Few would disagree that a lending revolution is well under way, especially in the areas of unsecured retail and small-business lending. The size of the prize is large and attractive. In the coming years, a large part of global banks’ revenues and profits is expected to come from lending to personal and small and medium enterprise (SME) segments. Furthermore, the way that institutions lend to these segments is being influenced by several disruptive forces, such as increased access to customers through deepening smartphone and internet penetration, a tsunami of digital data from multiple sources, fast-evolving customer expectations driven by exposure to e-commerce and consumer internet experience standards, and rapid advances in data computing and advanced analytics techniques.
Globally, several types of competitors—incumbent financial services firms as well as fintech attackers and large digital platform players—are battling for dominance, and in the process changing traditional lending paradigms.
The good news is that lenders have just begun scratching the surface of this opportunity. The lending pie can be expanded many times over through digitization, as it expands to include underserved segments: both new-to-credit customers (especially in emerging markets, where millions of retail and SME customers rely on the informal market) and existing customers with underserved needs (for example, microlending, short-term credit, purpose-linked loans). Thanks to vast, ambient data, a deeper and more automated understanding of consumers and small businesses is possible, while digital channels enable a more convenient and seamless way to serve them.
However, very few lenders have succeeded at scale. Some incumbent lenders are playing catch-up with attacker lenders, saddled with legacy platforms, practices, and organizational constructs, along with an incumbency bias to prevent cannibalization. Others are experimenting to refine their operating models in a bid to become profitable. At the same time, several attacker lenders are struggling to build scale after initial launch, and many have closed shop due to their inability to manage high acquisition costs and minimize fraud, delinquency, risk losses across the cycle.
While digitization is having an impact on the lending value chain across all asset classes for retail and SME segments, the effect is particularly pronounced in unsecured (collateral-free) lending, which is the focus of this article. Collateral-based lending introduces additional considerations around the nature of the underlying collateral (e.g., verification, ownership validation, value assessment) which have not been addressed in this article.
Based on our experiences, we’ve gleaned ten lessons, outlined below across three major themes, that incumbents and attackers alike can follow:
Creating compelling propositions for the digital era
1. Reimagine the core lending product to meet granular needs
A granular understanding of customer needs and, more importantly, context is a critical first step to designing a winning digital lending product. For example, Goldman Sachs, ahead of launching Marcus, surveyed about 10,000 customers in the United States (a relatively well-penetrated credit market) to better understand current pain points.[1] For the “prime borrower,” they offered a proposition that had better rates (vis-à-vis credit cards), no fees (including no late fees), and flexibility in choosing monthly repayment amount and date (for example, linking the date to monthly inflow such as salaries). As a result, within the first year, Marcus disbursed $1.9 billion in loans.
Similarly, several leading lenders have innovated on a number of product dimensions to increase uptake rates of their digital lending product. Consider a few examples:
- Micro-ticket sizes. Companies offering loans in the range of $100 to $1,500—5 to 20 percent the size of prevailing consumer lending tickets—include Cashalo in the Philippines, which offers loans of average ticket size $150, and WeLabs and WeBank in China, offering loans averaging $1,000.
- Bundled products offered at point of sale. Combining payments and lending, some banks offer a line of credit with a card that can be used as a payment instrument at time of purchase or end use (for example, for commerce, travel, medical expenses) and paid back in convenient monthly installments over time. Global fintech Klarna created a “buy now, pay later” offering that an online shopper can access at the time of checkout and repay in convenient installments. In addition to benefiting customers, partner platforms saw a 68 percent increase in order value and 44 percent lower abandonment rates.[2] In India, point-of-sale purchase credit player Bajaj Finserv has amassed about 16.5 million customers through its unique EMI card product, more than India’s top credit card issuers.[3]
- Preapproved offers. To serve existing customers, banks can apply internal data to make preapproved offers that customers can accept online with a few clicks. For example, HDFC Bank in India pioneered the ten-second personal loan for its customers. Similarly, banks can serve nonbanking partners’ customers by offering targeted preapproved loans during the purchase journey. ICICI Bank partnered with PayTM to offer a preapproved credit limit to select customers making purchases on the online marketplace. And a leading Southeast Asian bank has begun to offer its SME customers short-term “top-up” unsecured loans that can be taken out in a few clicks, to augment the traditional term loan. Within two months of launching, conversion rates tripled.
2. Deliver a breakthrough customer experience
Leading lenders set a high bar for experience, not just to beat the existing offline process of visiting a bank branch, but rather to match the experience of purchasing and consuming products on mobile commerce platforms.
Besides the “anywhere, anytime” paperless promise of digital products, leading lenders focus on three key input metrics: time to apply (within minutes, paperless), time to yes (from multiple days to minutes), and time to cash (from weeks to less than 24 hours or even minutes). Doing so is, however, not easy. To improve these experience metrics, lenders have already identified several best practices:
- Leverage design thinking to make customer-facing interfaces more intuitive, with reduced need for data entry, coupled with smart, contextualized prompts and remote assistance (call centers, virtual chatbots) as needed.
- Redesign back-end processes, using a zero-based approach and extreme automation to eliminate manual tasks (for example, machine vision techniques, robotic process automation, screen-scraping technologies), and integrate data trapped in multiple silos to enable near-real-time processing and decision making.
- Access smartphone data and proprietary and third-party data sets to pre-underwrite customers and to minimize data entry and manual checks during the application process. For example, fintech CreditVidya validates applicants’ claimed association with an employer using software development kits embedded in the lender’s app. As a result, according to the company, verification takes minutes instead of days, enabling lenders to give customers faster decisions.
3. Partner extensively to integrate in nonbanking ecosystems
Digitization is transforming how companies fulfill customer needs, leading to the emergence of multiple ecosystems and interconnected businesses. Lenders are seamlessly embedding lending products in end-use purchase customer journeys to make financing available early in the decision process and reducing the friction in getting access to credit (in many cases, using the partners’ data to pre-underwrite customers). By doing so, they create a win-win proposition for partners, as credit fuels additional purchases and engagement on the partner platforms.
For example, a leading Asian player shows customers an offer for preapproved line of credit while they are browsing the latest smartphone models on a partner e-commerce website. The customer can obtain the loan on the e-commerce website without applying for a loan on a separate website. Similarly, accounting platform Xero, in partnership with fintech Waddle, allows small-business customers to draw down working-capital funds against unpaid invoices when they file these invoices in the core accounting software. In China, Didi partnered with China Merchants Bank to offer car installment loans to DiDi drivers, where the credit decisions are based on data about drivers from the Didi platform.[4]
For digital lending franchises, such partnerships will become increasingly critical and a strategic differentiator—not just in acquiring customers at scale, but also in offering novel propositions, getting additional platform data to underwrite customers, and continuing to engage customers well past the loan origination (Exhibit 1). Capitalizing on this advantage, and regulations permitting, several large technology companies with access to large customer bases are making forays in lending-based products to fuel growth on their platforms and unlock new revenue streams.

Leveraging data to drive end-to-end decision making
4. Drink from the data firehose
Ability to harness insights from data is a core strategic capability for digital lenders. The digital era has delivered a tsunami of data, with many new external data sources emerging: telco players, utilities, government platforms, device data (for example, from smartphones and point-of-sale, or POS, machines), nonbanking partner platforms (for example, purchase history on e-commerce platforms), and behavioral data from onboarding journeys.
Typically, for new-to-credit customers, lenders go beyond historical bureau records to evaluate data points spanning multiple data sources, to enable decision-making across key factors:
- Verification of identity. Telco and mobile GPS data collected from the smartphone can be used to verify residential and work addresses.
- Capacity to repay. Income estimation is possible through use of proxies such as telco metadata (e.g., ratio of outgoing to incoming calls); amount paid on utility bills; SMS data on spending, purchases, and deposits; and for SMEs, transaction flow data from point-of-sale machines and QR codes.
- Likelihood to repay. Relevant data types include frequency of utility bill payments, frequency of calls to close family, make of smartphone and nature of operating platform, and manner in which the applicant fills out the form (for example, application forms filled out in all caps or between 1:00 a.m. and 6:00 a.m.).
Furthermore, to ingest and derive insights from such disparate sources, lenders are investing in building state-of-the-art credit data platforms that collate data from multiple internal sources (for example, core banking, loan origination system) and external sources (using API-based architecture). Well-designed data platforms enable continuous training and improvement of the credit engine, which is a combination of multiple advanced analytics and machine-learning models of, for example, propensity to buy, probability of default, and optimal ticket size. Some lenders view building these data platforms as an investment in competitive advantage which requires collecting data over extended time periods along with iterative testing and refinement. Leading lenders, with deep and well-trained models, are able to approve and price customers more effectively than newer competitors with more nascent credit models.
5. Go “all-in” on automating decisions
Leading digital lenders go all-in on automation, striving to replace human decision-making with advanced analytics and machine-learning models. In many cases, these models outperform traditional models and human-based decision making by exploiting new hypotheses and correlations, exhibiting 10 to 50 percent improvement in the models’ predictive power (measured as a Gini coefficient). At a leading Southeast Asian consumer-lending firm, the predictive power of analytics models increased dramatically through the use of non-traditional techniques and external data (Exhibit 2).
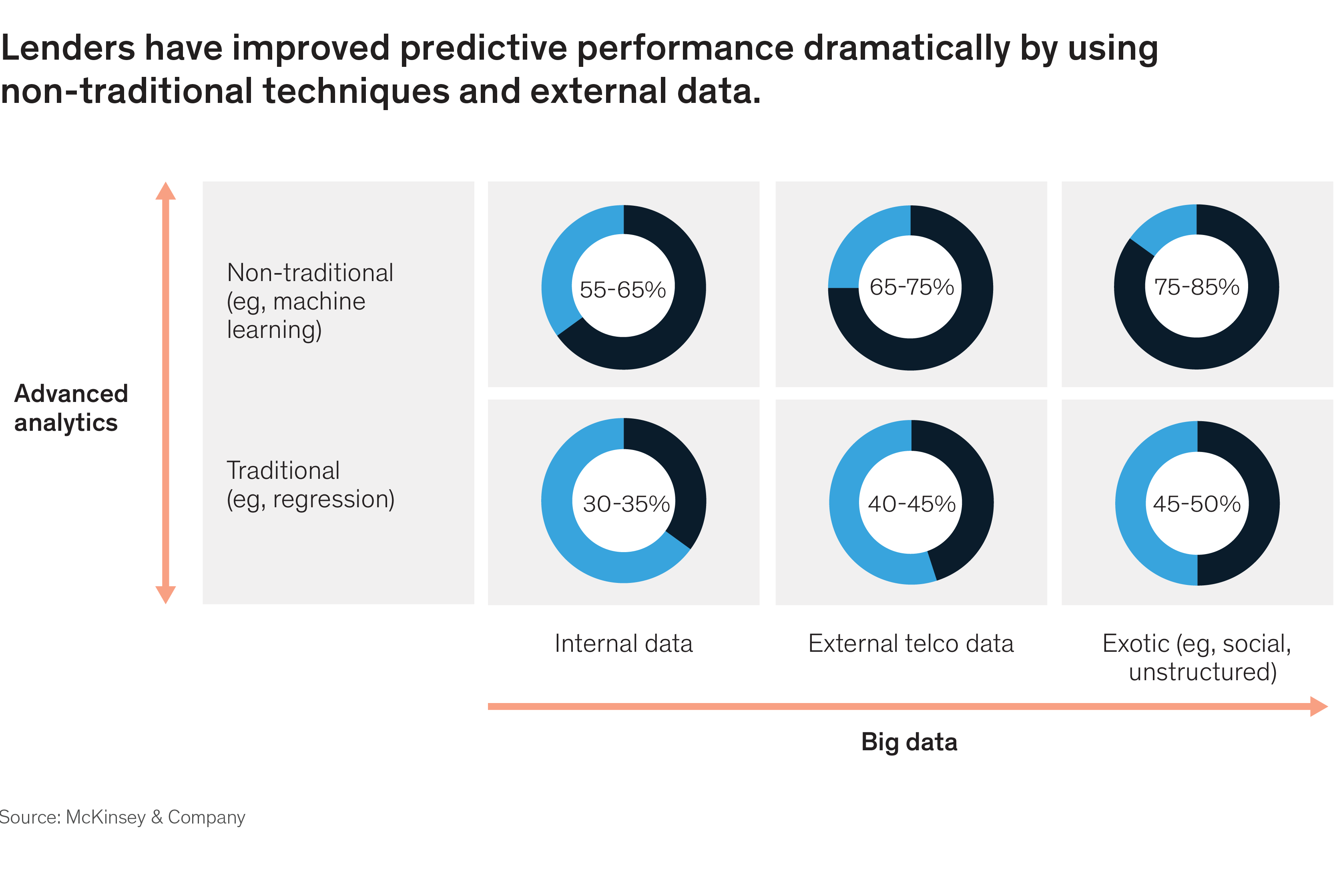
More than 20 kinds of key decisions can potentially be automated across the credit decision cycle, from lead sourcing to ongoing cross-selling and servicing (Exhibit 3).

While their ambitions may be grand, many lenders start small with automation, typically passing a small percentage of decisions through the “fully automated” lane. The rest are processed through a hybrid approach of human judgment and machine-based input. Gradually, lenders migrate more volume (for some decisions up to 80 percent of volume) through the fully automated channels as they develop more confidence—and continuously improve—their decision models.
6. Upgrade to always-on digital risk management practices
In the digital era, the scope of risk management has increased. Lenders need to tackle traditional risk management challenges (for instance, document and signature fraud, likelihood to default) even as they combat new challenges (such as identity fraud, data hacks, denial-of-service attacks) to maintain the trust of customers and regulators and prevent catastrophic losses. Furthermore, today risk management needs to be “always on,” and lenders need to adopt the mind-set of continuous underwriting, rather than one-time underwriting at the time of loan application or renewal.
To do so, risk management requires state-of-the-art triggers and early-warning systems to enable effective loan monitoring. But that’s not enough. In the digital era, risk management also needs a fundamentally new set of capabilities, such as machine-learning techniques to identify fraudulent transaction patterns (for example, drawdowns at odd times, pattern of stating employer name in the application form), real-time integration with industry databases (for example, blacklist, defaulters), reading of SMS history of customers to identify new triggers (for example, new credit line booked, large payment, delay in receiving salary), predictive models and actions to increase probability of repayment (for example, detection of a low balance close to the repayment date triggering automated SMS and email-based reminders).
Similarly, to enhance collections efficiency, machine-learning models can be used to move away from broad customer archetypes and resolution strategies to 100 or more microsegments, each with a tailored approach to resolution. One consumer finance lending company in Southeast Asia is using machine-learning models to microsegment customers with payments past due to understand the likelihood of repayment and the appropriate communication strategy (that is, which channel to use, what time of the day to contact, what message to use), which it scaled through its various channels (SMS blasts, call centers, agents) to enhance overall collection efficiency.
7. Race to achieve scale early
There are enormous benefits of scale in the digital lending business, setting up a virtuous cycle of better data, improved underwriting, stronger partnerships, and enhanced acquisition (Exhibit 4).
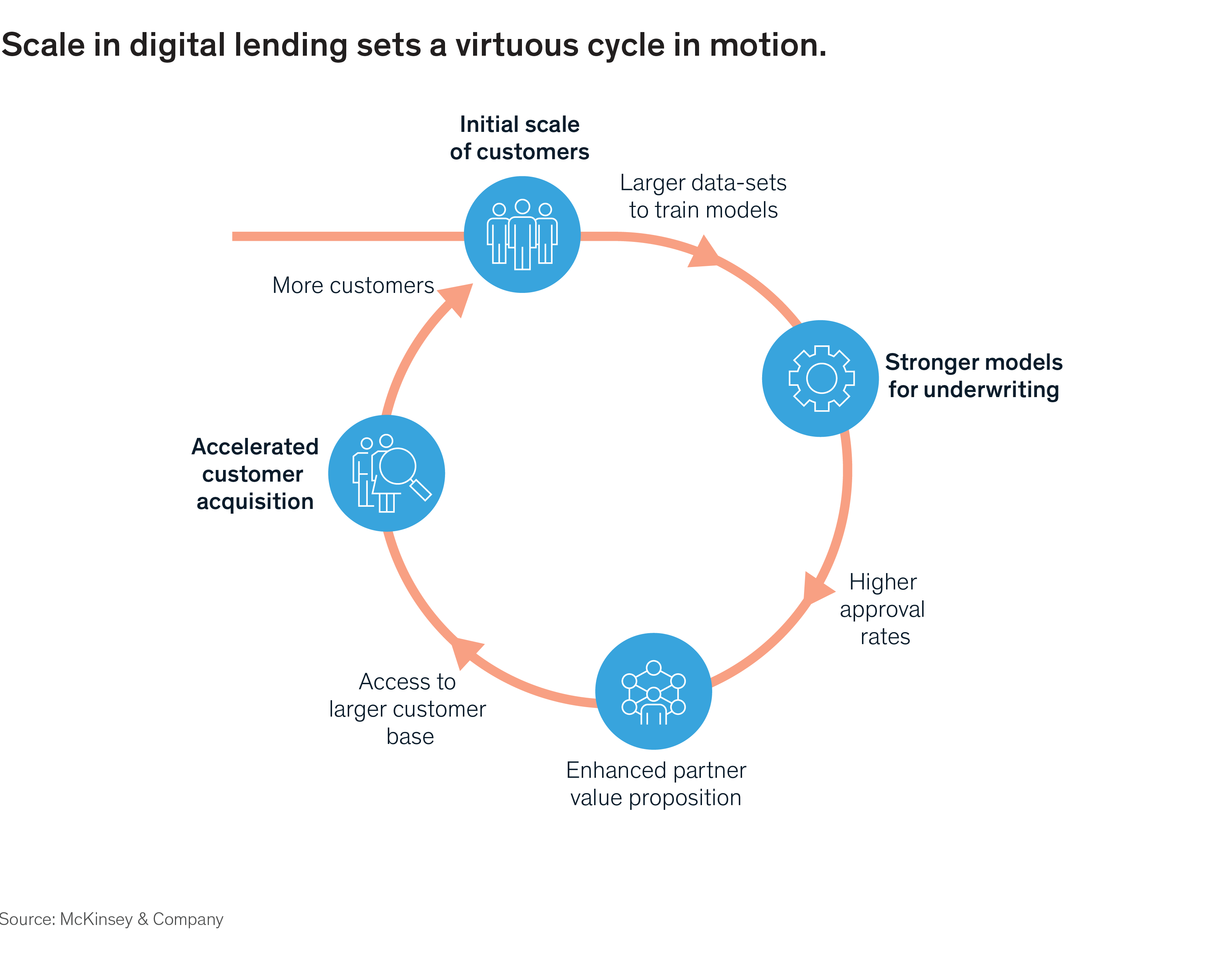
Lenders that amass customers early benefit from access to larger sets of behavioral data to better identify and manage key drivers of credit risk. For existing customers, lenders can offer more profitable, higher-ticket loans to the more creditworthy applicants, increasing the lifetime value of customers. For example, Bajaj Finance in India and Home Credit in Southeast Asia typically begin the relationship with new-to-credit customers with a small-ticket consumer-durable loan, followed by offering more profitable, higher-ticket cash loans to a subset of customers that exhibit good credit characteristics. For new customers, lenders can create proxy “bad profiles” based on past data, using the data for more effective screening and pricing of new customers (with lesser historical data) when they apply.
As a result, at-scale lenders are able to approve and underwrite a larger pool of customers, on account of stronger and continuously improving credit models. This advantage makes at-scale lending lenders more attractive to nonbanking partners, who seek to offer credit to reduce drop-off rates in customer purchases on their platforms and can in turn offer a captive consumer base, along with valuable data from the platform to further strengthen credit underwriting.
Adopting digital ways of working
8. Innovate faster using modern engineering and technology architecture
Digital lenders operate in a fast-evolving landscape and need to maintain a rapid pace of innovation, delivering new features in weeks, as opposed to months and years. In so doing, they also must be able to exchange data, information, and services with a range of external partners.
Inspired by Silicon Valley giants, digital lenders have invested in nimble technology stacks. While fintech natives have the advantage of starting fresh and building modern platforms from scratch, incumbent lenders have built fast-speed layers on top of legacy core transaction platforms. These new layers make it easier to maintain and scale micro-services-based architecture along with API gateways to enable easy integration with a plethora of external data and solution partners. Furthermore, by adopting DevOps practices and implementing the DevOps toolchain, lenders are automating parts of the software delivery lifecycle and dramatically reducing the time to market for new features.
9. Master the art of digital marketing and remote assist to scale effectively
Digital acquisition costs can make or break loan-unit economics, with many lenders struggling to contain costs as they scale. A leading Asian digital lender, through effective marshaling of digital marketing practices, was able to maintain a digital cost of acquisition that was 20 to 60 percent lower than traditional offline marketing, and with this advantage, it successfully built scale.
Effective digital marketing goes beyond traditional SEO and keyword optimization. It involves acting on the comprehensive set of levers that include using digital-first creatives, adopting personalization at scale, and applying advanced analytics and machine learning to implement targeting models that optimize when, where, how, and with what message to approach customers. Furthermore, digital marketing performance can be dramatically improved by adopting an agile approach wherein a continuous stream of experiments and A/B tests are run to determine which hypothesis are effective for scaling. This experiment-led approach needs to be backed by a modern marketing technology stack that captures granular data across different points of the targeting journey to inform the feedback loop on campaigns.
Another lever to improve acquisition effectiveness is seamlessly blending intelligent prompts and remote-assist models in the onboarding journey to reduce drop-offs. At one leading digital lender in Asia, a well-functioning assist infrastructure integrated with the digital marketing efforts helped reduce application drop-offs by up to 40 percent and decrease the overall cost of digital acquisition.
10. Build muscle for continuous learning and innovation
Internally, leading digital lenders are more nimble and agile, similar to leading technology companies. In particular, they have committed to developing the capacity to learn and continuously improve their end-to-end operating model and speed of innovation.
To begin with, their talent is more diverse and comes from a variety of backgrounds, including experience designers, data engineers, machine-learning experts, technical architects, application developers, DevOps engineers, and digital-marketing specialists. These positions often outnumber traditional banking roles.
These leaders embrace an agile way of working. They set up cross-functional and autonomous teams (versus siloed departments), operate in small and frequent sprints that deliver an end-to-end functionality (versus multiyear projects), and emphasize rapid experimentation and time to market through controlled launches of products and models that they test frequently with end users in order to iterate rapidly before scaling.
Additionally, they invest heavily in building well-functioning feedback loops enabled by: setting granular operating metrics that need to be optimized, establishing tech and data infrastructure to collect data across end-to-end journeys, and making it available in real time for teams to analyze in order to identify corrective actions. These feedback loops are applied across operating model, whether it be digital marketing, underwriting, risk management, or product design.
For incumbent lenders, transforming from slow-moving caterpillars to agile butterflies is a formidable challenge. As many incumbent institutions that have launched digital lending franchises have realized, an easier way is to set up self-contained “digital garages” that pilot the desired practices and culture. Then they can expand slowly to include the broader organization as the business becomes larger.
Despite a wealth of opportunities, success is not easy in digital lending. Launching and scaling a profitable digital lending franchise requires multiple considerations.
For institutions planning to launch a new unsecured lending business or that have not fully embraced digitization in their existing business, the lessons outlined above can act as guideposts to making key strategic choices (e.g., differentiated proposition, partnerships needed for acquisition and data, technology stack, talent model) and execution imperatives (e.g., design of low-touch operating model, setting-up agile teams, instituting infrastructure for continuous learning from day one).
Lenders that are active in digital lending and in the process of scaling up their franchises can use the ten lessons to pressure test their plans and identify implications for their context. These could range from new strategic choices (e.g., what ecosystem partnerships do you need to enable a sustainable cost of customer acquisition? do you need to redesign the core offering or experience to better differentiate from competition?) to gaps in execution that must be filled (e.g., do you need to institutionalize a credit data platform to enable continuous feedback into your credit and decision models? what are the next wave of decisions in the value chain that can be automated?).
Whatever the starting position, a rigorous scrutiny of plans, operations, and business model in digital lending will be necessary to capture the large opportunity ahead, and not be left behind in an increasingly dynamic and competitive landscape.
[1] Olivia Oran, “Goldman targets credit-card borrowers with new lending business,” Reuters, October 13, 2016, reuters.com.
[2] Klarna.com.
[3] Shobhana Subramanian, “Lack of banking licence is not something we miss: Bajaj Finserv MD,” Financial Express, March 28, 2019, financialexpress.com.
[4] Tracey Xiang, “China fintech: Ride-hailing network DiDi now offering full-fledged financial services,” China Tech Economy (blog), July 10, 2018, chinatechecon.wordpress.com.