. The approach has the most potential where there are well-documented failure modes with high associated downtime impact, for example in a critical machine on a larger production line. It also works well when it can be applied at scale to a large fleet of identical assets where there is sufficient reliability history to spread the development and management costs, as in offshore wind farms or fleets of locomotives. Thus, equipment manufacturers are strategically positioned to drive predictive-model development and deployment at scale for their end users—but these efforts have yet to materialize widely.
Capturing the digital dividend
Does the relatively limited scope PdM has achieved mean that maintenance and reliability are somehow exempt from the digital imperative? Absolutely not. In fact, we propose that companies press well beyond one particular type of digital tool and think about how digital and advanced analytical techniques can transform their entire maintenance and reliability system. This means looking end to end for opportunities to make better use of data, and apply user-centric design principles to digitize processes. Sustainable impact will require a combination of new digital tools, changes in asset strategy, and improved reliability practices.
Would you like to learn more about our Operations Practice?
An integrated approach to digital reliability and maintenance
Reliability and maintenance activity has two basic parts: a program element, which encompasses asset strategies and maintenance plans, and an execution element, which encompasses identifying, prioritizing, scheduling, and performing work. Digital reliability and maintenance (DRM) encompasses both those elements, and underpins them with a set of enablers—the infrastructure, processes, and tools companies need to manage their assets, data, and people, in improving asset reliability and maintenance performance (exhibit).
Digital reliability enablers
We start from the foundation up, with enablers. Most importantly, digital processes are fueled by data. That’s why establishing a robust data backbone is a fundamental enabler for digital reliability and maintenance. Most organizations already have systems in place to record maintenance- and reliability-related data, but the effectiveness of such systems can be undermined by poor housekeeping. The same assets or issues may be described in different ways in different systems, for example, making integration difficult. Companies may use free-text fields to record issues or maintenance actions, making automated search or data analysis harder. Or critical data may be inaccessible, locked away in spreadsheets or on paper notes.
Fixing these challenges often depends not on investment in new technology but on the adoption of more rigorous standards for asset identification and data recording. Artificial-intelligence techniques, such as natural-language processing, can help organizations transform poorly organized historical data into a form more suitable for automated analysis.
Similarly, the plummeting cost of data storage and network bandwidth mean that it is now easier and cheaper than ever to collect data streams from machine-control systems and external sensors. This data, which may be inaccessible or even discarded today, is useful for condition-based monitoring, diagnostics, and failure-mode analysis, either using conventional approaches or the application of advanced analytics and machine learning.
Once they have their data in place, companies need a means to access it. For most organizations, this requires a new step. A consolidated data-services layer, or “data lake,” collects data from multiple systems and sources, creating a single source of truth and bridging the information gap between systems to provide a complete picture of an asset’s health. This critical component of the data architecture has multiple uses: it provides the basis for digital performance management, descriptive analytics, and dashboards, while also serving as a unified layer for new maintenance and reliability applications and supplying the data required for advanced-analytics models.
The next essential enablers for DRM are digital tools for reliability-engineering analysis. Root-cause problem solving, using approaches such as fault-tree analysis as well as cause-and-effect or failure-modes-and-effects analysis (FMEA), is a fundamental part of any organization’s maintenance and reliability strategy. Today, however, these activities are often conducted manually, and their outcomes are rarely recorded in a centralized manner. Integrating reliability-engineering tools into an organization’s DRM architecture ensures that analyses are conducted in a consistent, structured way, accelerates and simplifies access to input data, and captures analytical outcomes for future use.
Creating a digital platform capable of handling the full range of tools and data sources used in digital reliability and maintenance can be challenging, but getting this right early in the DRM program will deliver enduring benefits. One oil and gas company had already started to build maintenance solutions onto an existing platform. As leaders mapped out their digital-maintenance ambitions, however, they realized that the system didn’t have the technical capabilities they required. Since seamless interconnection between tools was central to its long-term maintenance vision, the company chose to integrate all its maintenance solutions into a brand-new platform, even though this required rework in the short term. The result is a DRM function that can expand with the organization’s needs and digital capabilities, rather than providing only a temporary boost that quickly falls behind as competition (and technical capabilities) advance.
Significantly, the enablers discussed so far focus on the application of digital technologies to accelerate, streamline, and improve existing reliability-engineering practices. Digitization is also providing reliability-engineering teams with a plethora of new tools and approaches. As we have already described, the application of machine-learning techniques to monitor asset condition has already received considerable attention, even though their cost and complexity may ultimately limit their application.
Not all condition-monitoring techniques require elaborate algorithms or complex models, however. Data-driven condition-monitoring approaches use simple queries that are run periodically or in real time against time-series data generated by machines and external sensors. If threshold conditions are passed, these systems can trigger investigative or corrective action in the digital-reliability-engineering workflow, or directly to maintenance execution.
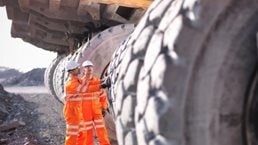
Smarter choices: Raise asset availability and reduce operating costs
Digital performance management
The enabling technologies described above establish DRM’s foundation, but don’t actually improve asset reliability or maintenance effectiveness. Those improvements come from the way an organization uses its digital data to optimize maintenance activities: adapting schedules, streamlining plans, and prioritizing resource allocation.
A digital performance-management system is central to the operation of an effective DRM program. This involves the use of descriptive analytics and data visualizations to provide a real-time view of asset health and reliability performance. Digital performance management automates the generation and presentation of the key metrics and qualitative information that companies use in their reliability programs, such as overall equipment effectiveness (OEE) data or loss reasons. This kind of automation is a surprisingly powerful improvement lever, freeing maintenance staff from the time-consuming and error-prone process of data collection and analysis. And it supports rapid trend identification, fact-based decision-making, and timely intervention, as well as changes in equipment investment, processes, and policies.
Sometimes, companies already have much of the digital infrastructure they need to manage maintenance performance. One mining company, for example, was preparing to source a new system to track mobile-equipment maintenance. As it outlined the requirements for the new system, it realized that the required functionality existed within its current computerized maintenance-management system. The relevant modules had even been piloted within the organization, but never scaled up.
The cycle time and effectiveness of reliability-engineering activities are often hampered by missing information or poor alignment between operations, reliability, and maintenance teams. Digital reliability-engineering workflow systems help address those gaps by tracking the full lifecycle of each unit of work conducted by the reliability-engineering function. At a minimum, these systems capture the details of the event or events that trigger an investigation by the reliability-engineering team, the actions taken in response, and the outcome of those actions.
Digital asset strategies
New digital tools can also help to accelerate and standardize the cost-benefit analyses and decision-making that underpin maintenance and reliability activities. Digital asset-management tools, for example, help reliability teams plan and manage repair or replacement choices over the lifecycles of individual assets or entire fleets. Similarly, new digital tools can support reliability-centered maintenance, helping teams choose the right maintenance strategy (such as run-to-fail, planned preventative maintenance, or condition-based maintenance) for each asset.
Digital work management
New digital tools are also transforming the way companies plan and manage the execution of maintenance and reliability activities. Digital work management includes process digitization and data-driven analytics to improve the effectiveness and efficiency of maintenance work. Example applications include automated scheduling algorithms, digitized planning environments, and tablets or wearable devices for field data entry and retrieval.
Most industrial players are already on a DRM journey, whether they are aware of it or not. They are already recording their work orders in an enterprise-resource-planning or asset-management system, and many of their assets are already generating and collecting data, even if this data is widely dispersed and little-used.
Right now, however, this “digitization by default” approach isn’t delivering the full potential impact it could. When we surveyed a group of maintenance managers earlier this year, only 50 percent said their current information and operational (IT/OT) architecture adequately supports their maintenance and reliability processes and fewer than 20 percent felt that their maintainers have a positive user experience.
The critical step for most organizations is the shift to a proactive, comprehensive and well-thought-out approach to their digital maintenance and reliability strategy. This involves a detailed assessment of current maintenance and reliability practices to identify where visibility from improved data capture, insights from advanced analytics, and increased control from new digital maintenance execution systems can create impact. The key is to take a broad end-to-end view of potential applications and to think about how new tools, technologies and approaches can be integrated and combined.
Like any significant change effort, moving to this new digital reliability and maintenance world will require companies to be bold in their aspiration, structured in their transformation approach, and long-term in their vision.