As the technologies of the Fourth Industrial Revolution continue to evolve, the role of data has become indisputable. In an effort to extract even more value from data, organizations are increasingly linking and connecting diverse data sets, a practice that stands as one of the primary factors shaping today’s global economy. From 2017 to 2019, the percentage of companies forming data-related partnerships rose from 21 percent to 40 percent. A growing share of business competitors are also deciding to connect their data—rising from 7 percent to 17 percent. Overall, McKinsey estimates that connecting data across institutional and geographic boundaries could create roughly $3 trillion annually in economic value by 2020.
Stay current on your favorite topics
The application of commercial data-sharing practices to confront humanitarian and development challenges (in areas such as poverty, public health, environment, and sustainable agriculture) is also gaining momentum. Data collaboratives that include leveraging private companies, research institutions, and government agencies to help solve public problems offer great promise. Yet, while there have been some impressive results from public-private partnerships to date, there are still few global success stories where large-scale, commercially controlled personal data is used for the common good. An entangled set of legal, technical, social, ethical, and commercial risks have created an environment where the incentives for innovation have stalled. And lack of trust among individuals and institutions creates even more uncertainty.
Given the risks and challenges, it can be easy for leaders to forgo pursuing public-private partnerships. However, the cost of inaction can be immense as well. According to the director of UN Global Pulse, the lack of data innovation is resulting in a failure to protect the public from preventable harm.1
So how can leaders best balance the imperative to innovate for the common good with the need to protect against emerging risks?
Based on the output of a series of global workshops and summits with business, government, academic, and civil society leaders and experts and practitioners, the World Economic Forum, in collaboration with McKinsey & Company, has outlined a holistic governance framework designed to help leaders strengthen trust, balance competing interests, and deliver impact.
This article, extracted from the full report—Data collaboration for the common good: Enabling trust and innovation through public-private partnerships—summarizes key findings. From a stakeholder perspective, this report focuses primarily on the commercial entities that function as the data holders from the supply side (particularly from the mobile-network-operator, financial-services, healthcare, and social-media sectors) and, from the demand side, the needs of large-scale international organizations and the United Nations System.
Strengthening trust to achieve impact
While growing evidence shows the value of public-private data collaboration, the challenges and risks remain daunting (Exhibit 1). Interconnected issues related to security, privacy, commercial risk, cross-border data flows, reputational concerns, due process, and regulatory uncertainty all serve to create an environment that operates at a slow and deliberate pace. Underlying these concerns is a profound and growing lack of trust among individuals, institutions, and governments.
Strengthening trust will require a number of coordinated actions related to the economics, operations, and governance of public-private data collaborations. Without this cooperation, these partnerships will face difficulty balancing tensions between the need to protect data and the opportunities to innovate in its use.
Aligning on shared taxonomies can serve as an initial step for diverse stakeholder communities to pursue common goals in concrete ways. Accordingly, the World Economic Forum has arrived at six dimensions of trust:
- Security involves having the people, processes, and tools required to ensure that the confidentiality, integrity, and availability of data will be upheld and protected throughout the life cycle against malicious attacks, unintended accidents, and naturally occurring “acts of God.”
- Accountability involves processes to ensure that network stakeholders are held responsible for upholding accepted standards and agreements so that relationships remain reliable and predictable.
- Transparency involves giving stakeholders meaningful ways to understand how relationships are structured and how data is being used. It also requires organizations have the capacity and oversight to ensure all outcomes from a data collaboration are accurate and that biases (intentional or unintentional) are not systemic.
- Auditability involves the creation of feedback loops for externally checking, verifying, and monitoring data flows across an array of stakeholders and jurisdictions.
- Equity involves ensuring that value is apportioned fairly and that outcomes are unbiased.
- Ethics guide stakeholders through potentially ambiguous, uncertain, or highly context-dependent decisions. For data collaborations, one core ethical principle should involve protecting the rights, aspirations, and intentions of vulnerable populations.
Would you like to learn more about McKinsey Analytics?
Establishing an evidence-based framework
Through a series of global workshops, expert interviews, and use-case analyses, the World Economic Forum has established an evidence-based framework designed to identify specific areas for strengthening trust. The approach’s emphasis on iterative alignment, consistent communication, and comprehensive governance is intended to help stakeholders understand and respond in pragmatic and practical ways. This holistic approach identifies five areas—stakeholder alignment, responsible data governance, insight generation and validation, insight adoption, and economic sustainability and scalability—for strengthening trust and catalyzing action (Exhibit 2).
1. Achieving stakeholder alignment at the outset of a partnership. The first step toward an effective data collaboration is for all relevant stakeholders—including government, industry, civil society, nongovernmental organizations, and individual data producers—to align on a shared value statement and to gain assurances that there is a long-term commitment by all parties. There are four areas that can help strengthen trust during the stakeholder-alignment phase:
- Conduct rigorous due diligence to ensure commitment and resource availability. Particular attention should be paid to ensure that the input of frontline workers is incorporated into the decision-making processes of senior leaders. Actively engaging with vulnerable populations is also critically important, as the needs, risks, and aspirations of those at risk of becoming “digital invisibles” are often overlooked. Lastly, establishing an open and flexible process for defining and balancing limits on scarce resources (available time and funding) is necessary to minimize implementation risks.
- Identify and balance competing stakeholder incentives. Transparency of stakeholders’ questions and concerns about their counterparts can ensure mission alignment from the start. With a shared-value proposition, stakeholders are better encouraged to deliver on the collaboration’s purpose.
- Commit to a clear use case with measurable outcomes. The collaboration must have a clear goal with defined outcome measurements to guide participants. Stakeholders should have some flexibility in working toward their objectives and should be free to pursue new directions if results indicate another path is more promising.
- Establish an operational plan with auditing and ethical oversight processes. Translating stakeholders’ incentives, requirements, objectives, and commitments into an operational plan will establish execution requirements and demonstrate clear accountability on roles, responsibilities, and duties of care, while providing a contingency plan to manage ethical or other operational uncertainties that may arise.
2. Establishing responsible data governance. This phase aims to establish an approach that is legal, fair, and just in the use of data.2 The scope of governance concerns extends beyond privacy and data protection to include a wider set of issues related to the agency of individuals and safeguarding against group harms in the use of demographically identifiable information (DII).3 There are four responses that can help strengthen trust during the responsible-data-governance phase:
- Implement information-sharing protocols and guidelines to balance security, privacy, and ethical considerations in the use of data. This includes creating an ecosystem map with data-flow diagrams to demonstrate what data processes are occurring, when, and by which individuals; articulating the value of required data and how it relates to the specified purpose of the collaborative effort; and establishing a comprehensive data-impact assessment to identify severe and likely data risks and to balance competing interests and potential impacts (negative and positive) across the broader ecosystem.
- Build a secure, resilient, and fit-for-purpose governance structure and technical infrastructure that meets the use-case demands. Requirements can range from addressing concerns about data security, data-storage location, access rights, and regulatory restrictions. A thorough understanding of the requirements, strengths, and trade-offs of various technical design archetypes will equip stakeholders to make the best implementation decisions.
- Establish external review boards to address legal ambiguities and ethical uncertainties. An external oversight body can provide guidance on ensuring fairness, local engagement, and transparency. It can also assist with decisions about the use of data and the intended outcomes. These review boards can be a helpful counterweight to the internal power dynamics that shape how decisions are made.4
- Turn principles into practice. Data collaborations need clear standard operating processes and pragmatic frameworks that enforce rigorous security measures and protect data subjects. Pragmatic frameworks that guide decision makers through the complex reputational, social, legal, and ethical trade-offs within a data collaborative are also required. These concrete processes and frameworks should be communicated to all stakeholders and at all levels of an organization. Contracts and agreements are also essential tools for ensuring that stakeholders are held accountable to governing principles and that they understand the frontline risks, realities, expectations, roles, penalties, duties, and responsibilities as they embark on data-collaboration efforts.
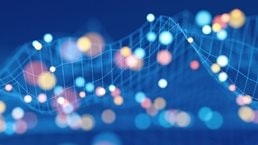
Applying artificial intelligence for social good
3. Delivering insights that are accurate, unbiased, and explainable. As it relates to trust, the concerns at this phase are multilayered: the data inputs should be legitimately collected, complete, and accurate; the data processing should be reliable, replicable, and interpretable; and the derived and packaged outputs should be valid, fair, and interoperable within a defined context. There are four responses that can help strengthen trust during the insight-generation and -validation phase:
- Verify the provenance, completeness, and accuracy of data inputs. Stakeholders can introduce cross-functional metadata standards with common attributes to understand the origins, completeness, and accuracy of data sets. This will help aid in minimizing bias and model-performance testing.
- Ensure the explainability of algorithms and derived data insights. Investing in interpretability measures on the front end—such as designing a model with fewer features or visually mapping critical data inputs—will make outputs easier to understand for data engineers and end users alike.5
- Establish feedback loops to check the reliability and legitimacy of outputs. These mechanisms will encourage input from local experts who are closer to the data collaboration’s application, while encouraging the collective and iterative refinement of insights.
- Implement responsible governance processes for the distribution of data products. Stakeholders should ask themselves the following questions: What decisions and actions will the packaged data products and services support? How should they be published or presented? How often should they be updated to protect against model deterioration?6
4. Providing decision makers with the tools, processes, and support to act on new insights. Barriers to insight adoption most often arise due to challenges implementing data products and/or a lack of alignment around the monitoring and evaluation of the success of new decision-making processes. There are three areas of focus to help strengthen trust during the insight-adoption phase:
- Identify top-down and bottom-up champions for change. Word of mouth and peer-to-peer exchanges on the benefits of using the new tool can drive adoption from the bottom up. Linking job-performance evaluations to how often individuals have used a new tool to make decisions can serve as an effective top-down measure.
- Establish two-way product-support communication channels. It will be essential to explain and continuously improve new products. Organizations should also have robust and responsive product-support channels to answer specific questions and receive feedback from the field.
- Deliver sustained training to build capabilities, including investing in time and technologies to train users in how the tool works, its limits, and how it fits into existing decision-making processes. While initial product support may come from outside the adopting organization, a transition plan needs to be in place for how product support and training will be managed over time.
5. Ensuring long-term economic sustainability. Given that many existing collaboratives were initially underwritten by donors (or started as “data-philanthropy” donations from the private sector), the question of sustainable economics has historically been less of a priority for data collaboratives. However, as early-stage data collaboratives mature, the question of how to establish sustainable economics has promoted the need for a more holistic framing of the challenge. There are three areas of focus to help strengthen trust during the economic-sustainability and -scalability phase:
- Evaluate a variety of funding mechanisms matched with the project objectives. Stakeholders need to establish a sustainability strategy as early as possible and should explore innovative pricing and platform economics within controlled marketplaces.
- Identify opportunities to expand and scale across industries and geographies. To minimize tensions surrounding economic sustainability and scalability, stakeholders require a thorough and clear understanding of the collaboration’s intended path to scale.
- Encourage commercial opportunities that draw on the notion of data trusts and the engagement of community co-ops, credit unions, and trade associations. Explore the notion of data trusts as a means both to provide ethical, architectural, and governance support for trustworthy data processing and to reapportion the value of data products and services back to individuals and local data producers.
Addressing society’s most complex global challenges, making the best decisions during times of crisis, and monitoring progress on UN Sustainability Development Goals all require access to the most granular, timely, and complete sets of data available. That won’t be possible without robust public-private data collaboration. The framework discussed in the full report provides leaders with a new approach on how to encourage positive change by both strengthening relational trust and unlocking new forms of technical innovation. It also outlines concrete partnership opportunities that came out of the World Economic Forum’s Global Dialogue on Public-Private Data Collaboration.
The report originally appeared on the World Economic Forum website and is excerpted here by permission.