Industrial companies have run headlong into a scale problem in using the cloud for operational technology (OT). Issues include distinct differences in how technology is architected at each site, a sometimes dangerous operating environment, and isolation measures that have historically made it difficult to transfer operational data to the cloud and leverage it. These are issues across industrial sectors, from mines and agribusinesses to power plants, manufacturing plants, and other distributed industrial sites.
These issues impede many industrial sites from capturing the cloud’s massive value potential. Our experience shows that about two-thirds of leading-edge use cases implemented at lighthouse sites are enabled by cloud technologies. Factories that have implemented these use cases have seen productivity increases of up to 50 percent, overall equipment effectiveness gains of up to 30 percent, and decreases in cost of quality (such as scrap cost) of up to 70 percent.1 One European steel plant deployed more than 50 AI use cases to achieve a 15 percent increase in EBITDA—a whopping leap in an industry where single-digit-percentage EBITDA growth is cause for celebration.
Recent technology advances present opportunities for industrial companies to capture a much greater share of value in the cloud, provided they adopt the right architecture for cloud-enabled OT. Our work with clients has enabled us to develop a blueprint for such an architecture based on features that should be familiar to IT and cloud professionals, giving companies a known starting point.
Industrial sites and the challenge of the cloud
Many industrial sites face an array of hurdles to using the cloud effectively, including the following three that are most common:
- Unique sites. Industrial sites are heavily customized for the products they produce and how they produce them. Sensors, data-management methods, and controls vary greatly. Even the same finished goods can be produced at different sites in completely different ways, using completely different technology. More specifically, equipment data is often structured differently between sites—and even within a single site. This makes relating similar data sets about equipment almost impossible and eliminates opportunities to conduct analysis of such data at any real scale. Emerging technology such as generative AI with its wealth of unstructured data has exacerbated the issue.
- Systems for physical safety. Many industrial sites rely on on-premises technology, such as maintenance systems, personnel location trackers, and proximity detectors, to help keep workers safe. There is no margin for error when a failure of any of these systems can jeopardize physical safety. Site infrastructure is controlled—and protected—in part or entirely by specially designed industrial process controllers.
- Unreliable connectivity. Many sites are in remote locations that may not be able to reliably use terrestrial networks, so they use satellite links that are not designed to cost-effectively support mass data transfer. For safety and security reasons, some systems are intentionally disconnected from the network, which keeps them safe from cyberattacks but traps useful data. Sites can also be vast, spanning square miles, with mobile equipment that might move hundreds of miles in any given day in harsh environments. Maintaining reliable connectivity, even with local Wi-Fi or private 5G, is an ongoing headache. As a result, it is difficult to regularly transfer data from the specific equipment and systems to the cloud.
Would you like to learn more about Cloud by McKinsey?
An architecture for industrial sites
Industrial sites’ challenges can be daunting, but advances in cloud computing—particularly in security and edge computing—have come a long way. Some industrial sites are already adopting standards in site data collection, such as OPC Unified Architecture (OPC UA), a machine-to-machine communication protocol that allows control systems to exchange data securely and consistently.
To take advantage of these developments and maximize value from the cloud, industrial companies should consider a hybrid cloud architecture, which is helpful to consider at three levels:
- site-level assets and activities, where cloud-based tools can improve data operations (collection and management) at the site
- secure connectivity, which focuses on ensuring exchanges between the cloud and on-premises environments are consistent and secure
- the cloud platform, where services can provide the site with capabilities
This architecture balances on-premises productivity with the advantages of cloud scale by introducing new technology, such as edge computing, to the existing site-level technology stack. It unlocks site-level access to the cloud in a secure, reliable way, so that companies can deploy cloud-enabled solutions to sites without reliability concerns (exhibit).
This architecture provides a baseline set of components that can be used to extract data from the site and process it in the cloud. While many of these elements are familiar to most industrial companies’ CIOs and enterprise architects, few have implemented them. Investment in the infrastructure to bring edge computing and cloud capabilities to industrial sites can be significant, so technology leaders must make architecture decisions that position cloud services to deliver the most impact. It is critical to define those that can be used by the greatest number of sites, standardize the application development stack for cloud and edge capabilities, define cost-effective data transfer standards, and develop a data lake strategy that doesn’t overwhelm developers and data scientists with data that isn’t useful.
Site assets
Site OT is responsible for making production happen. Automated process controllers, autonomous equipment, and site information systems generally store OT data in site-based servers, which is a missed opportunity. Cloud-based tools can simplify data management throughout its life cycle by automating routine tasks, providing self-service tools, and—crucially—freeing up users for other tasks.
Edge technology is bringing more cloud-based tools to site assets and is especially useful to remote sites as they overcome telecommunication reliability issues, and it can even perform some analytical computations on-site. Edge computing works as a local holding pen for data and can be connected to existing systems with adapters. Applying an adapter pattern to site technology enables a “plug-and-play” approach that allows other technologies to quickly access site data and reduces the cost of maintaining site-level cloud integrations. Adapter patterns also allow companies to deploy a more standard set of edge services and cloud integrations by making the necessary connections between standard edge or cloud services and on-premises systems. As a result, when rolling out a service to a new site, only the adapter needs to be configured to communicate with the on-premises assets.
Once edge computing is in place, organizations can build new, high-impact user-facing channels that give operators and site managers access to cloud-generated insights. Dashboards, apps, and web interfaces can be developed using cost-effective cloud technology, enabling sites to modernize and enhance operator workflow.
Secure connectivity
Access to the cloud may be interrupted by connectivity gaps, especially since many industrial sites are remote. Edge computing can be useful during these connectivity gaps; even though industrial sites generate large volumes of data, not all of it has to be processed at once. Edge computing can store a subset of data at a site, and in some cases can even provide cloud compute capabilities, thus allowing sites to continue to use cloud capabilities even if network connectivity is lost.
Of course, the corresponding edge computing architectures—the amount of computing needed to store and process data before sending it to the cloud—will vary based on the size of the connectivity gap, the amount of data to be transferred, and the use of digital assets, such as sensors and recording devices. Edge computing also manages data’s return trip from the cloud to sites, making cloud-dependent, on-site applications faster and more reliable, since it reduces reliance on network connectivity. It even gives sites access to real-time data processing using the same algorithms and models hosted in the cloud, a helpful feature that makes temporary connectivity gaps less likely to interfere with sites’ ability to process data.
For sites that operate remotely, multiple methods of communication may be used to connect to the cloud and to ensure reliability. Not all methods may be dedicated to a single site and isolated. Still, industrial sites can benefit from cloud-enabled features without compromising cybersecurity by using such security elements as end-to-end encryption, dedicated virtual private networks (VPNs), and certificate-based API calls (a way to authenticate the maker of a request). This kind of vigilance is based on zero-trust security, which requires all users, both inside and outside an organization, to be authenticated before gaining access to applications and data. It balances connectivity with security and can help companies avoid costly breaches and remain compliant, while extracting the benefits of the cloud.2
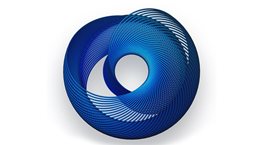
Cloud by McKinsey Insights
Cloud platforms
Cloud technology is well suited to analytics that use operational data or build applications that make sites safer, easier to maintain, and more productive. Once operational data reaches the cloud, it can be analyzed and used in applications. It also positions sites to take advantage of cloud providers’ services to glean value from innovations such as generative AI.
Cloud-based data life-cycle management decouples data storage from on-site infrastructure, guaranteeing that data will always be available. It also allows users and organizations to easily add or remove storage and computing resources as needed, a notable benefit for companies whose data volumes fluctuate in predictable ways, such as by season.
Stakeholders can also use cloud solutions, such as cloud-based machine learning, to analyze large volumes of data, find patterns, and make informed decisions. Forward-thinking users could also tap into data from their organization’s other sites to discern patterns for high-impact use cases. A global manufacturer struggled to glean insights from data pulled from disparate sources. It used one cloud-based solution to collect and aggregate real-time data in a central data lake, and another to build, train, and deploy machine learning models at scale to maximize site resources. These efforts boosted the company’s profits and allowed site managers to predict equipment failures up to 90 days in advance.3
Analytics
Cloud technology has the unique ability to perform the analytics required to inspect materials at site environments and to learn—with guidance from a human operator—to spot problematic products or materials. Significantly for industrial leaders, many staff already have some knowledge that can help data scientists and software developers quickly develop the right algorithms. Some local engineers who have been trained in cloud technology might even be able to develop such algorithms independently. More complex and rigorous solutions—from formal data science disciplines that require algorithms to out-of-the-box low-code/no-code data science solutions—can augment their work. And of course, analytics can produce KPIs that feed dashboards and reports to help decision makers monitor performance, identify issues, and achieve consistent productivity gains.
One large wireless equipment manufacturer developed a new factory around 5G and Industry 4.0 disciplines, combining private 5G, cloud-driven analytics, and cloud-native services. Over the course of 18 months, its team delivered at least 34 cloud-enabled applications and analytics use cases that included digital performance management, augmented reality, and digital twins on an industrial IoT platform. One result: throughput increased by 10 to 15 percent.
Taking full advantage of cloud initiatives requires changes in how companies work. CIOs need to ensure that teams are trained on DevOps capabilities to support the development and deployment of cloud capabilities.
Applications
Useful cloud-enabled applications can provide operators with timely, easily accessible, and insightful information that enhances their work. But their acceptance often hinges on operators’ ability to trust the data and insights they provide, which is where superior application design becomes critical.
This is not to say that industrial sites don’t already use cloud applications. Some of them do. But the back-end structure of most sites’ architecture requires each application to be tailored to a specific data stream to be usable. The architecture we sketch out, on the other hand, describes how sites can both extract data from the cloud and move data into it, expanding the types of usable applications. For example, applications that allow users to use data from sources such as IoT devices, which generate a continuous stream of data, can be built using a subset of the components outlined above. The result is the ability to convert as much data into insights as users may need, while maintaining the global availability and centralized security the cloud offers.
Getting started
Although the technology and many capabilities are already available, bringing the cloud to industrial sites and integrating OT needs to be carefully choreographed. Broadly, industrial companies should consider doing three things.
First, identify and clarify the value and the steps needed to transition to the new architecture and capture that value.4 Decision makers should articulate the kind and magnitude of impact they’re seeking at the proof-of-concept stage and quantify it throughout the implementation.
Next, teams should develop a thorough plan that addresses how they will implement edge, develop adapters, and deploy new solutions without disrupting safety or productivity. This is a must for site technology. The plan should tie together cloud use cases, on-premises technology considerations, cloud considerations, and contingency plans should a software deployment prove unsuccessful or inadvertently disrupt operations.
Finally, it’s important to proceed with use case pilots at the proof-of-concept stage even if the conditions or the data are less than perfect. Instead, the emphasis should be on deploying solutions—even experiments—and learning from experience. For instance, a low-earth-orbit satellite may be a viable solution for a site’s connectivity challenges, even if it isn’t fully integrated with the site’s network. Using the results from the proof-of-concept stage, stakeholders can design their enterprise’s full target-state architecture.
Technological advances have put cloud OT well within reach for industrial sites. Getting started with a hybrid architecture is the first step toward tapping into the value potential of the cloud.