Manufacturers globally are under intense pressure to meet demand under increasingly challenging circumstances. In a resource-constrained environment where talent gaps and supply chain shortages are the norm, digital twins are emerging as a frontrunner technology for rapidly scaling capacity, increasing resilience, and driving more efficient operations.
In fast-paced, continuous operations, factory digital twins—real-time virtual representations of the factory—provide manufacturers with the ability to support faster, smarter, and more cost-effective decision making. They can deepen manufacturers’ understanding of complex physical systems and production operations, optimize production scheduling, or simulate “what-if” scenarios to understand the impact of new product introductions, for example.
The technology is advancing at lightning speed, and a recent McKinsey survey of senior executives revealed that most can now see a practical application for digital twins in their production operations. Deploying a digital twin is no longer an option for industry leaders only. The “factory of the future” is here and unlocking value today.
Factory digital twins are top of mind for leaders
According to McKinsey’s 2022 survey of senior executives in industrials, two burning issues are keeping manufacturing leaders awake at night: material and labor constraints caused by rising costs and talent gaps, and a need for improved production visibility through better demand forecasting, inventory processes, manufacturing flexibility, and real-time visibility of the factory floor.1
Factory digital twins are becoming a highly sought-after technology to solve these problems, the survey found. Across industries, 86 percent of respondents said a digital twin was applicable to their organization. Some 44 percent said they have already implemented a digital twin, while 15 percent were planning to deploy one (Exhibit 1).
How factory digital twins work
Factory digital twins provide a comprehensive model of the factory floor. They simulate outcomes from real-time factory conditions, enabling “what-if” analyses across production scenarios, such as process or layout changes. In their most advanced state, they can be integrated into real-time decision making, such as production scheduling—either with manual review and intervention or through full automation.
Digital twin use cases vary based on the operational context of the factory. During initial investment and build of a greenfield factory, for example, a digital twin can validate layout design, optimize the footprint, and estimate inventory size. Depending on the level of detail of the twin, they can even evaluate spatial parameters for assets—for example, clearances, ergonomics, and employee movement within a cell.
In more established operations, factory digital twins can predict production bottlenecks where traditional modeling in spreadsheets falls short. Hard-to-predict stochastic processes, inventory buffers, material travel times, and changeovers can all be modeled with high fidelity using live data.
Insights from the twin can be applied to different types of decision time scenarios, too, from slower decisions, such as line balancing and prioritization of continuous improvement opportunities, to real-time decisions, like optimizing production schedules.
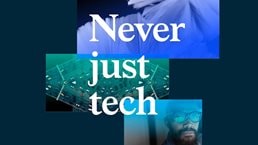
Creating value beyond the hype
Delivering value in the factory environment
Factory digital twins are unlocking value in all kinds of industries and use cases. A factory digital twin developed and deployed for an industrials player was recently used to redesign the production schedule, compressing overtime requirements at an assembly plant and resulting in a 5 to 7 percent monthly cost saving.
By accurately simulating real-time bottlenecks on the production line, the digital twin also uncovered hidden blockages in the manufacturing process. The model integrated into the existing manufacturing execution system (MES) platforms, Internet of Things (IoT) devices, and inventory databases to determine the optimal sequencing of different product lines to minimize downtime. This was achieved within the parameters of customer delivery requirements and the physical confines of warehouse storage and production line capacity.
Similarly, a factory digital twin developed for a metal fabrication plant has helped identify ideal batch sizes and production sequences to optimize the scheduling of thousands of potential product combinations across four parallel production lines. To handle this level of complexity, an AI-based agent was trained to build the optimal order sequence using the digital twin through reinforcement learning (RL). The RL algorithm created significant cost reduction and yield stability when compared to manual scheduling.
Building a modular, scalable digital twin
Digital twins operate by integrating several data sources together and arranging tech feeds along a common data pathway (the “tech stack”) to analyze data and visualize performance. For best results, the tech stack should be modular, scalable, and provide a single source of truth (Exhibit 2).
While many manufacturers tend to opt for natively built digital twins that are designed to bespoke specifications, there are a variety of “starter packs” that can be incorporated into digital twin design, supporting interconnected data, providing a viable user interface, or acting as an optimizer for different production inputs.
Universally, a modular tech stack is designed using building block components that are clearly segmented and standardized. A scalable stack has standard data integration, application program interfaces (APIs), and templates to ensure modular components can be added with minimal effort. Creating a single source of truth, such as a unified name space (UNS) architecture, ensures data is properly classified, structured, and accessed in such a way that insights are consistently formed.
Sourcing, storing, and processing data: Data sits at the base of the tech stack, comprising production data sourced from PLCs and MES platforms to indicate the status of the line and most recent cycle times by asset. Inventory data shows raw-material availability, current work in progress, and finished goods, while demand data is ingested either directly from the customer or through the ERP.
Systematic data cleaning is critical to ensure modeling is conducted in a repeatable and expected manner. Data is cleaned, structured, and compiled, typically into intermediate data tables designed for consumption by the simulation tool.
Creating a standard language: Data service integration software enables data from disparate streams to be united into a common data pathway for processing and segmentation. This allows data to be manipulated and organized into a useful and categorical “language” for integration. Creating one common data model that integrates disparate data sources enables a step-change in operational insights. One architecture approach, the UNS, applies a common naming convention for all business data in a clear and easy-to-understand way that vastly reduces the complexity of scaling up use cases.
Layering in simulation software: The most accurate way to simulate the factory floor is with discrete event simulation software or natively built code. This produces a virtual rendering of the factory to run thousands of simulated production sequences to identify bottlenecks and production constraints.
Optimization: Layering optimizer software on top of a digital simulation enables the digital twin to run millions of hypothetical production sequences and isolate the optimal sequences that maximize productive time. Although optimization approaches have been around for decades, recent advancements such as genetic algorithms, Baysien-based “optionization,” active learning, and deep reinforcement learning are game changing in creating new ways to optimize the factory. For example, incorporating machine learning (ML) algorithms allows for sequences to respond to both historic patterns and real-time variance to create a system of repeatable business rules that can step-change production output. Combining these ML and optimization approaches with a simulated replica of the plant and leveraging the latest in high-performance computing is allowing companies to drive a new level of performance in real time.
A recent deployment of factory digital twins illustrates how these elements translate into real-world value. Factory leaders connected multiple, disparate data sources into a common operating picture that replicated a production line in a virtual environment. This enabled the team to monitor the amount of time each unit spent in every step of the production process—measuring the amount of time that the processing step was “starved” (sitting idle waiting to receive the next unit) or “blocked” (waiting to advance a unit to the next step in production after work had been completed).
Putting this together, the team was able to identify different sequences that reduced the overall processing time, specifically by minimizing the blocked and starved time at the critical bottleneck station. By leveraging a rudimentary optimizer solution to identify and develop repeatable sequencing rules that optimized the production sequence for the bottleneck station, the team reduced total processing time by about four percent.
How to get started
While most surveyed manufacturing executives now see an application for digital twins, the desire to implement a digital twin does not always mean manufacturers are ready to do so. Executives often highlight several specific challenges, including limited awareness of the full capabilities of a digital twin; fragmented and arcane data landscapes that inhibit high-impact, scalable solutions; and a lack of in-house talent capable of building and deploying a digital twin solution.
Overcoming these obstacles starts with adopting an iterative, agile way of working based on continuous testing, validation, and refinement of algorithmic logic. This approach helps increase the digital twin’s accuracy prior to deployment—raising the odds of long-term adoption.
External support may be needed to fill talent gaps. To design and build a digital twin, one large manufacturer partnered with a cross-functional product team of industrial or manufacturing engineers, operations managers, data engineers or scientists, and IT architects to connect data sources, trial a minimum viable product, and build a scalable solution.
The first step of digital twin development involved rapidly building a proof of concept to demonstrate the feasibility of the twin and refine impact estimates. Next, the team targeted a higher fidelity, minimum viable product simulation, fully identifying data feeds and designing the future-state architecture. At this point, some value capture was already possible by highlighting process change opportunities, for example, optimizing staffing, understanding the impacts of product mix on the line, and identifying bottlenecks under different conditions.
Then, the simulation was connected to live data feeds and embedded into operations, transitioning from the sandbox to the production environment. Finally, optimizer platforms were added, using ML algorithms to identify optimal scheduling patterns or campaigns in real time.
This type of development journey can span two to three months or take more than a year, depending on the experience and capabilities of the product team and the complexity of the factory.
Unlocking the full potential from digital twins can be revolutionary when scaled across the factory network. This next frontier may be incredibly powerful, given that many industrials players have complex and vertically integrated production systems with component fabrication, assembly, and distribution occurring across many different nodes. If each of these nodes had its own digital twin, the end-to-end network could be optimized for incredibly complex planning problems and capacity analytics.
Factory digital twins are likely to continue to evolve over the next several years as virtual models integrate closely with generative AI technologies. It is feasible that high-functioning AI language models could interact more seamlessly with factory leadership and make recommendations in real time, alerting operators and managers to potential improvements or ways to address unexpected disruptions and estimated recovery timelines. As these models and AI agents become more sophisticated and integrated, they will likely start to interact upstream to understand potential disruptions from the supply chain as well as downstream on changes in demand patterns or shifting customer behaviors.
The possibilities this technology offers today—and will offer in the future—are changing the game for how manufacturers make decisions and drive efficiency. In a world where fast decision making can unlock competitive advantage, or help manufacturers adapt to disruption and economic headwinds, digital twins are set to evolve from a nice-to-have technology into a must-have tool for manufacturers of all kinds—and may eventually be required to interact in a fully virtualized supply chain.