Most R&D executives agree that data, analytics, and the Internet of Things will transform future product development. But only a fifth of companies think their current efforts are bearing fruit.
There's a virtuous circle turning with enough force to change the world. Fast, efficient communication networks and low-cost electronics have allowed manufacturers to connect their products to the internet at a bewildering rate into the internet of things (IoT). Estimates vary widely, but by 2021 there are likely to be between 20 and 50 billion connected devices in the world. Those products, from computers and smart phones to microcontrollers embedded in toys, lightbulbs, and industrial machines, will transmit an estimated 3.3 zettabytes1 of data per year. Meanwhile, computing power continues to grow even faster than the 24 percent annual rise in data volumes. As the world's production of data expands, so does its ability to process that data and transform it into valuable information.
Companies in many sectors have already achieved notable results through application of big data and analytics in their R&D activities. One pharma company used advanced analytics to inform its choice of clinical trial sites, helping it to reduce time to market by 15 percent and trial costs by more than 10 percent. Consolidating and analyzing data from disparate systems helped a computer OEM identify 11 productivity measures that led to a 15 percent reduction in R&D man-days and 11 percent savings in R&D costs. And a car manufacturer used customer-preference analysis to reduce the complexity its product offering, cutting costs by 20 percent.
The executives in our global R&D readiness survey are well aware of the potential of big data, IoT, and advanced analytics. About 75 percent of them say that these technologies will play a pivotal role in their future R&D activities.
Their role today, however, is a minor one. Only 25 percent of respondents say their organizations make wide use of advanced-analytics techniques in R&D, and only 16 percent say the same about big data and IoT (Exhibit 1).
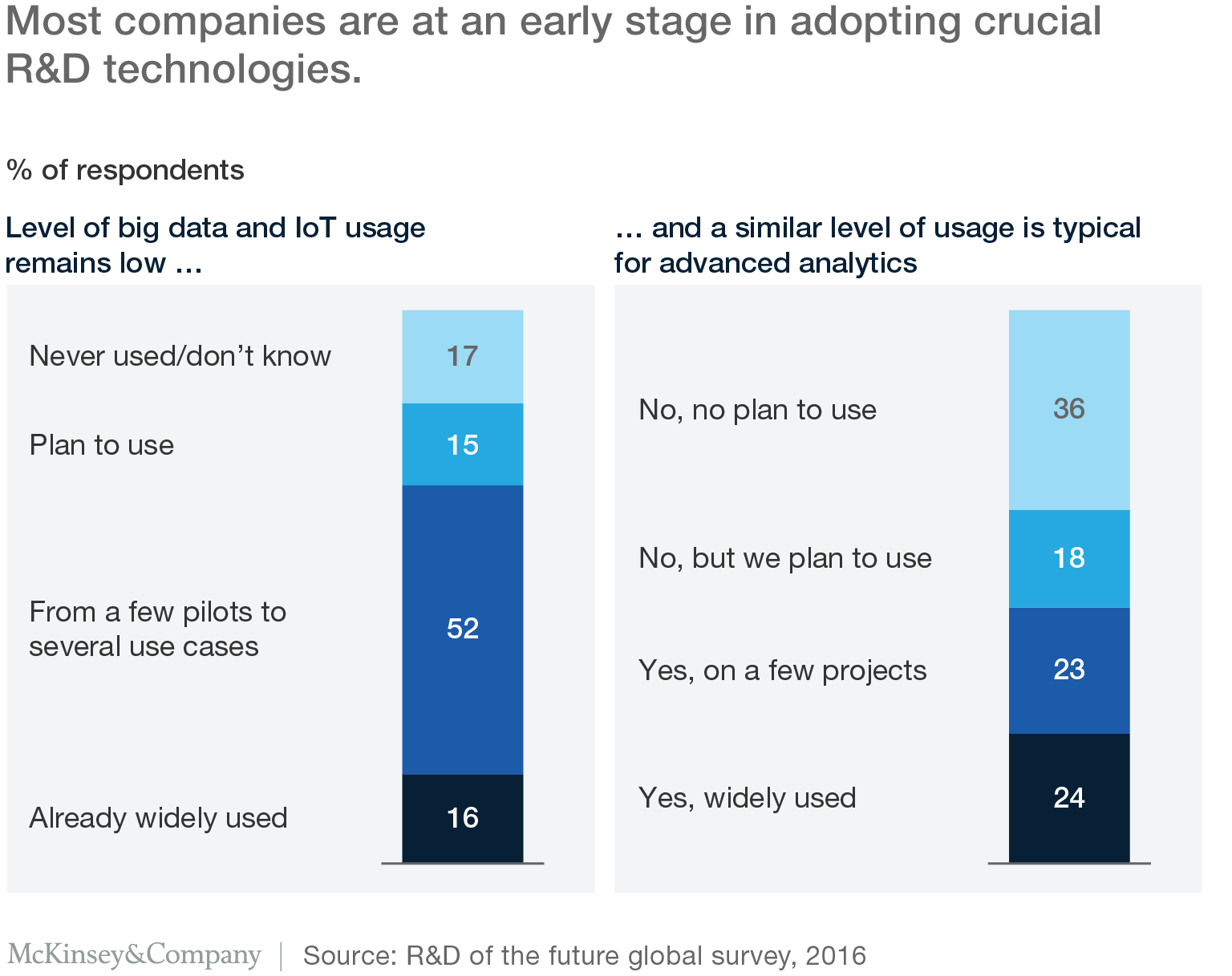
More troubling, across industries, geographies, and roles, an overwhelming majority of respondents think their companies are "behind the curve" in their adoption of these techniques (Exhibit 2).

When we asked respondents why they hadn't progressed their efforts in these areas, they cited a combination of organizational and technical barriers. Many admitted that their organizations didn't yet understand how to integrate IoT or big data into their processes and business models.
In some companies, it is clear that a mindset shift is required before progress can be made. "Why would social media know better than me how to develop my products?" said one executive, when asked about the use of internet-derived customer insights to inform product development. "We have enough difficulty integrating our own requirements, we don't need additional inputs," was the response of another. Other respondents suggested that a significant roadblock was a lack of capabilities and resources, from appropriate data to the IT personnel and analytics specialists required to make sense of it.
Getting it right
To realize the potential of big data and advanced analytics, we believe that companies need to get four things right.
1. Create a compelling and ambitious vision
To align the organization from the top management on down, companies need to create and communicate a clear, compelling vision. That vision should focus not on the use of specific technologies, or on data in general, but on the business priorities they will address. Most companies find it useful to articulate their vision over three horizons:
- Building excitement. Which use cases can be developed, tested, and deployed rapidly to deliver short term wins and to create pull from the wider organization?
- Expanding and consolidating. How can successful applications be scaled up and rolled out across the business? What organizational capabilities and governance processes will be needed to allow that to happen in a quick, but orderly manner?
- Delivering a competitive edge. How can the organization turn its emerging skills, resources, and knowledge into sources of competitive advantage?
2. Adopt a case-based approach
Rather than trying to do everything at once, winning players focus their efforts on a few targeted, high-impact use cases. While the optimal set of use-cases will be specific to each company, they typically address one of three key strategic questions faced by many R&D departments:
- How can we optimize the organization's product definition and innovation pipeline?
- How can we maximize development efficiency?
- How can we create new products or services that monetize big data, advanced analytics, or IoT technologies?
To address the first question, an organization might pursue approaches that use customer insights to increase the hit-rate for new-product introductions. To address the second, it can analyze its internal processes in order to minimize project costs or reduce time to market. Answers to the third question might include the application of IoT and advanced analytics to build predictive-maintenance or on-line support capabilities into products, or to enable the transition to a pay-per-use business model.
3. Build the right foundations
When developing the infrastructure, they need to support their data-driven R&D efforts, companies need to balance flexibility and speed of execution against sufficient structure and discipline to ensure solutions are robust, scalable, and sustainable.
- Data. Start with the data you have, taking steps to manage, structure, and consolidate the existing data within the business. Over time, enhance these resources with data from more complex sources, such as unstructured input from customers or other external sources
- Analytics. The world of data analytics is evolving extremely rapidly. Companies should start off right—using external expertise from academia or industry specialists to access the latest techniques. They should be careful, however, not to treat analytics as a black box. Solutions will need to be tailored to the needs of the business, and their impact should be measured in business-relevant ways, such as impact on P&L.
- IT. Big impact doesn't require big IT investments. It is usually better to pilot pragmatically, using cloud solutions or workarounds to overcome technical barriers. Once the business case for a new approach is clear, the organization can invest to develop the appropriate IT infrastructure.
- People. Analysts and data scientists are important, but they are not the scarce resource they once were. Where many organizations struggle is in finding enough "translators": people who combine a broad understanding of IT and data analytics with domain-specific expertise in the organization's individual products and markets. Such people are essential to the success of real-world projects, and they should be cultivated assiduously.
- Processes. Big data and advanced analytics projects require an agile development approach, and a readiness to test, fail, and restart until a workable solution is found. Especially for hardware companies used to a slower, more risk-averse way of working, this may require a significant change in established development processes. A willingness to embrace small-scale pilots and beta-tests with "minimum viable products" is a must.
4. Operating model
Finally, companies need the right operating model. That operating model encompasses the organizational structures and capabilities they provide to support their big data, advanced analytics, and IoT efforts, along with the processes and governance needed for support the changes are scaled up and rolled out across the organization.
Many companies find it advantageous to establish a dedicated center of competence (CoC) for analytics. Initially, the CoC can serve as a pool of expertise, supporting the identification, testing, and development of specific use cases. Subsequently, it can spearhead the creation of standard processes and blueprints for data quality, data lifecycle management, and analytics technologies. Finally, it can become a catalyst for a wider organizational transformation, supporting the large-scale capability-building and change-management work required to build new approaches and techniques into the DNA of the R&D function, and the business as a whole.
* * *
Digital technologies, including the IoT, big data, and advanced analytics, are set to revolutionize both the products and services companies develop and the way that development is done. To capture the potential before competitors do, companies need a compelling and ambitious vision, a pragmatic approach that focuses on business value, and a willingness to invest in new capabilities, processes, and tools.
About the authors: Elia Berteletti is an associate partner in McKinsey’s Taipei office, Eric Hannon is a partner in the Frankfurt office, and Thomas Morel is an associate partner in the Lyon office. The authors wish to thank Sander Smits and Florian Weig for their contributions to this article.
1 1021 bytes, or about 8 million years of ultra-high-definition video data.